Knowledge Transfer Between Brain Lesion Segmentation Tasks With Increased Model Capacity
COMPUTERIZED MEDICAL IMAGING AND GRAPHICS(2021)
摘要
Convolutional neural networks (CNNs) have become an increasingly popular tool for brain lesion segmentation in recent years due to its accuracy and efficiency. However, CNN-based brain lesion segmentation generally requires a large amount of annotated training data, which can be costly for medical imaging. In many scenarios, only a few annotations of brain lesions are available. One common strategy to address the issue of limited annotated data is to transfer knowledge from a different yet relevant source task, where training data is abundant, to the target task of interest. Typically, a model can be pretrained for the source task, and then fine-tuned with the scarce training data associated with the target task. However, classic fine-tuning tends to make small modifications to the pretrained model, which could hinder its adaptation to the target task. Fine-tuning with increased model capacity has been shown to alleviate this negative impact in image classification problems. In this work, we extend the strategy of fine-tuning with increased model capacity to the problem of brain lesion segmentation, and then develop an advanced version that is better suitable for segmentation problems. First, we propose a vanilla strategy of increasing the capacity, where, like in the classification problem, the width of the network is augmented during fine-tuning. Second, because unlike image classification, in segmentation problems each voxel is associated with a labeling result, we further develop a spatially adaptive augmentation strategy during fine-tuning. Specifically, in addition to the vanilla width augmentation, we incorporate a module that computes a spatial map of the contribution of the information given by width augmentation in the final segmentation. For demonstration, the proposed method was applied to ischemic stroke lesion segmentation, where a model pretrained for brain tumor segmentation was fine-tuned, and the experimental results indicate the benefit of our method.
更多查看译文
关键词
Brain lesion segmentation, Knowledge transfer, Fine-tuning, Increased model capacity
AI 理解论文
溯源树
样例
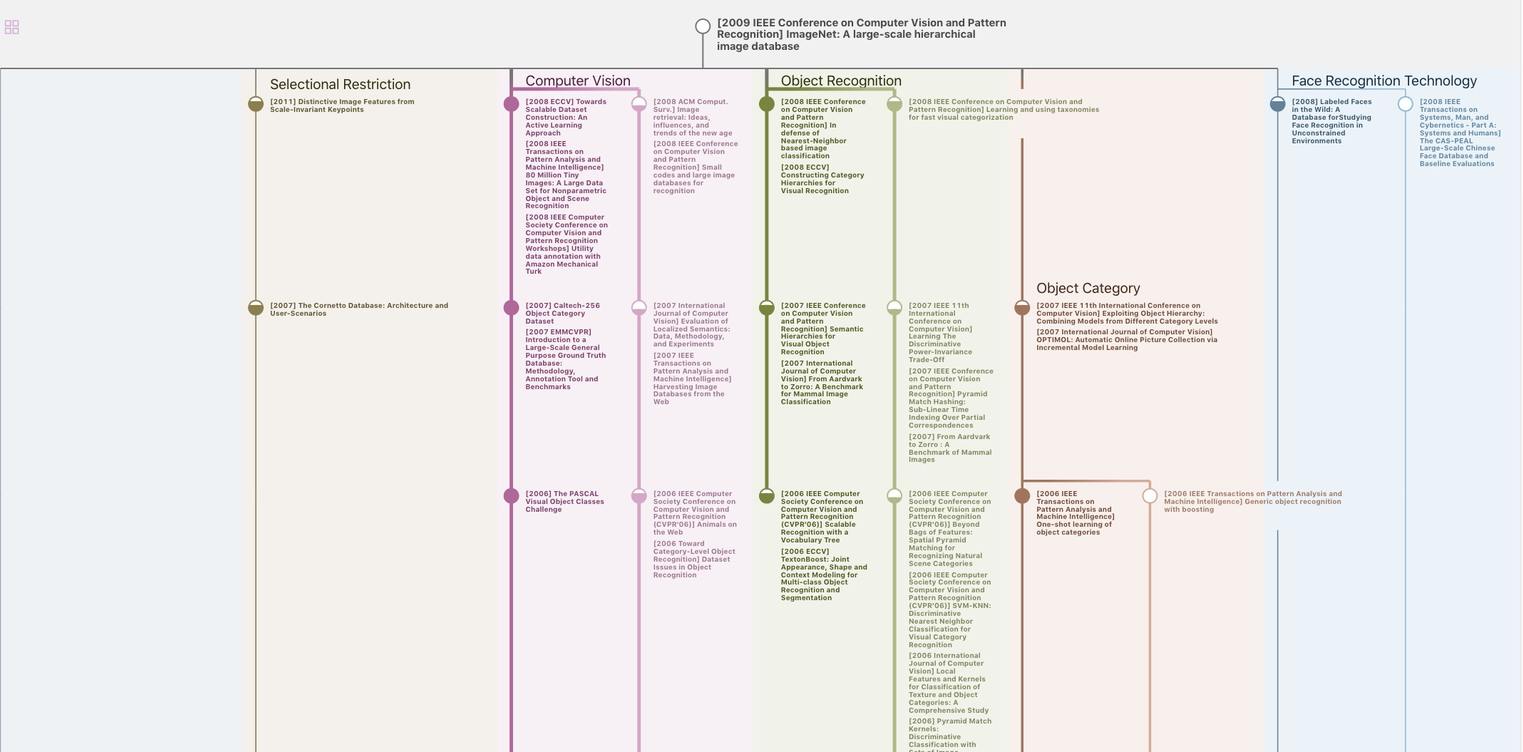
生成溯源树,研究论文发展脉络
Chat Paper
正在生成论文摘要