Regressive Event-Tracker: A Causal Prediction Modelling of Degradation in High Speed Manufacturing
Procedia Manufacturing(2020)
摘要
Abstract The proposed work describes a dynamic regression based event-tracker for high speed production process. The methodology discussed is a causal system and provides trends and estimations of the sensors based on a flexible regression model of the historical sensor values. A safety threshold is defined that provides a boundary of the tolerant working for the regime condition of production. This threshold is used as a reference to calculate the remaining useful life of the critical component. The estimated remaining useful life is compared with the Weibull reliability analysis. The proposed methodology provides a remaining useful life of ∼ 10 weeks for the thermal regulator use-case when compared to ∼ 9 weeks for Weibull analysis. The overestimation of the methodology is discussed and along with the alternative methodology. The sensitivity analysis is conducted on the noise and training periods are studied for better prediction.
更多查看译文
关键词
predictive maintenance, machine learning, regression, failure-rate, event based prediction, Industrial IoT, Industry 4.0, degradation model, remaining useful life
AI 理解论文
溯源树
样例
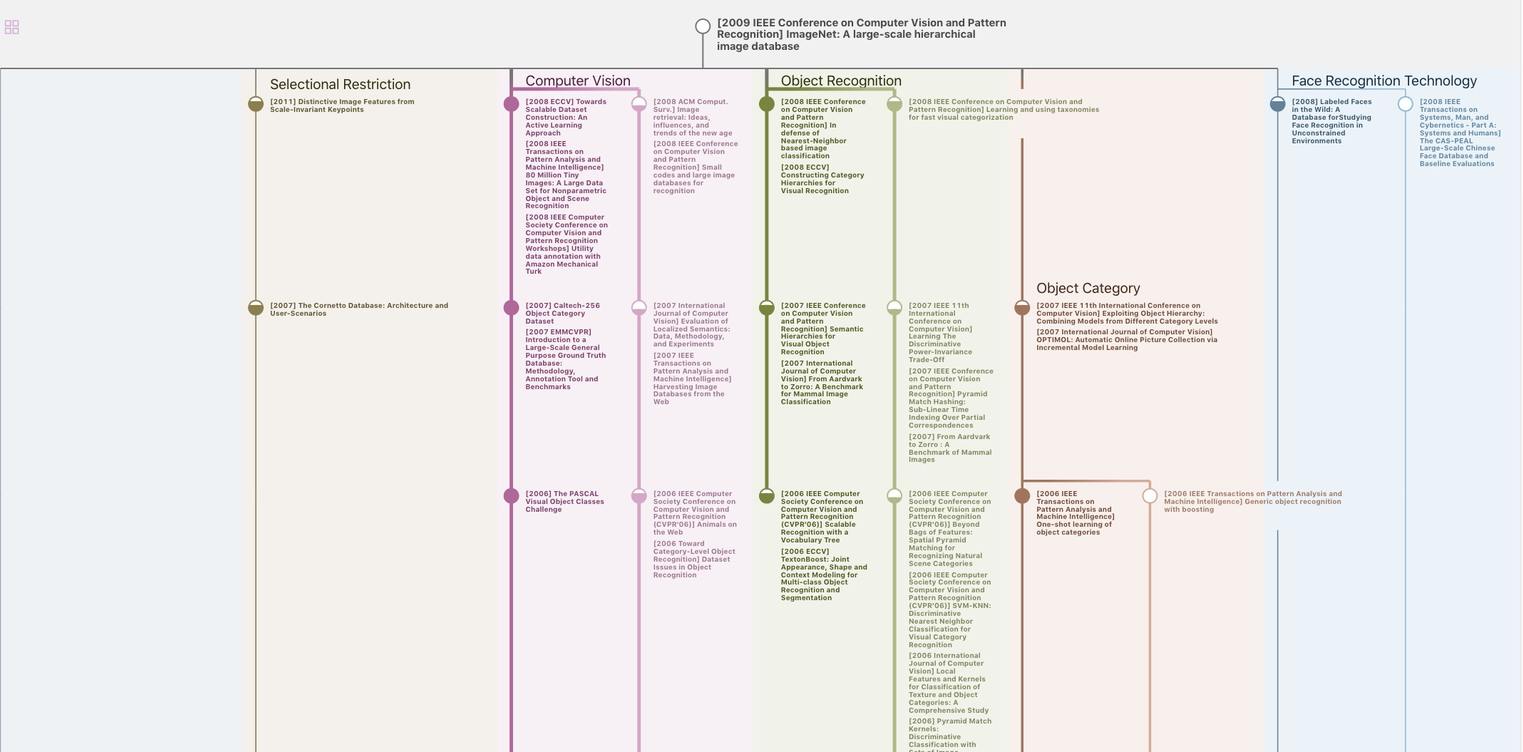
生成溯源树,研究论文发展脉络
Chat Paper
正在生成论文摘要