An Efficient Recurrent Adversarial Framework for Unsupervised Real-Time Video Enhancement
International Journal of Computer Vision(2023)
摘要
Video enhancement is a challenging problem, more than that of stills, mainly due to high computational cost, larger data volumes and the difficulty of achieving consistency in the spatio-temporal domain. In practice, these challenges are often coupled with the lack of example pairs, which inhibits the application of supervised learning strategies. To address these challenges, we propose an efficient adversarial video enhancement framework that learns directly from unpaired video examples. In particular, our framework introduces new recurrent cells that consist of interleaved local and global modules for implicit integration of spatial and temporal information. The proposed design allows our recurrent cells to efficiently propagate spatio-temporal information across frames and reduces the need for high complexity networks. Our setting enables learning from unpaired videos in a cyclic adversarial manner, where the proposed recurrent units are employed in all architectures. Efficient training is accomplished by introducing one single discriminator that learns the joint distribution of source and target domain simultaneously. The enhancement results demonstrate clear superiority of the proposed video enhancer over the state-of-the-art methods, in all terms of visual quality, quantitative metrics, and inference speed. Notably, our video enhancer is capable of enhancing over 35 frames per second of FullHD video (1080x1920).
更多查看译文
关键词
Video enhancement, Video quality mapping, Real-time, Recurrent networks, Generative adversarial networks, Joint distribution learning
AI 理解论文
溯源树
样例
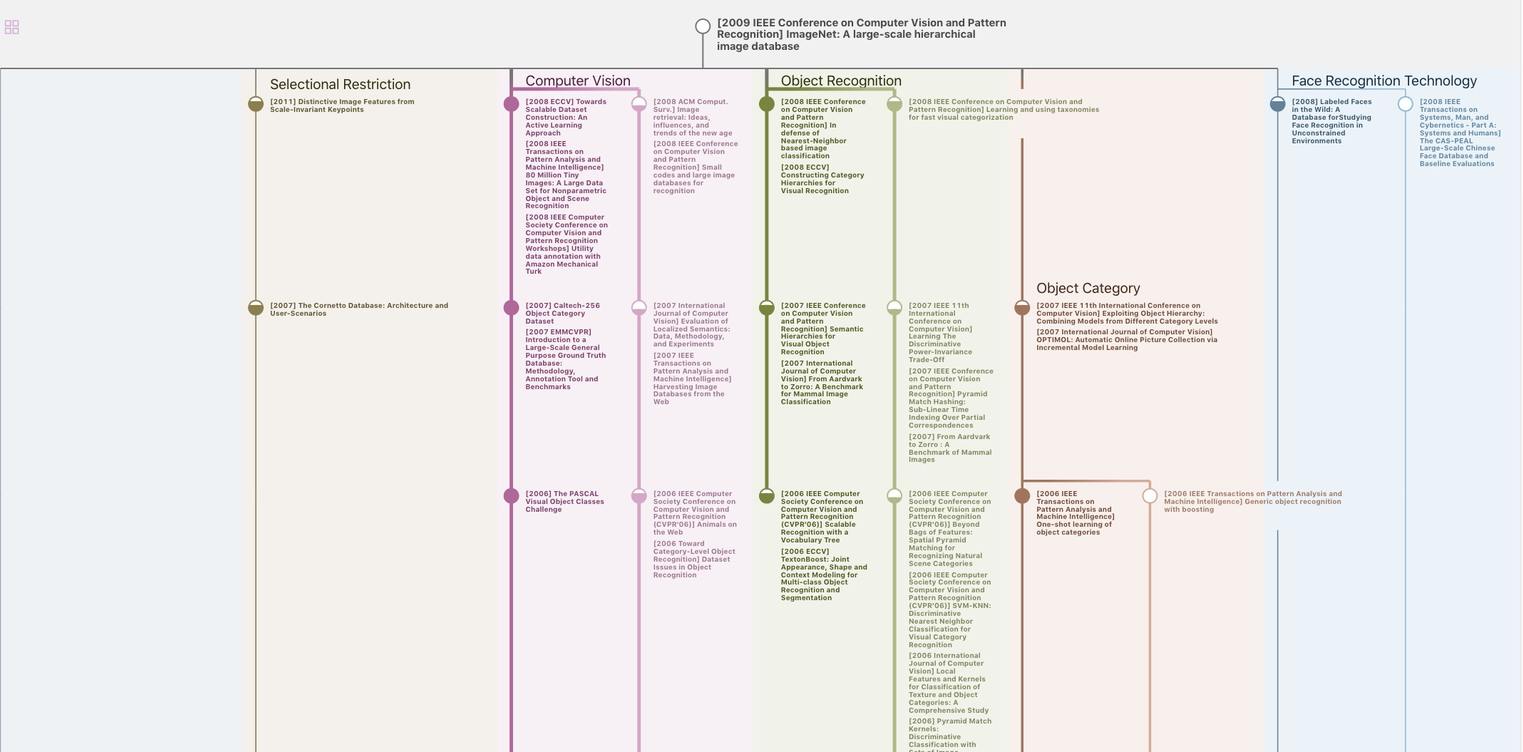
生成溯源树,研究论文发展脉络
Chat Paper
正在生成论文摘要