Intelligent Fault Diagnosis by Fusing Domain Adversarial Training and Maximum Mean Discrepancy via Ensemble Learning
IEEE Transactions on Industrial Informatics(2021)
摘要
Nowadays, the industrial Internet of Things (IIoT) has been successfully utilized in smart manufacturing. The massive amount of data in IIoT promote the development of deep learning-based health monitoring for industrial equipment. Since monitoring data for mechanical fault diagnosis collected on different working conditions or equipment have domain mismatch, models trained with training data may not work in practical applications. Therefore, it is essential to study fault diagnosis methods with domain adaptation ability. In this article, we propose an intelligent fault diagnosis method based on an improved domain adaptation method. Specifically, two feature extractors concerning feature space distance and domain mismatch are trained using maximum mean discrepancy and domain adversarial training respectively to enhance feature representation. Since separate classifiers are trained for feature extractors, ensemble learning is further utilized to obtain final results. Experimental results indicate that the proposed method is effective and applicable in diagnosing faults with domain mismatch.
更多查看译文
关键词
Domain adaptation,domain adversarial training (DAT),ensemble learning,fault diagnosis,maximum mean discrepancy (MMD)
AI 理解论文
溯源树
样例
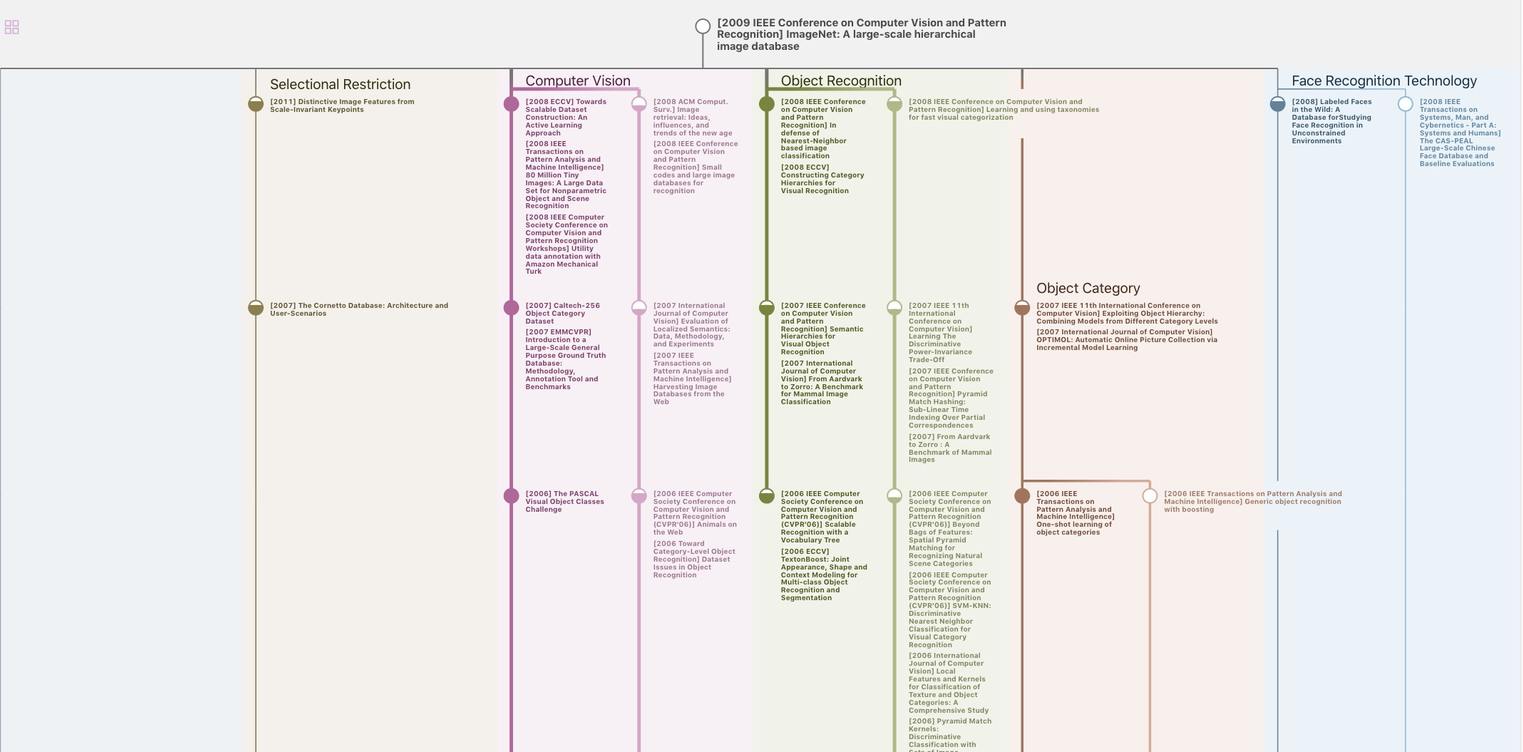
生成溯源树,研究论文发展脉络
Chat Paper
正在生成论文摘要