Spatial Contrastive Learning for Few-Shot Classification
MACHINE LEARNING AND KNOWLEDGE DISCOVERY IN DATABASES(2021)
摘要
In this paper we explore contrastive learning for few-shot classification, in which we propose to use it as an additional auxiliary training objective acting as a data-dependent regularizer to promote more general and transferable features. In particular, we present a novel attention-based spatial contrastive objective to learn locally discriminative and class-agnostic features. As a result, our approach overcomes some of the limitations of the cross-entropy loss, such as its excessive discrimination towards seen classes, which reduces the transferability of features to unseen classes. With extensive experiments, we show that the proposed method outperforms state-of-the-art approaches, confirming the importance of learning good and transferable embeddings for few-shot learning. Code: https://github.com/yassouali/SCL.
更多查看译文
关键词
Few-shot learning, Contrastive learning, Deep learning
AI 理解论文
溯源树
样例
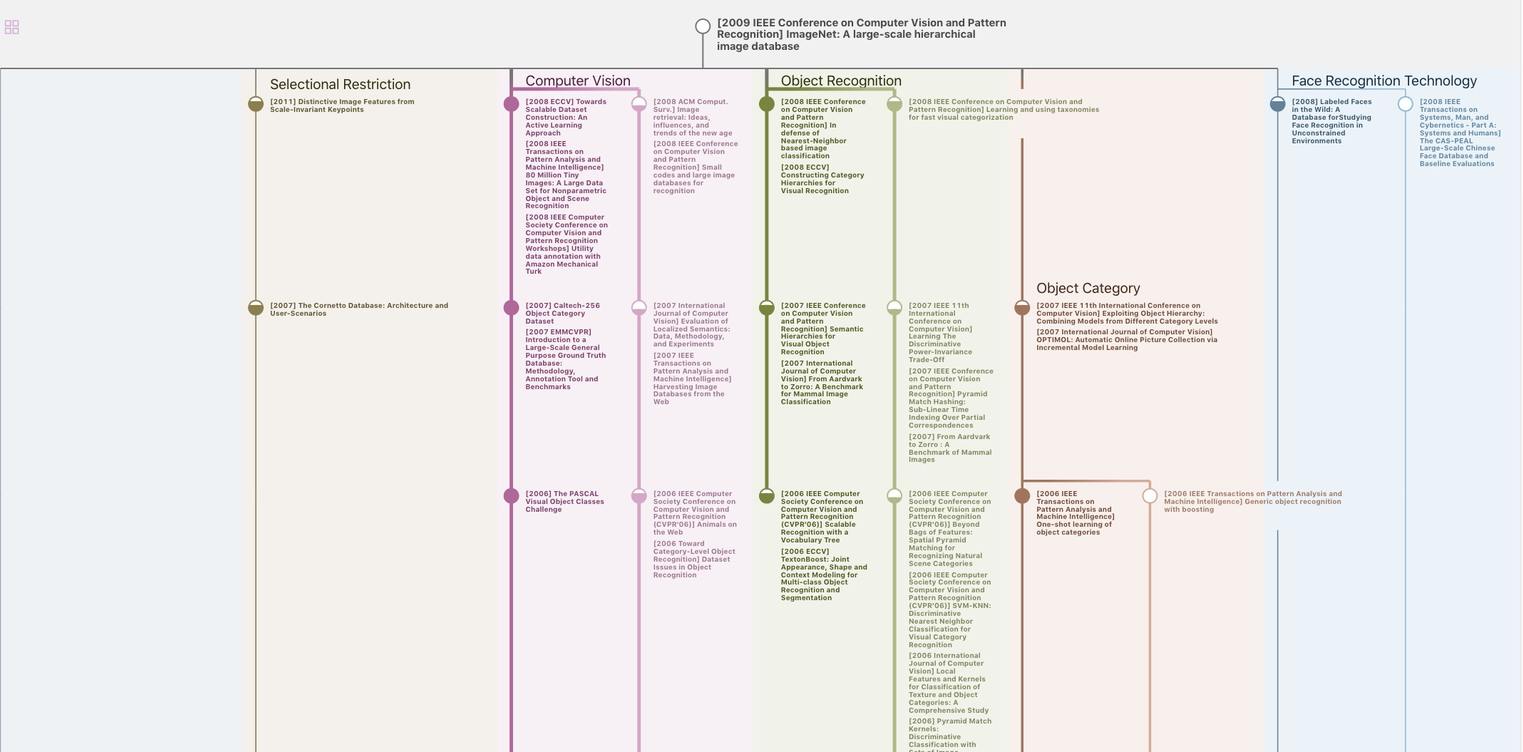
生成溯源树,研究论文发展脉络
Chat Paper
正在生成论文摘要