Learning the Predictability of the Future
2021 IEEE/CVF CONFERENCE ON COMPUTER VISION AND PATTERN RECOGNITION, CVPR 2021(2021)
摘要
We introduce a framework for learning from unlabeled video what is predictable in the future. Instead of committing up front to features to predict, our approach learns from data which features are predictable. Based on the observation that hyperbolic geometry naturally and compactly encodes hierarchical structure, we propose a predictive model in hyperbolic space. When the model is most confident, it will predict at a concrete level of the hierarchy, but when the model is not confident, it learns to automatically select a higher level of abstraction. Experiments on two established datasets show the key role of hierarchical representations for action prediction. Although our representation is trained with unlabeled video, visualizations show that action hierarchies emerge in the representation.
更多查看译文
关键词
predictability,future,learning
AI 理解论文
溯源树
样例
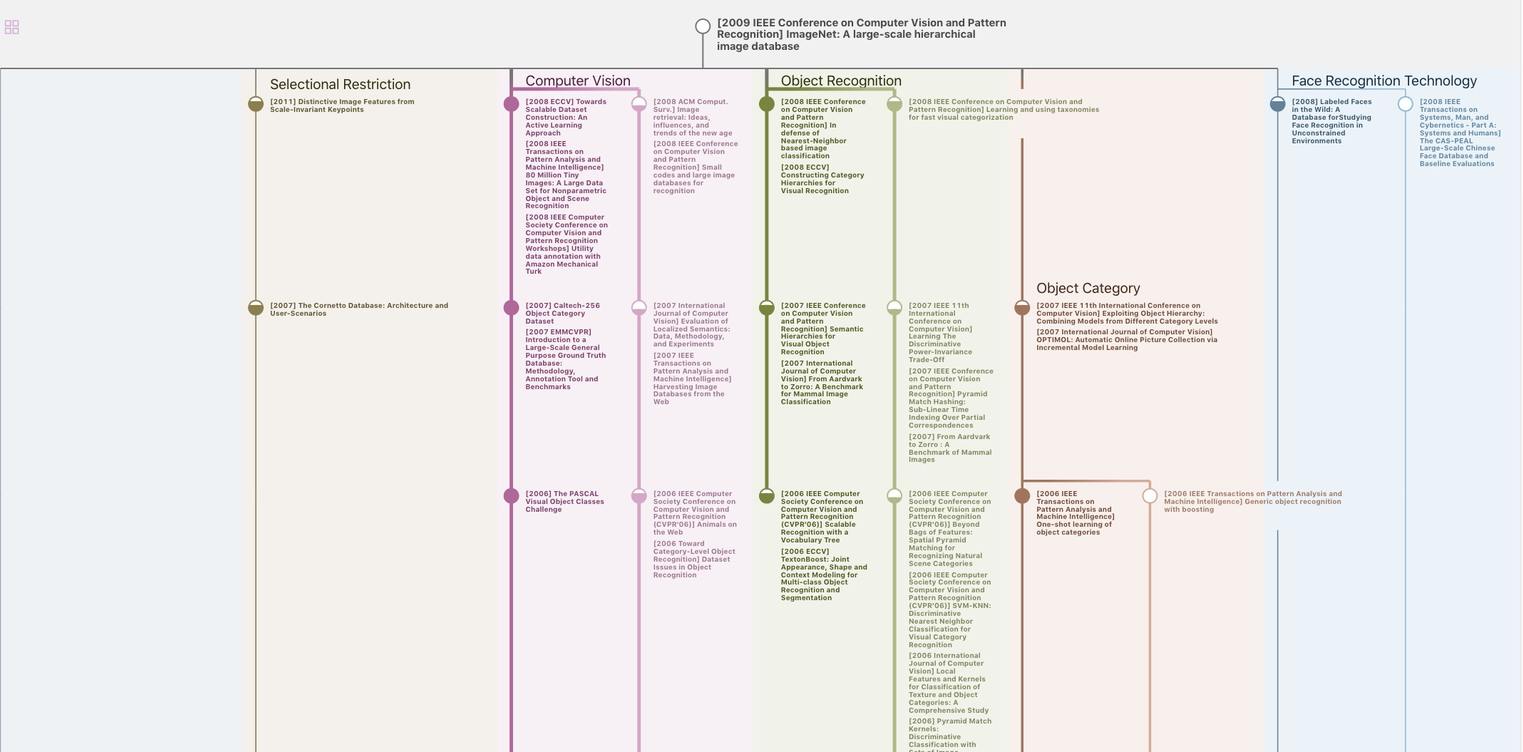
生成溯源树,研究论文发展脉络
Chat Paper
正在生成论文摘要