A Hybrid Deep Learning Approach to Predict Malignancy of Breast Lesions Using Mammograms
Proceedings of SPIE(2018)
摘要
Applying deep learning technology to medical imaging informatics field has been recently attracting extensive research interest. However, the limited medical image dataset size often reduces performance and robustness of the deep learning based computer-aided detection and/or diagnosis (CAD) schemes. In attempt to address this technical challenge, this study aims to develop and evaluate a new hybrid deep learning based CAD approach to predict likelihood of a breast lesion detected on mammogram being malignant. In this approach, a deep Convolutional Neural Network (CNN) was firstly pre-trained using the ImageNet dataset and serve as a feature extractor. A pseudo-color Region of Interest (ROI) method was used to generate ROIs with RGB channels from the mammographic images as the input to the pre-trained deep network. The transferred CNN features from different layers of the CNN were then obtained and a linear support vector machine (SVM) was trained for the prediction task. By applying to a dataset involving 301 suspicious breast lesions and using a leave-one-case-out validation method, the areas under the ROC curves (AUC) = 0.762 and 0.792 using the traditional CAD scheme and the proposed deep learning based CAD scheme, respectively. An ensemble classifier that combines the classification scores generated by the two schemes yielded an improved AUC value of 0.813. The study results demonstrated feasibility and potentially improved performance of applying a new hybrid deep learning approach to develop CAD scheme using a relatively small dataset of medical images.
更多查看译文
关键词
Computer-aided diagnosis (CAD),Deep learning,Convolutional neural network (CNN),Deep transfer learning,Mammographic mass classification
AI 理解论文
溯源树
样例
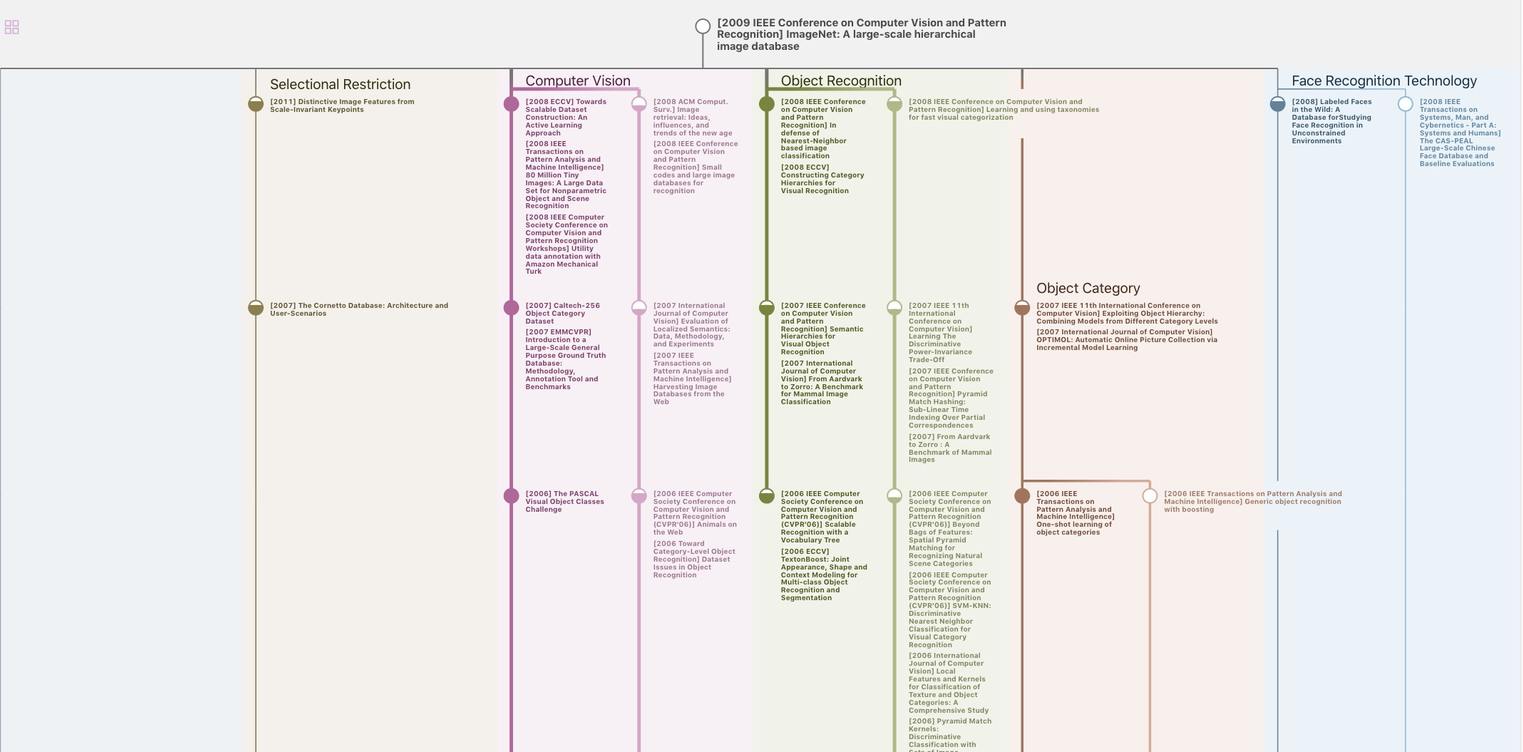
生成溯源树,研究论文发展脉络
Chat Paper
正在生成论文摘要