Exploring Brain Dynamic Functional Connectivity Using Improved Principal Components Analysis Based on Template Matching
BRAIN TOPOGRAPHY(2021)
摘要
Principle components analysis (PCA) can be used to detect repeating co-variant patterns of resting-state dynamic functional connectivity (DFC) of brain networks, accompanied with sliding-window technique. However, the robustness of PCA-based DFC-state extraction (DFC-PCA) is poorly studied. We investigated the reliability of PCA results and improved the robustness of DFC-PCA for a limited sample size. We first established how PCA-based DFC results varied with sample size and PC order in five rounds of bootstrapping with different sample sizes. The consistency across trials increased with increasing sample size and/or decreasing PC order. We then developed a framework based on PC matching and reordering to obtain a more reliable estimation of co-variant DFC patterns. With either the identical template generated by the surrogate dataset itself or with the external template obtained from existing results, the perceptual hash algorithm was used to reorder PCs according to their patterns. After order correction, reliable results were obtained by averaging across trials within each surrogate dataset. This newly developed framework allowed simultaneous measurement and improvement of DFC-PCA. This consistency could also be used as a criterion for PC selection and interpretation to support the reliability and validity of the conclusion.
更多查看译文
关键词
Brain network, Dynamic functional connectivity, Principal components analysis, Resting-state, Template matching
AI 理解论文
溯源树
样例
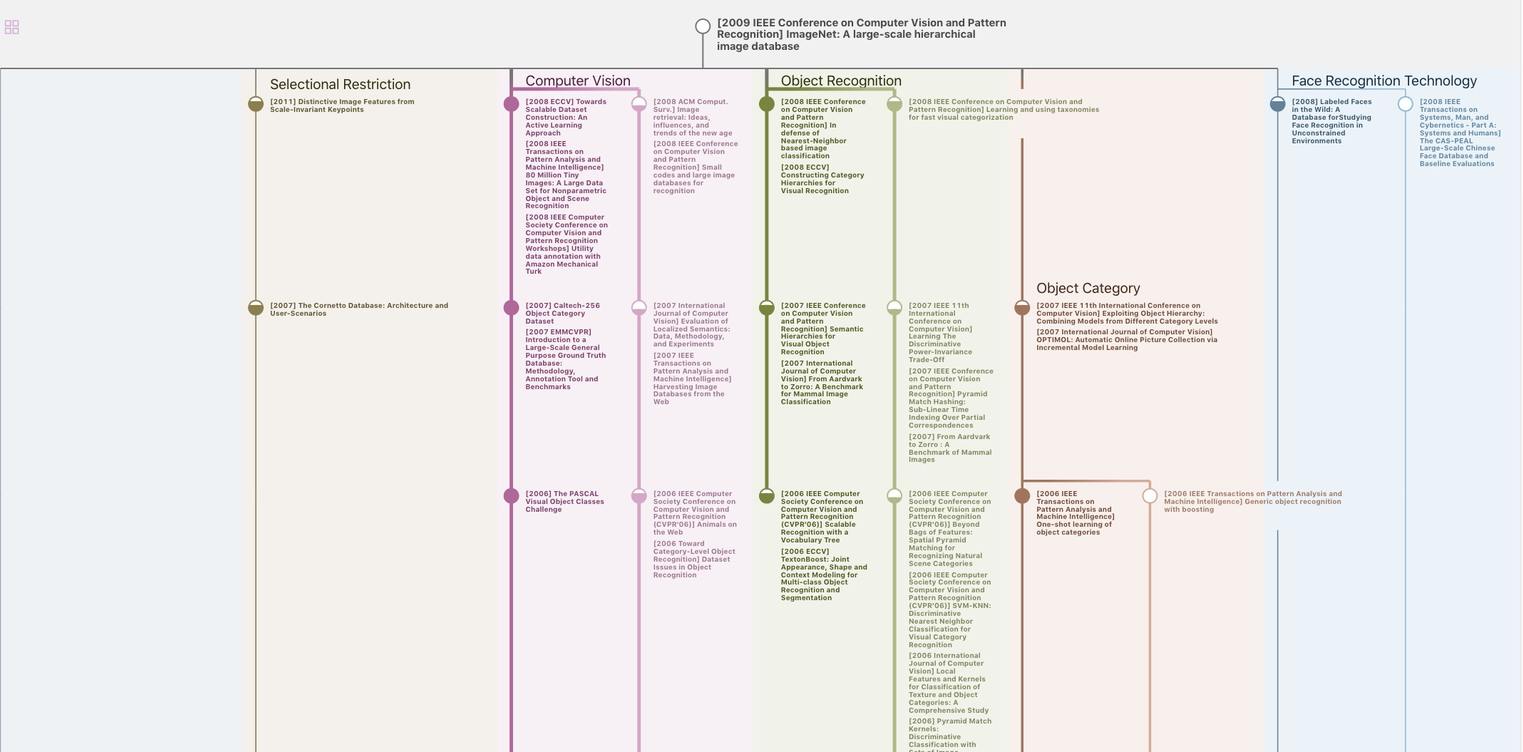
生成溯源树,研究论文发展脉络
Chat Paper
正在生成论文摘要