Quantitative Evaluation of Stroke Patients' Wrist Paralysis by Estimation of Kinematic Coefficients and Machine Learning
SENSORS AND MATERIALS(2020)
摘要
The increasing population of stroke survivors naturally produces needs for more effective rehabilitation systems for both patients and therapists. Robotic therapies are widely studied and practiced in various fields since they enable intense exercise as well as numerical evaluations. In this paper, along with the rehabilitation robot we developed, we propose a quantitative evaluation method for wrist paralysis in stroke patients using kinematic coefficients estimated from the joint model and machine learning. Through experiments on five hemiplegic patients, we observed the spring-damper characteristics of their paralyzed wrists and computed the coefficients that represent stiffness and viscosity. During wrist extension, a patient at Brunnstrom stage 3 showed a high average stiffness of 4.453 Nm/rad and viscosity of 4.533 Nms/rad toward the rest position, whereas a patient at Brunnstrom stage 4 showed smaller coefficients of 1.135 Nm/rad and -0.669 Nms/rad, respectively. We applied a support vector machine and a k-means method to the estimated stiffnesses and viscosities to classify the patients into three different clusters. The two coefficients not only helped discriminate patients in accordance with their Brunnstrom stage, but also revealed that patients at the same stage could be more finely categorized.
更多查看译文
关键词
stroke,hemiplegia,rehabilitation,robotic therapy,machine learning
AI 理解论文
溯源树
样例
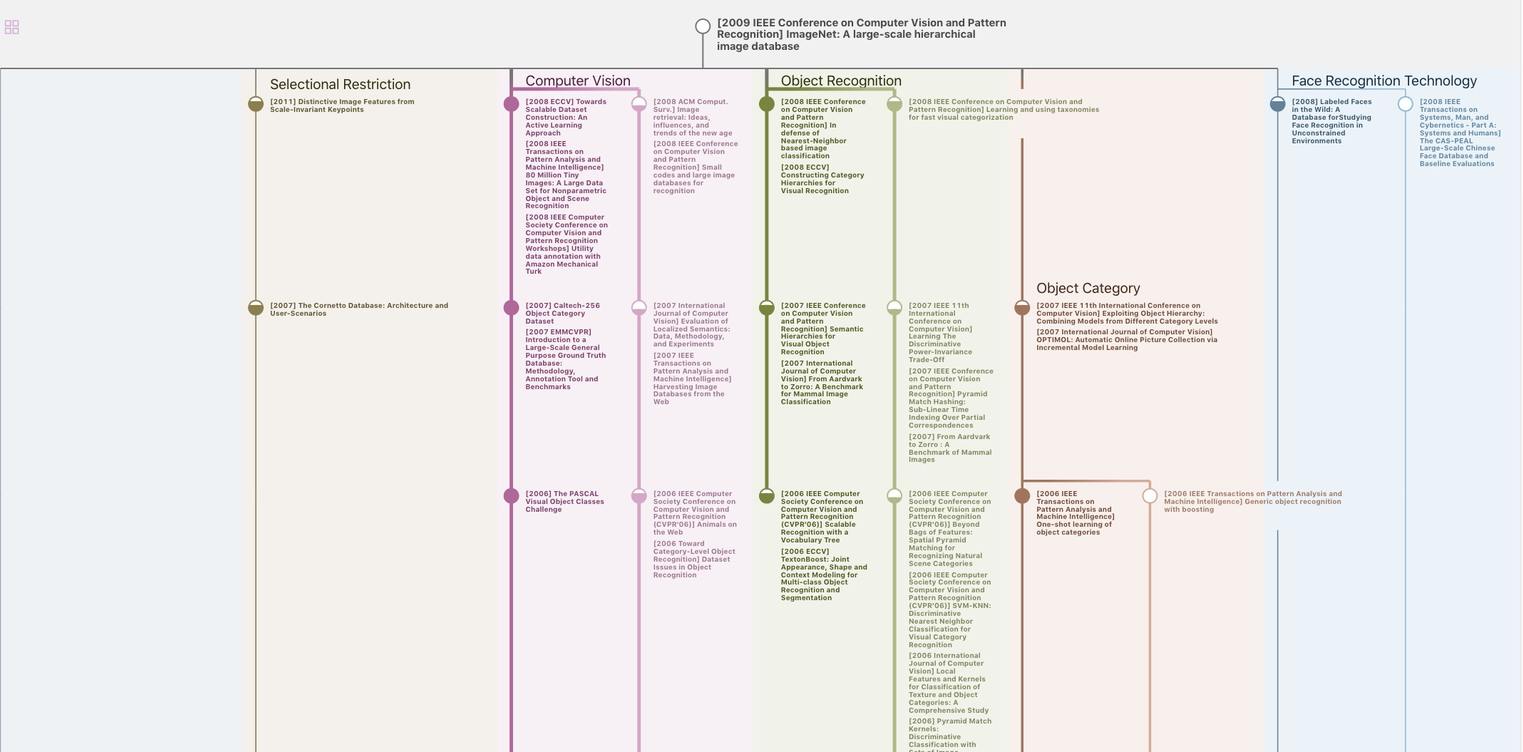
生成溯源树,研究论文发展脉络
Chat Paper
正在生成论文摘要