ALVIO: Adaptive Line and Point Feature-based Visual Inertial Odometry for Robust Localization in Indoor Environments
arxiv(2020)
摘要
The amount of texture can be rich or deficient depending on the objects and the structures of the building. The conventional mono visual-initial navigation system (VINS)-based localization techniques perform well in environments where stable features are guaranteed. However, their performance is not assured in a changing indoor environment. As a solution to this, we propose Adaptive Line and point feature-based Visual Inertial Odometry (ALVIO) in this paper. ALVIO actively exploits the geometrical information of lines that exist in abundance in an indoor space. By using a strong line tracker and adaptive selection of feature-based tightly coupled optimization, it is possible to perform robust localization in a variable texture environment. The structural characteristics of ALVIO are as follows: First, the proposed optical flow-based line tracker performs robust line feature tracking and management. By using epipolar geometry and trigonometry, accurate 3D lines are recovered. These 3D lines are used to calculate the line re-projection error. Finally, with the sensitivity-analysis-based adaptive feature selection in the optimization process, we can estimate the pose robustly in various indoor environments. We validate the performance of our system on public datasets and compare it against other state-of the-art algorithms (S-MSKCF, VINS-Mono). In the proposed algorithm based on point and line feature selection, translation RMSE increased by 16.06% compared to VINS-Mono, while total optimization time decreased by up to 49.31%. Through this, we proved that it is a useful algorithm as a real-time pose estimation algorithm.
更多查看译文
关键词
Visual Inertial Odometry (VIO), Visual Inertial Navigation System (VINS), 3D line features, Sensitivity analysis, Adaptive feature selection, Indoor localization
AI 理解论文
溯源树
样例
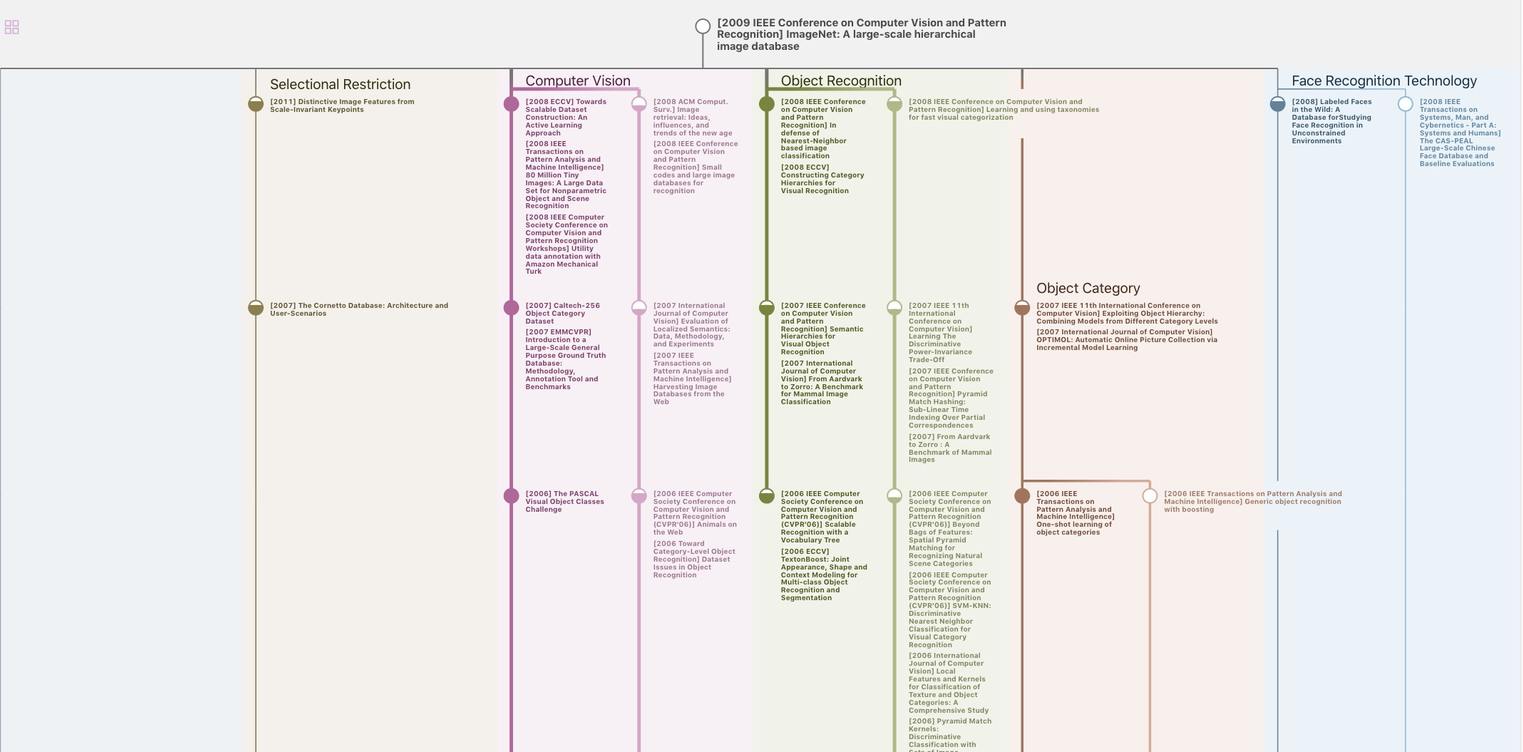
生成溯源树,研究论文发展脉络
Chat Paper
正在生成论文摘要