Robust-Optimal Design Using Multifidelity Models
Conference Proceedings of the Society for Experimental Mechanics Series(2015)
摘要
Applications in engineering analysis and design have benefited from the use of numerical models to supplement or replace the costly design-build-test paradigm. Previous work has acknowledged that design optimization should not only consider the performance of the model, but also be as insensitive as possible, or robust, to sources of uncertainty that are used to define the simulation. Clearly, evaluating robustness to sources of uncertainty can be computationally expensive, due to the number of iterations required at every step of the optimization. Multifidelity techniques have been introduced to mitigate this computational expense by taking advantage of fast-running lower-fidelity models or emulators. Herein, to achieve robust design, we argue that it is more effective to reduce the total range of variation in model performance rather than to reduce the standard deviation of model performances due to uncertainty in calibration variables of the model. We utilize a multifidelity approach to apply this paradigm to a sub-problem of the NASA Uncertainty Quantification Challenge problem, which is a high-dimensional and nonlinear MATLAB-based code used to simulate dynamics of remotely operated aircraft developed at NASA Langley. This method demonstrates an alternative and computationally efficient approach to robust design.
更多查看译文
关键词
Robust design,Multi-fidelity optimization,Metamodels,Uncertainty,Info-gap
AI 理解论文
溯源树
样例
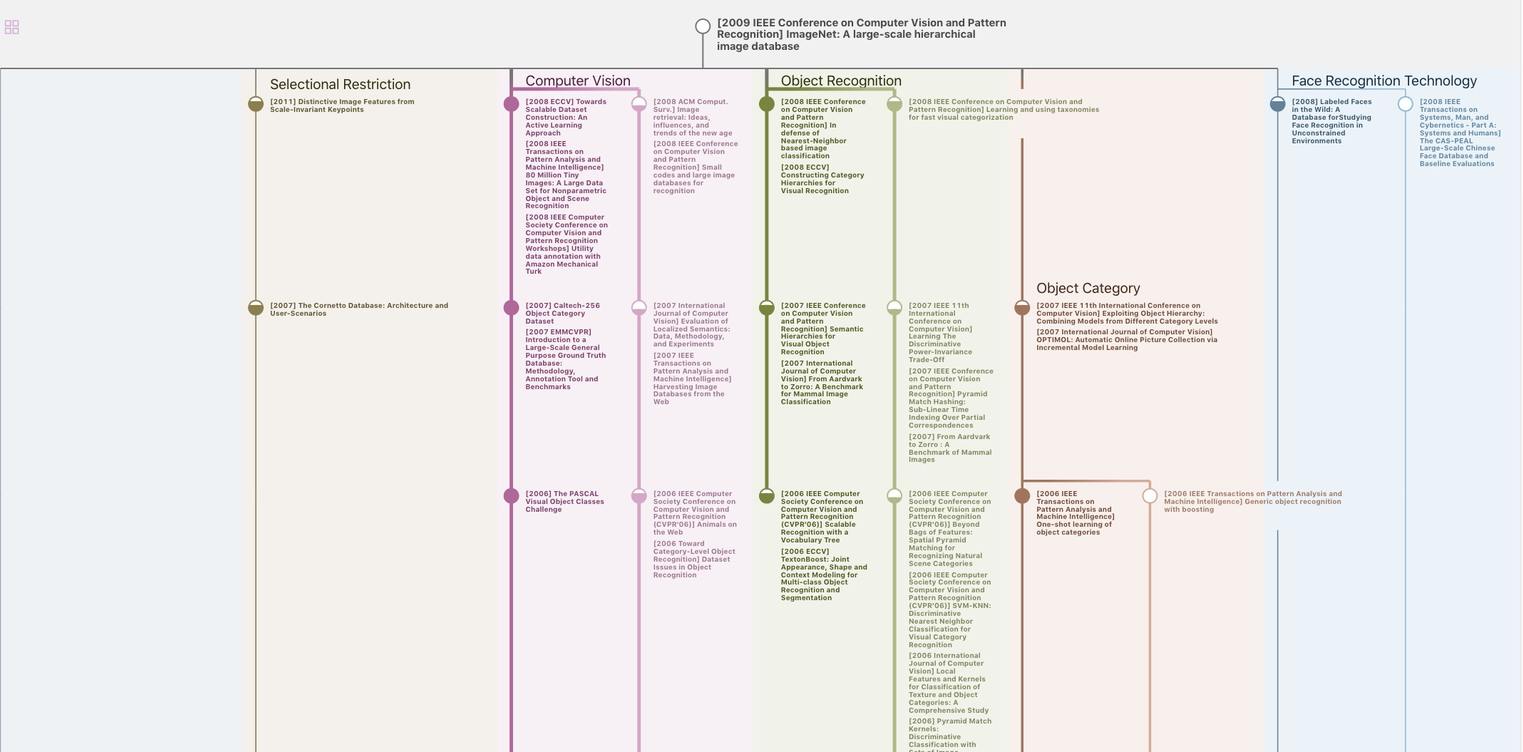
生成溯源树,研究论文发展脉络
Chat Paper
正在生成论文摘要