Hcgrid: A Convolution-Based Gridding Framework For Radio Astronomy In Hybrid Computing Environments
MONTHLY NOTICES OF THE ROYAL ASTRONOMICAL SOCIETY(2021)
摘要
Gridding operation, which is to map non-uniform data samples on to a uniformly distributed grid, is one of the key steps in radio astronomical data reduction process. One of the main bottlenecks of gridding is the poor computing performance, and a typical solution for such performance issue is the implementation of multicore CPU platforms. Although such a method could usually achieve good results, in many cases, the performance of gridding is still restricted to an extent due to the limitations of CPU, since the main workload of gridding is a combination of a large number of single instruction, multidata stream operations, which is more suitable for GPU, rather than CPU implementations. To meet the challenge of massive data gridding for the modern large single-dish radio telescopes, e.g. the Five-hundred-meter Aperture Spherical radio Telescope, inspired by existing multicore CPU gridding algorithms such as Cygrid, here we present an easy-to-install, high-performance, and open-source convolutional gridding framework, HCGrid, in CPU-GPU heterogeneous platforms. It optimizes data search by employing multithreading on CPU, and accelerates the convolution process by utilizing massive parallelization of GPU. In order to make HCGrid a more adaptive solution, we also propose the strategies of thread organization and coarsening, as well as optimal parameter settings under various GPU architectures. A thorough analysis of computing time and performance gain with several GPU parallel optimization strategies show that it can lead to excellent performance in hybrid computing environments.
更多查看译文
关键词
methods: data analysis, techniques: image processing, software: public release
AI 理解论文
溯源树
样例
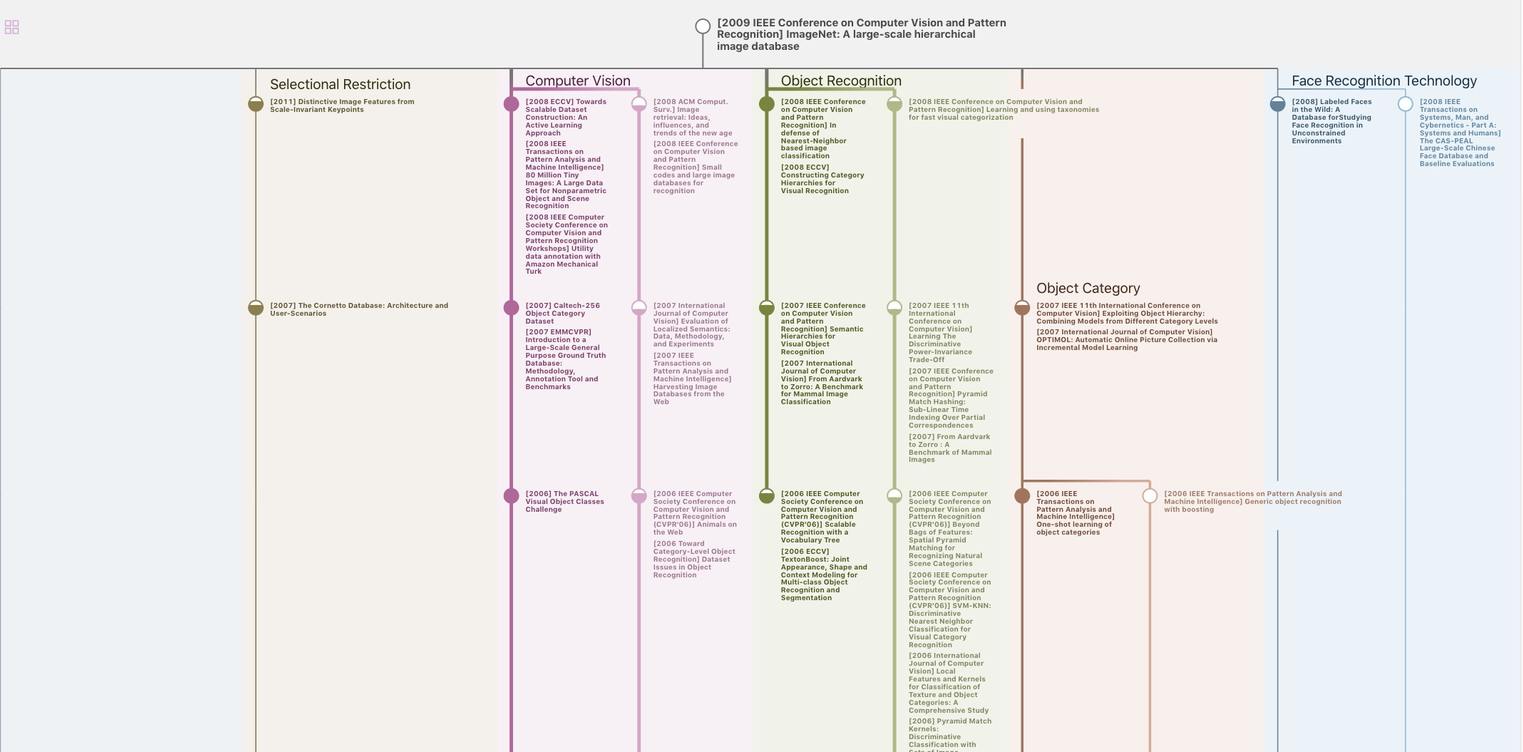
生成溯源树,研究论文发展脉络
Chat Paper
正在生成论文摘要