In-Series U-Net Network To 3d Tumor Image Reconstruction For Liver Hepatocellular Carcinoma Recognition
DIAGNOSTICS(2021)
摘要
Cancer is one of the common diseases. Quantitative biomarkers extracted from standard-of-care computed tomography (CT) scan can create a robust clinical decision tool for the diagnosis of hepatocellular carcinoma (HCC). According to the current clinical methods, the situation usually accounts for high expenditure of time and resources. To improve the current clinical diagnosis and therapeutic procedure, this paper proposes a deep learning-based approach, called Successive Encoder-Decoder (SED), to assist in the automatic interpretation of liver lesion/tumor segmentation through CT images. The SED framework consists of two different encoder-decoder networks connected in series. The first network aims to remove unwanted voxels and organs and to extract liver locations from CT images. The second network uses the results of the first network to further segment the lesions. For practical purpose, the predicted lesions on individual CTs were extracted and reconstructed on 3D images. The experiments conducted on 4300 CT images and LiTS dataset demonstrate that the liver segmentation and the tumor prediction achieved 0.92 and 0.75 in Dice score, respectively, by as-proposed SED method.
更多查看译文
关键词
deep learning, liver lesion segmentation, 3D segmentation display, U-Net, hepatocellular carcinoma, successive Encoder-Decoder
AI 理解论文
溯源树
样例
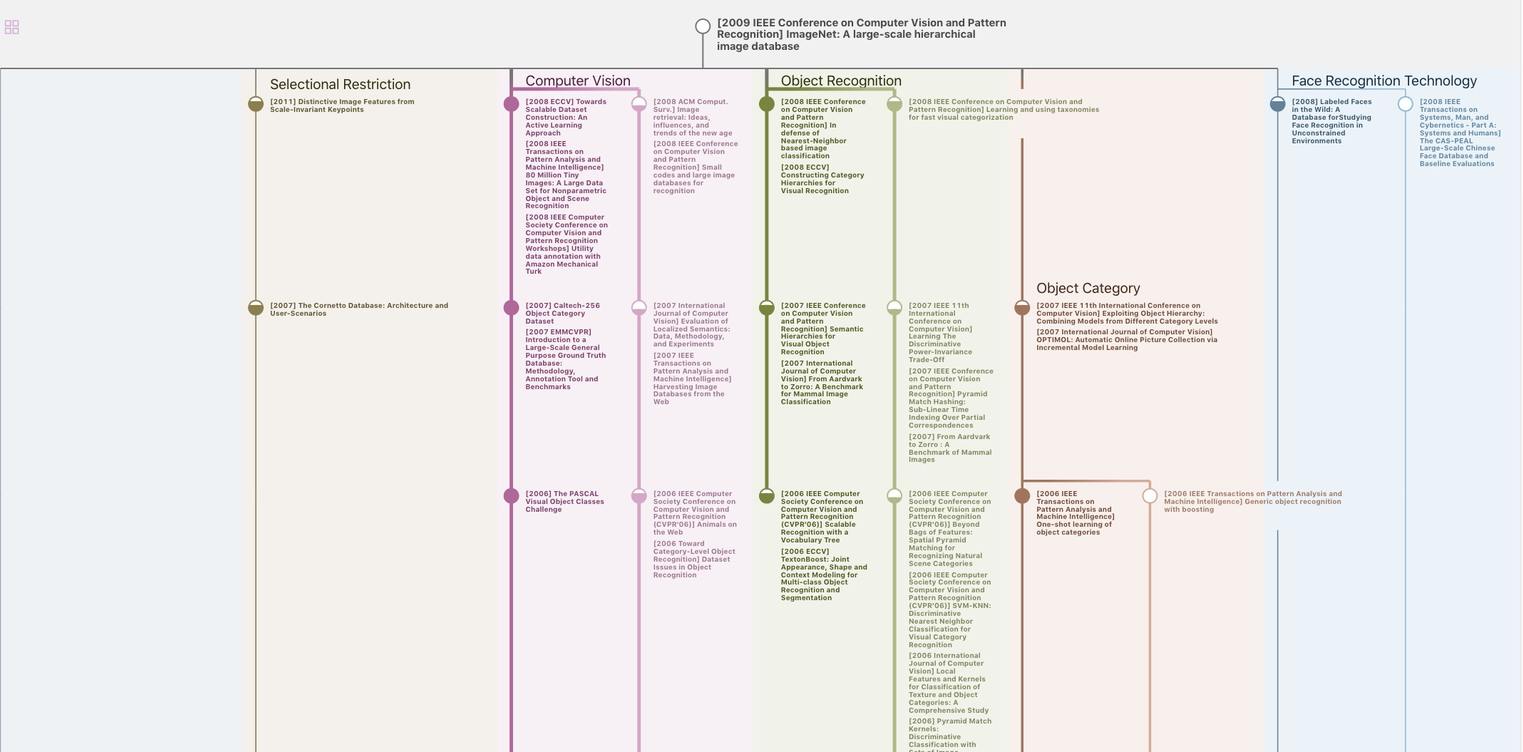
生成溯源树,研究论文发展脉络
Chat Paper
正在生成论文摘要