Spatio-Temporal Modeling For Forecasting High-Risk Freshwater Cyanobacterial Harmful Algal Blooms In Florida
FRONTIERS IN ENVIRONMENTAL SCIENCE(2020)
摘要
Due to the occurrence of more frequent and widespread toxic cyanobacteria events, the ability to predict freshwater cyanobacteria harmful algal blooms (cyanoHAB) is of critical importance for the management of drinking and recreational waters. Lake system specific geographic variation of cyanoHABs has been reported, but regional and state level variation is infrequently examined. A spatio-temporal modeling approach can be applied, via the computationally efficient Integrated Nested Laplace Approximation (INLA), to high-risk cyanoHAB exceedance rates to explore spatio-temporal variations across statewide geographic scales. We explore the potential for using satellite-derived data and environmental determinants to develop a short-term forecasting tool for cyanobacteria presence at varying space-time domains for the state of Florida. Weekly cyanobacteria abundance data were obtained using Sentinel-3 Ocean Land Color Imagery (OLCI), for a period of May 2016-June 2019. Time and space varying covariates include surface water temperature, ambient temperature, precipitation, and lake geomorphology. The hierarchical Bayesian spatio-temporal modeling approach in R-INLA represents a potential forecasting tool useful for water managers and associated public health applications for predicting near future high-risk cyanoHAB occurrence given the spatio-temporal characteristics of these events in the recent past. This method is robust to missing data and unbalanced sampling between waterbodies, both common issues in water quality datasets.
更多查看译文
关键词
harmful algal blooms, cyanobacteria, hierarchical Bayes, integrated nested Laplace approximation, remote sensing, predictive modeling
AI 理解论文
溯源树
样例
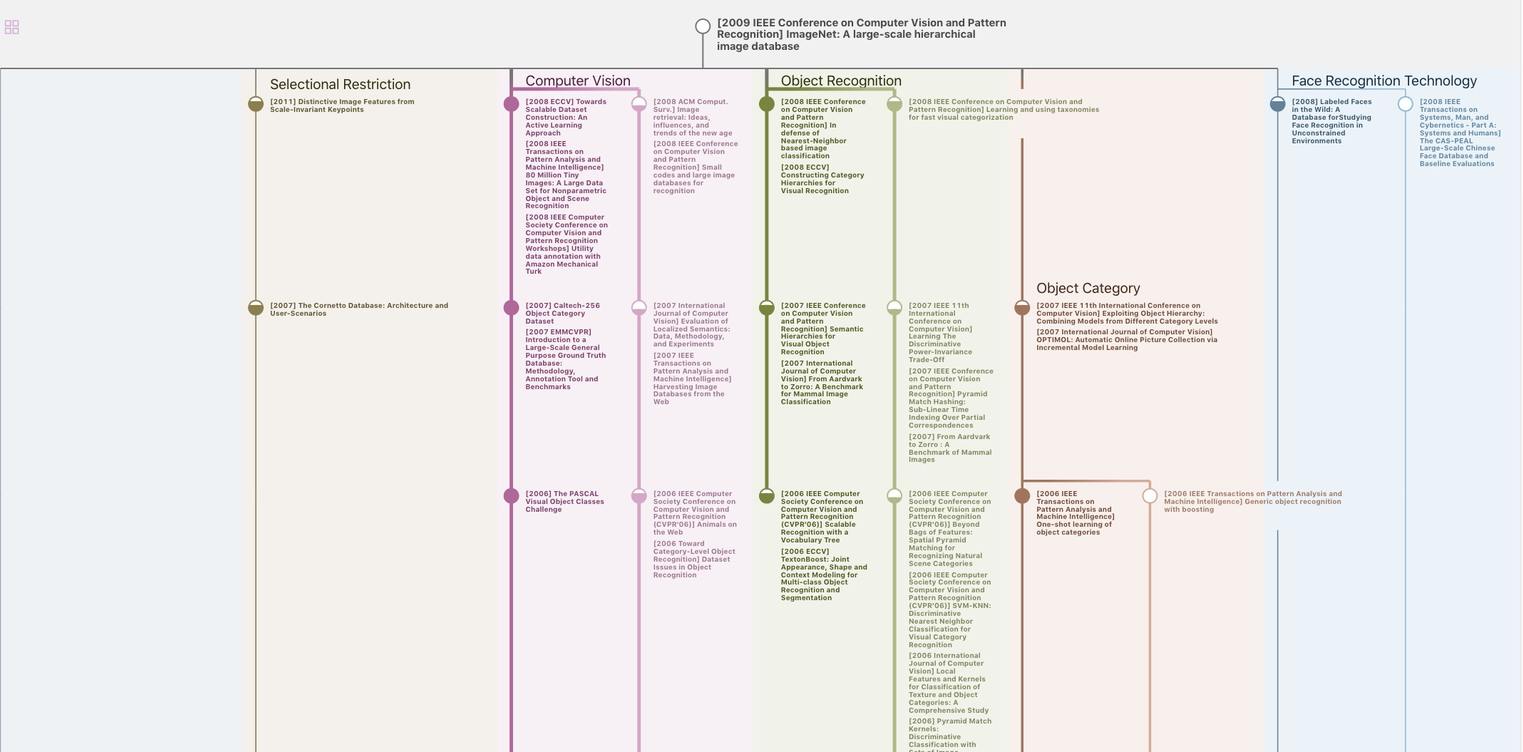
生成溯源树,研究论文发展脉络
Chat Paper
正在生成论文摘要