Deep learning-based method coupled with small sample learning for solving partial differential equations
MULTIMEDIA TOOLS AND APPLICATIONS(2020)
摘要
Partial differential equations (PDEs) are existing widely in the field of mathematics, physics and engineering. They are often used to describe natural phenomena and model dynamical systems, but how to solve the equations efficiently is still a hard task. In this paper, we develop a deep learning-based general numerical method coupled with small sample learning (SSL) for solving PDEs. To be more specific, we approximate the solution via a deep feedforward neural network, which is trained to satisfy the PDEs with the initial and boundary conditions. Then the proposed method is modeled to solve an optimization problem by minimizing a designed cost function, which involves the residual of the differential equations, the initial/boundary conditions and the residual of a handful of observations. With a few of sample data, the model can be rectified effectively and the predictive accuracy can be improved. The effectiveness of the proposed method is demonstrated by a wide range of benchmark problems in mathematical physics, including the classical Burgers equations, Schrödinger equations, Buckley-Leverett equation, Navier-Stokes equation, and Carburizing diffusion equations, which are applied in carburizing diffusion problems in material science. And the results validate that the proposed algorithm is effective, flexible and robust without relying on trial solutions.
更多查看译文
关键词
Partial differential equations,Neural network,Numerical solution,Small sample learning
AI 理解论文
溯源树
样例
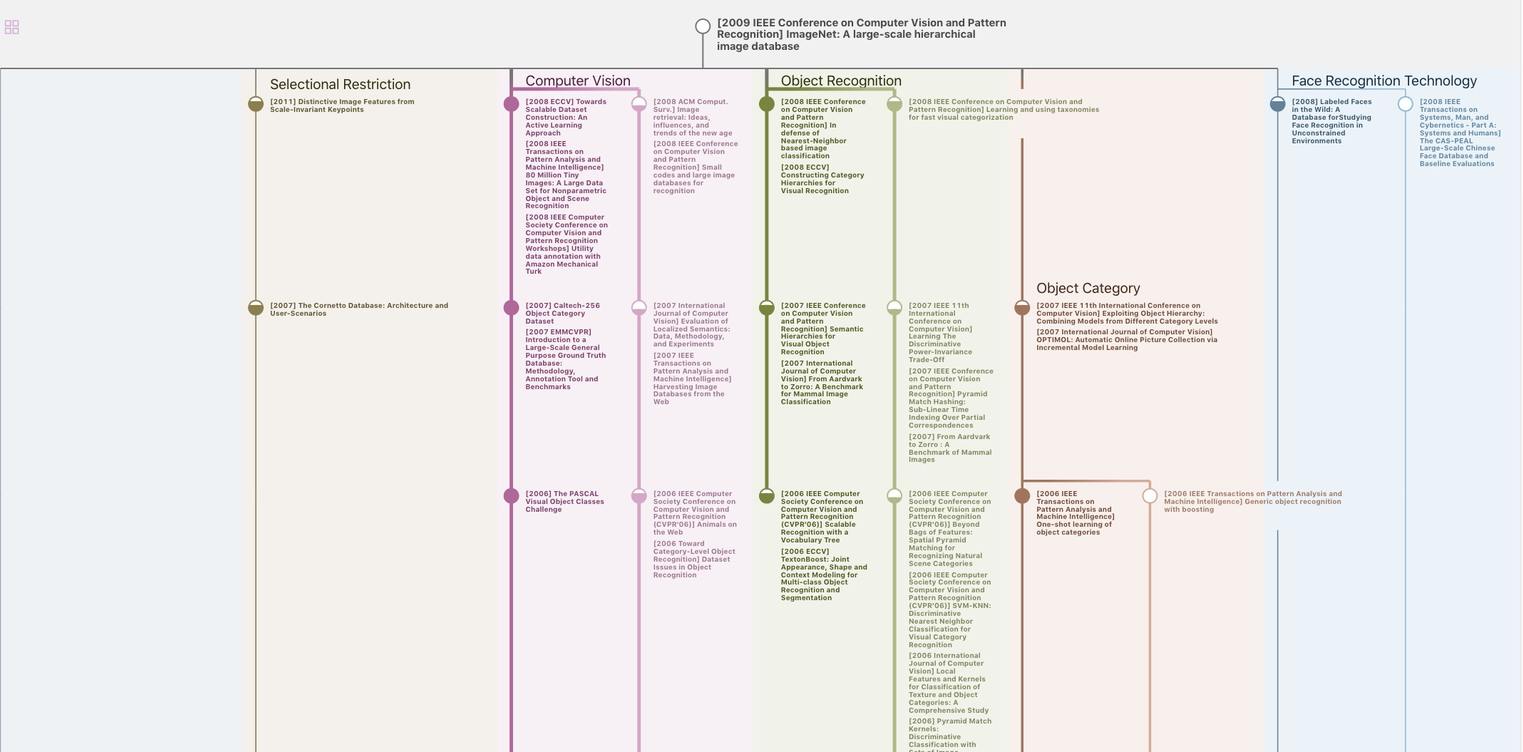
生成溯源树,研究论文发展脉络
Chat Paper
正在生成论文摘要