Enhanced Regularizers For Attributional Robustness
THIRTY-FIFTH AAAI CONFERENCE ON ARTIFICIAL INTELLIGENCE, THIRTY-THIRD CONFERENCE ON INNOVATIVE APPLICATIONS OF ARTIFICIAL INTELLIGENCE AND THE ELEVENTH SYMPOSIUM ON EDUCATIONAL ADVANCES IN ARTIFICIAL INTELLIGENCE(2021)
摘要
Deep neural networks are the default choice of learning models for computer vision tasks. Extensive work has been carried out in recent years on explaining deep models for vision tasks such as classification. However, recent work has shown that it is possible for these models to produce substantially different attribution maps even when two very similar images are given to the network, raising serious questions about trustworthiness. To address this issue, we propose a robust attribution training strategy to improve attributional robustness of deep neural networks. Our method carefully analyzes the requirements for attributional robustness and introduces two new regularizers that preserve a model's attribution map during attacks. Our method surpasses state-of-the-art attributional robustness methods by a margin of approximately 3% to 9% in terms of attribution robustness measures on several datasets including MNIST, FMNIST, Flower and GTSRB.
更多查看译文
关键词
robustness
AI 理解论文
溯源树
样例
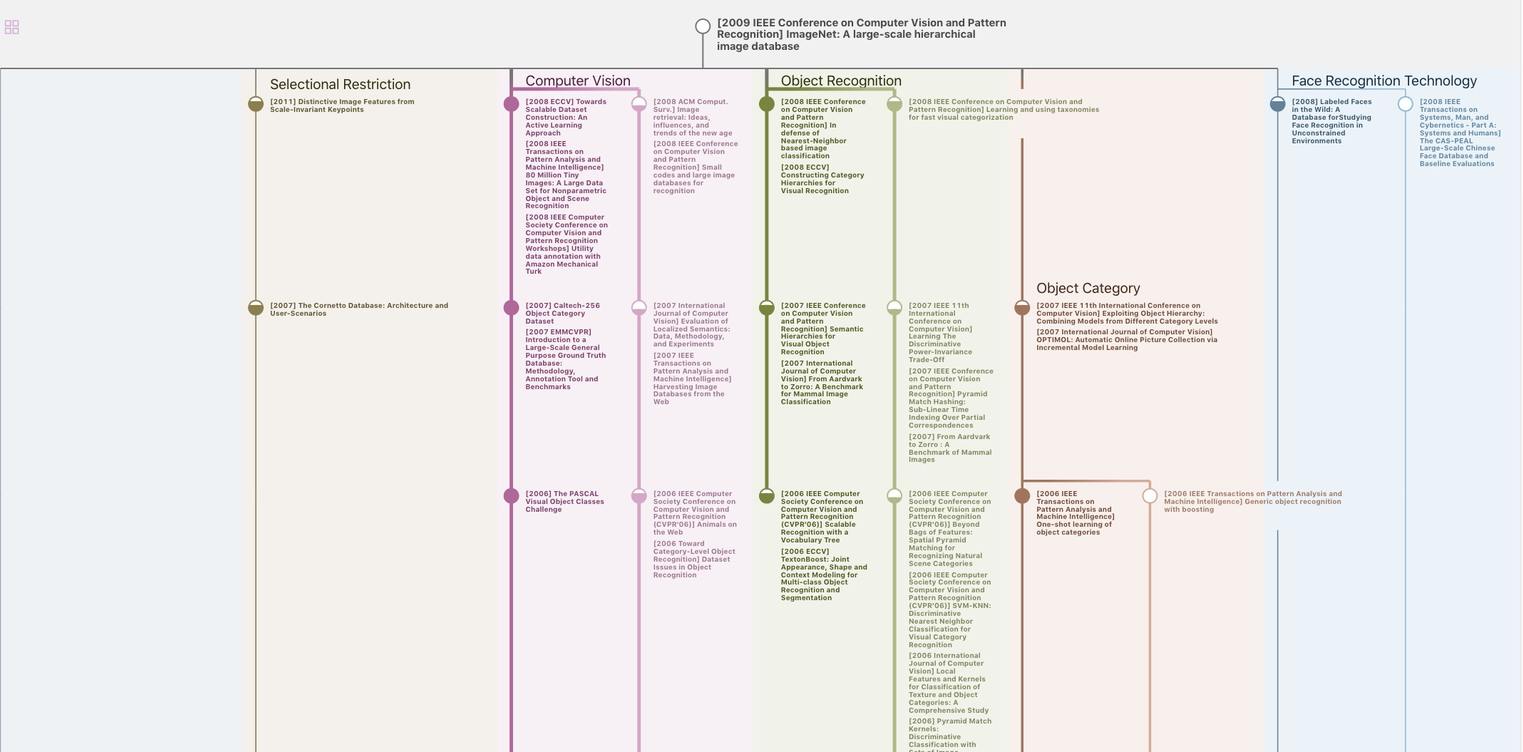
生成溯源树,研究论文发展脉络
Chat Paper
正在生成论文摘要