Data-efficient Weakly-supervised Learning for On-line Object Detection under Domain Shift in Robotics
arxiv(2020)
摘要
Several object detection methods have recently been proposed in the literature, the vast majority based on Deep Convolutional Neural Networks (DCNNs). Such architectures have been shown to achieve remarkable performance, at the cost of computationally expensive batch training and extensive labeling. These methods have important limitations for robotics: Learning solely on off-line data may introduce biases (the so-called domain shift), and prevents adaptation to novel tasks. In this work, we investigate how weakly-supervised learning can cope with these problems. We compare several techniques for weakly-supervised learning in detection pipelines to reduce model (re)training costs without compromising accuracy. In particular, we show that diversity sampling for constructing active learning queries and strong positives selection for self-supervised learning enable significant annotation savings and improve domain shift adaptation. By integrating our strategies into a hybrid DCNN/FALKON on-line detection pipeline [1], our method is able to be trained and updated efficiently with few labels, overcoming limitations of previous work. We experimentally validate and benchmark our method on challenging robotic object detection tasks under domain shift.
更多查看译文
关键词
robotics,detection,domain shift,data-efficient,weakly-supervised,on-line
AI 理解论文
溯源树
样例
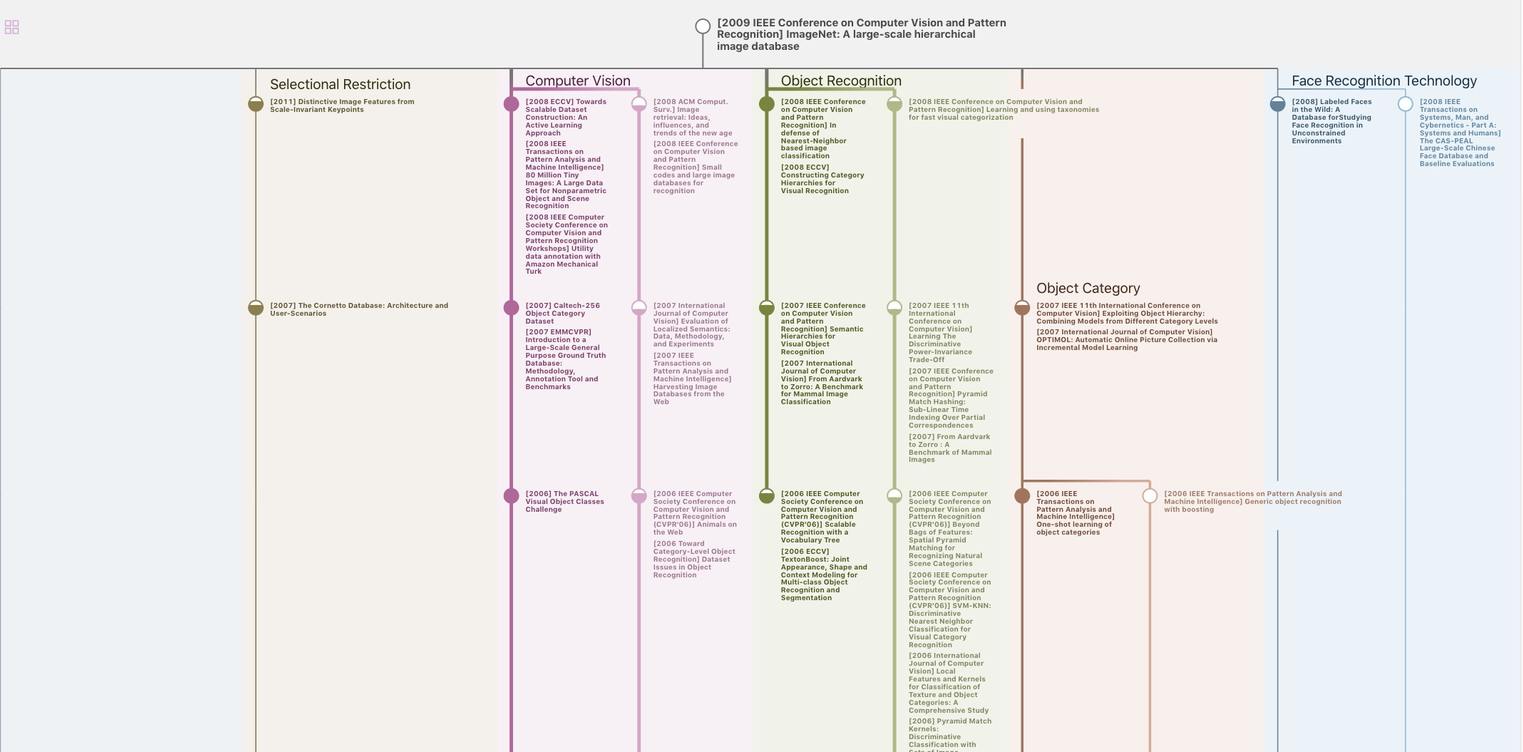
生成溯源树,研究论文发展脉络
Chat Paper
正在生成论文摘要