On the Performance of SQP Methods for Nonlinear Optimization
Springer Proceedings in Mathematics & Statistics(2015)
摘要
This paper concerns some practical issues associated with the formulation of sequential quadratic programming (SQP) methods for large-scale nonlinear optimization. SQP methods find approximate solutions of a sequence of quadratic programming (QP) subproblems in which a quadratic model of the Lagrangian is minimized subject to the linearized constraints. Numerical results are given for 1153 problems from the CUTEst test collection. The results indicate that SQP methods based on maintaining a quasi-Newton approximation to the Hessian of the Lagrangian function are both reliable and efficient for general large-scale optimization problems. In particular, the results show that in some situations, quasi-Newton SQP methods are more efficient than interior methods that utilize the exact Hessian of the Lagrangian. The paper concludes with discussion of an SQP method that employs both approximate and exact Hessian information. In this approach the quadratic programming subproblem is either the conventional subproblem defined in terms of a positive-definite quasi-Newton approximate Hessian or a convexified subproblem based on the exact Hessian.
更多查看译文
关键词
Nonlinear programming,Sequential quadratic programming,SQP methods,Interior methods,Convexification,Active-set methods,Quadratic programming
AI 理解论文
溯源树
样例
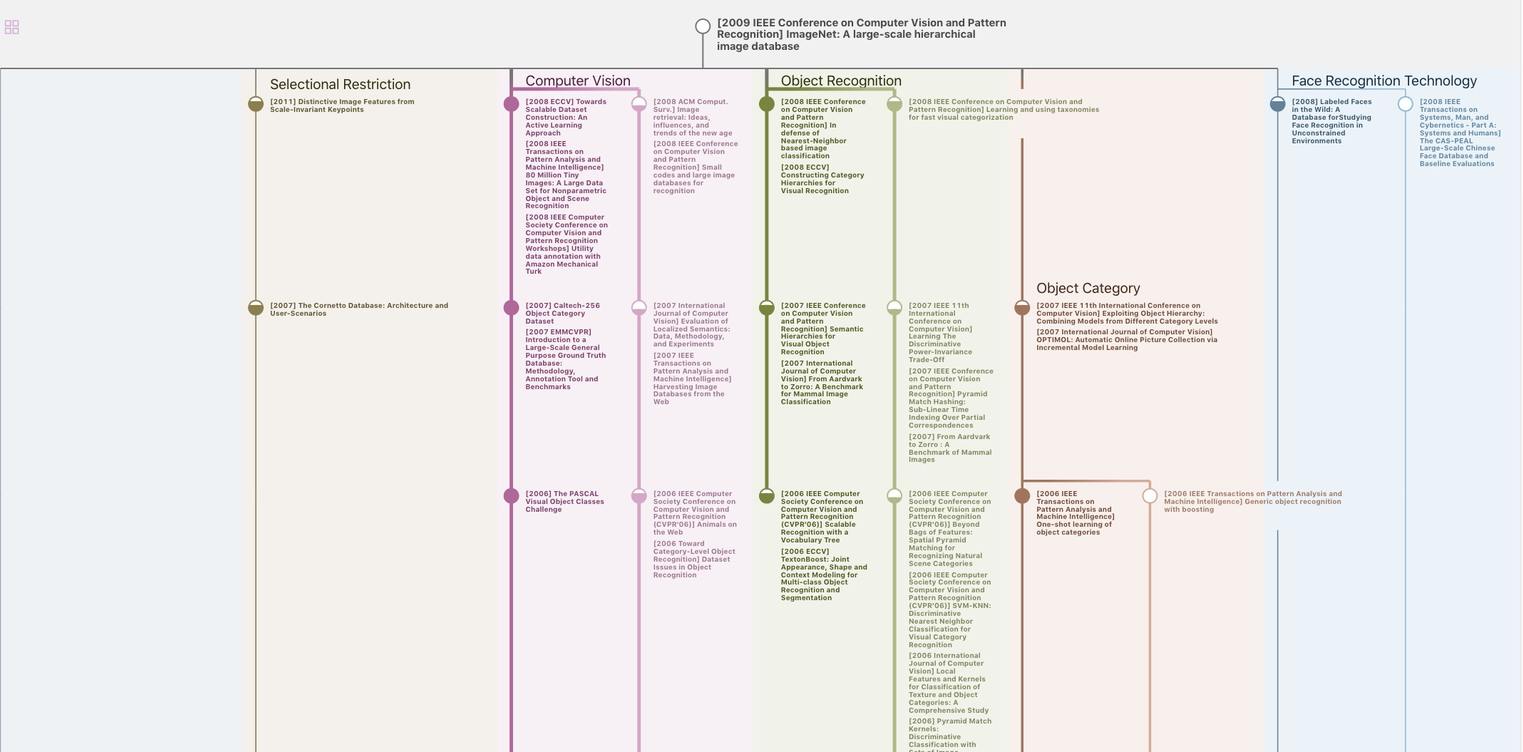
生成溯源树,研究论文发展脉络
Chat Paper
正在生成论文摘要