Optimization and prediction of volume shrinkage and warpage of injection-molded thin-walled parts based on neural network
The International Journal of Advanced Manufacturing Technology(2020)
摘要
Warpage and volume shrinkage are important indicators of the quality of thin-walled parts during injection molding. In this study, the optimization goals are warpage and volume shrinkage. Design parameters include mold temperature, melt temperature, injection time, holding time, cooling time, and holding pressure. Based on the orthogonal experimental design and response surface experimental design, the MoldFlow software is applied to the simulation of the thin-walled part injection molding process. The importance of various parameters on warpage and volume shrinkage was analyzed by using analysis of variance. Based on the simulation results, a two-layer hidden-layer back propagation (BP) neural network model is established and the genetic algorithm (GA) is used to optimize the weights and thresholds of the back propagation neural network (BPNN) model to reduce warpage and volume shrinkage by optimizing the design parameters significantly. A support vector machine (SVM) combined with GA-BP was used to build a prediction model for predicting warpage and volume shrinkage. Taking the automobile wire harness protection frame as an example, and verified by numerical simulation, the GA-optimized two-layer hidden-layer BP neural network combination method is an effective method for injection molding to reduce warpage and volume shrinkage of thin-walled parts. SVM-BP-GA can accurately provide predictions for optimization goals; the amount of warpage and the volume shrinkage were 0.93% and 1.9%, respectively.
更多查看译文
关键词
Injection molding simulation,BP neural network,Genetic algorithm,Support vector machines,Multi-objective optimization,Response surface method
AI 理解论文
溯源树
样例
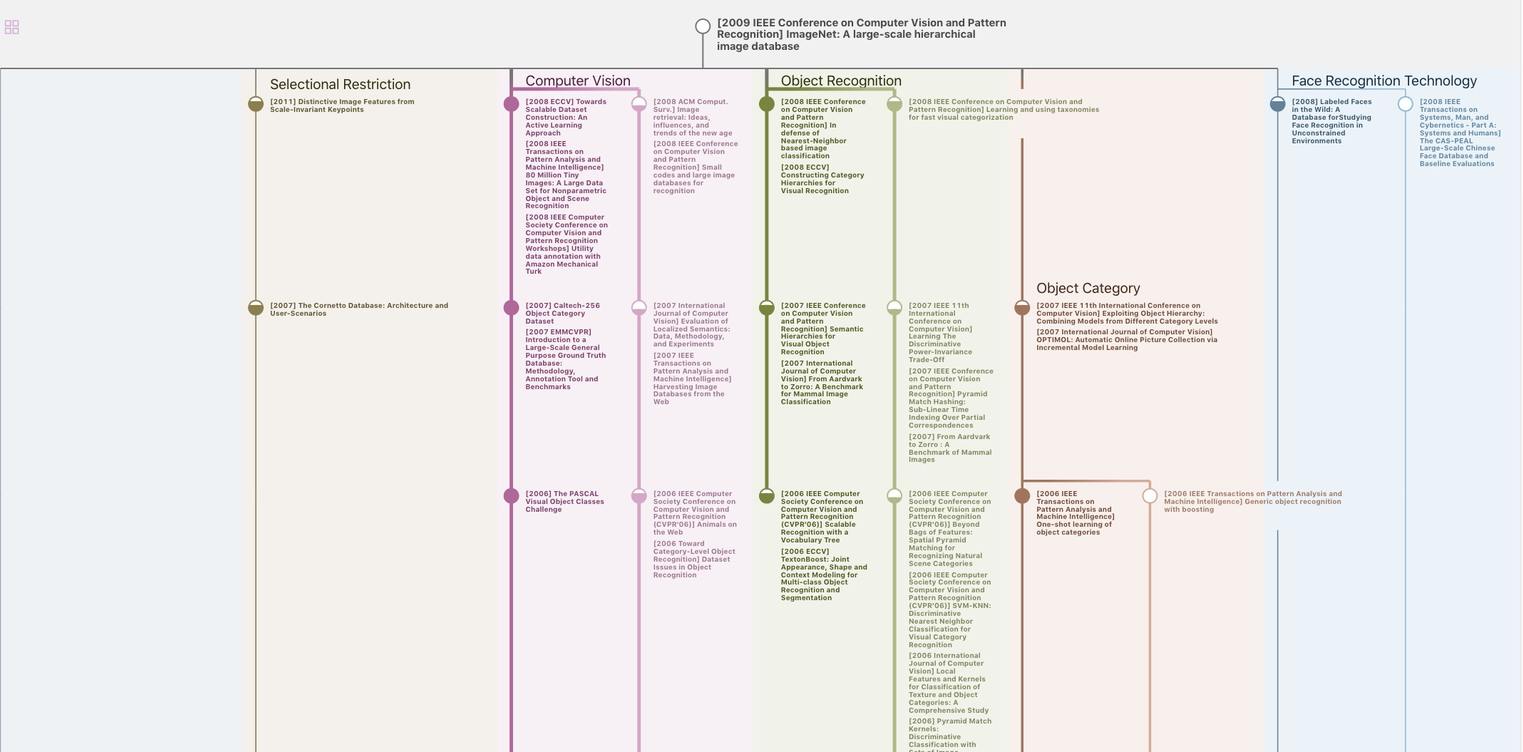
生成溯源树,研究论文发展脉络
Chat Paper
正在生成论文摘要