High Resolution OCT Image Generation Using Super Resolution via Sparse Representation
Proceedings of SPIE(2017)
摘要
In this paper we propose a technique for obtaining a high resolution (HR) image from a single low resolution (LR) image - using joint learning dictionary - on the basis of image statistic research. It suggests that with an appropriate choice of an over-complete dictionary, image patches can be well represented as a sparse linear combination. Medical imaging for clinical analysis and medical intervention is being used for creating visual representations of the interior of a body, as well as visual representation of the function of some organs or tissues (physiology). A number of medical imaging techniques are in use like MRI, CT scan, X-rays and Optical Coherence Tomography (OCT). OCT is one of the new technologies in medical imaging and one of its uses is in ophthalmology where it is being used for analysis of the choroidal thickness in the eyes in healthy and disease states such as age-related macular degeneration, central serous chorioretinopathy, diabetic retinopathy and inherited retinal dystrophies. We have proposed a technique for enhancing the OCT images which can be used for clearly identifying and analyzing the particular diseases. Our method uses dictionary learning technique for generating a high resolution image from a single input LR image. We train two joint dictionaries, one with OCT images and the second with multiple different natural images, and compare the results with previous SR technique. Proposed method for both dictionaries produces HR images which are comparatively superior in quality with the other proposed method of SR. Proposed technique is very effective for noisy OCT images and produces up-sampled and enhanced OCT images.
更多查看译文
关键词
High resolution,super resolution,sparse representation,optical coherent tomography
AI 理解论文
溯源树
样例
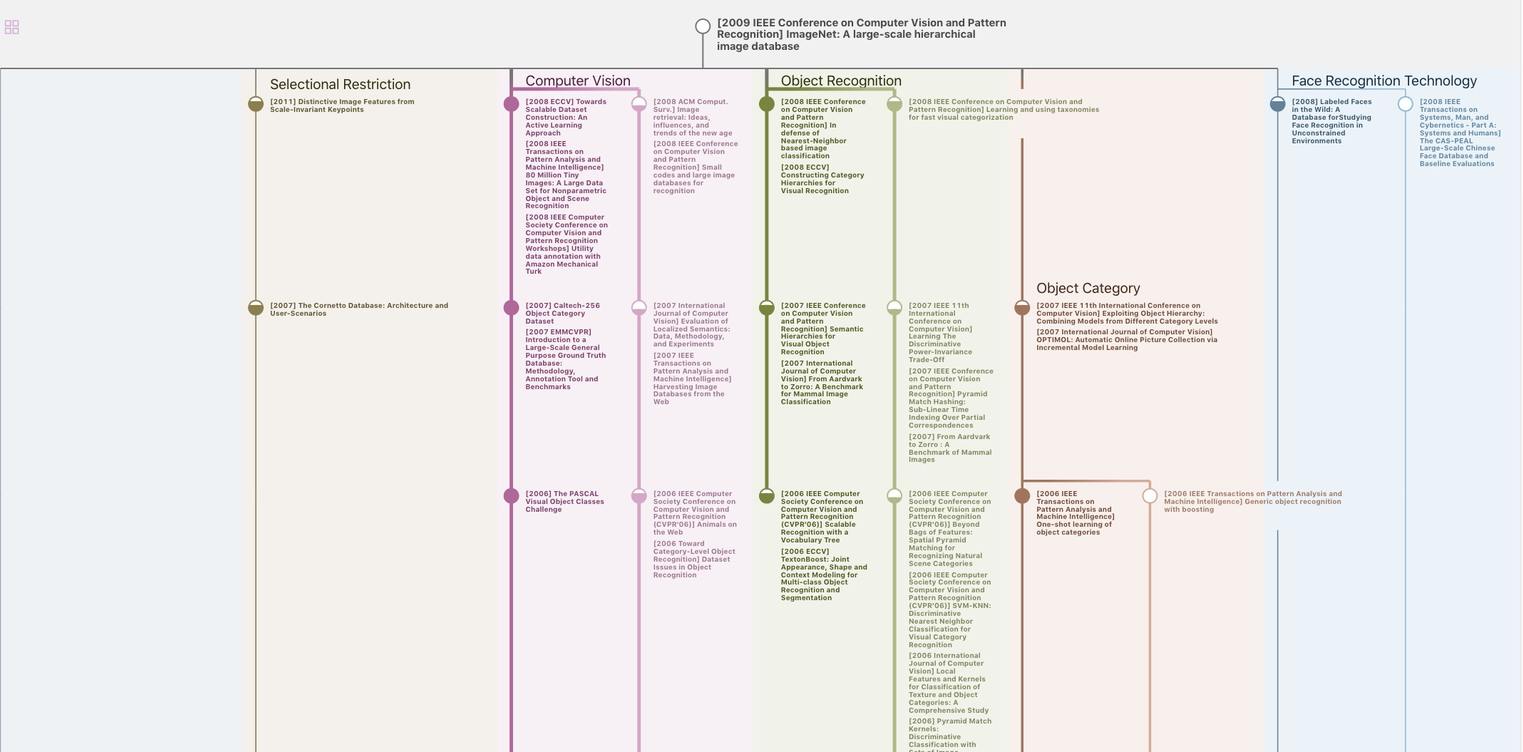
生成溯源树,研究论文发展脉络
Chat Paper
正在生成论文摘要