Global Context Networks
IEEE transactions on pattern analysis and machine intelligence(2023)
摘要
The non-local network (NLNet) presents a pioneering approach for capturing long-range dependencies within an image, via aggregating query-specific global context to each query position. However, through a rigorous empirical analysis, we have found that the global contexts modeled by the non-local network are almost the same for different query positions. In this paper, we take advantage of this finding to create a simplified network based on a query-independent formulation, which maintains the accuracy of NLNet but with significantly less computation. We further replace the one-layer transformation function of the non-local block by a two-layer bottleneck, which further reduces the parameter number considerably. The resulting network element, called the global context (GC) block, effectively models global context in a lightweight manner, allowing it to be applied at multiple layers of a backbone network to form a global context network (GCNet). Experiments show that GCNet generally outperforms NLNet on major benchmarks for various recognition tasks. The code and network configurations are available at https://github.com/xvjiarui/GCNet.
更多查看译文
关键词
deep network,self-attention model,global context,object detection
AI 理解论文
溯源树
样例
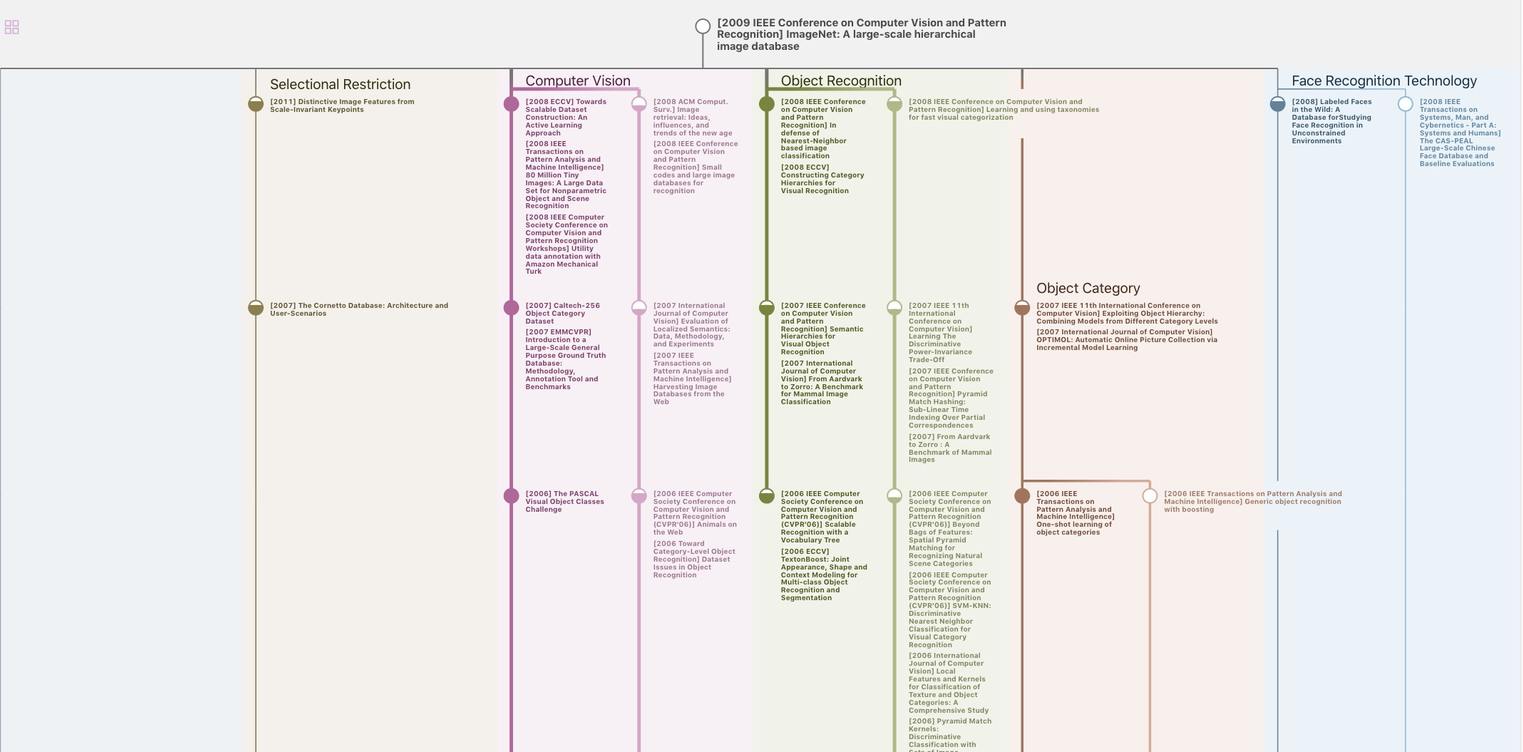
生成溯源树,研究论文发展脉络
Chat Paper
正在生成论文摘要