Confidence Intervals By Constrained Optimization-An Algorithm And Software Package For Practical Identifiability Analysis In Systems Biology
PLOS COMPUTATIONAL BIOLOGY(2020)
摘要
Practical identifiability of Systems Biology models has received a lot of attention in recent scientific research. It addresses the crucial question for models' predictability: how accurately can the models' parameters be recovered from available experimental data. The methods based on profile likelihood are among the most reliable methods of practical identification. However, these methods are often computationally demanding or lead to inaccurate estimations of parameters' confidence intervals. Development of methods, which can accurately produce parameters' confidence intervals in reasonable computational time, is of utmost importance for Systems Biology and QSP modeling.We propose an algorithm Confidence Intervals by Constraint Optimization (CICO) based on profile likelihood, designed to speed-up confidence intervals estimation and reduce computational cost. The numerical implementation of the algorithm includes settings to control the accuracy of confidence intervals estimates. The algorithm was tested on a number of Systems Biology models, including Taxol treatment model and STAT5 Dimerization model, discussed in the current article.The CICO algorithm in a software package freely available in Julia (https://github.com/insysbio/LikelihoodProfiler.jl) and Python (https://github.com/insysbio/LikelihoodProfiler. py).Author summaryDifferential equations-based models are widely used in Systems Biology and Quantitative Systems Pharmacology and play a significant role in the discovery of new disease-directed drugs. Complexity of models is a trade off from their employment to crucial fields of biology and medicine. These areas of application require large non-linear models with many unknown parameters. How accurately can the parameters of a model be recovered from experimental data? What is the identifiable subset of parameters? Can the model be reduced or reparameterized to become identifiable? All those questions of identifiability analysis are essential for model's predictability and reliability. That explains why the topic of identifiability of Systems Biology models has received a lot of attention in recent scientific research. However, existing numerical methods of identifiability analysis are computationally demanding or often lead to inaccurate estimations. Development of methods, which can accurately produce parameters' confidence intervals in reasonable computational time, is of utmost importance for Systems Biology and QSP modeling. We propose an algorithm and a software package to test identifiability of Systems Biology models, designed to speed-up confidence intervals estimation and reduce computational cost. The software package was tested on a number of Systems Biology models, including Taxol treatment model and STAT5 Dimerization model, discussed in the current article.
更多查看译文
AI 理解论文
溯源树
样例
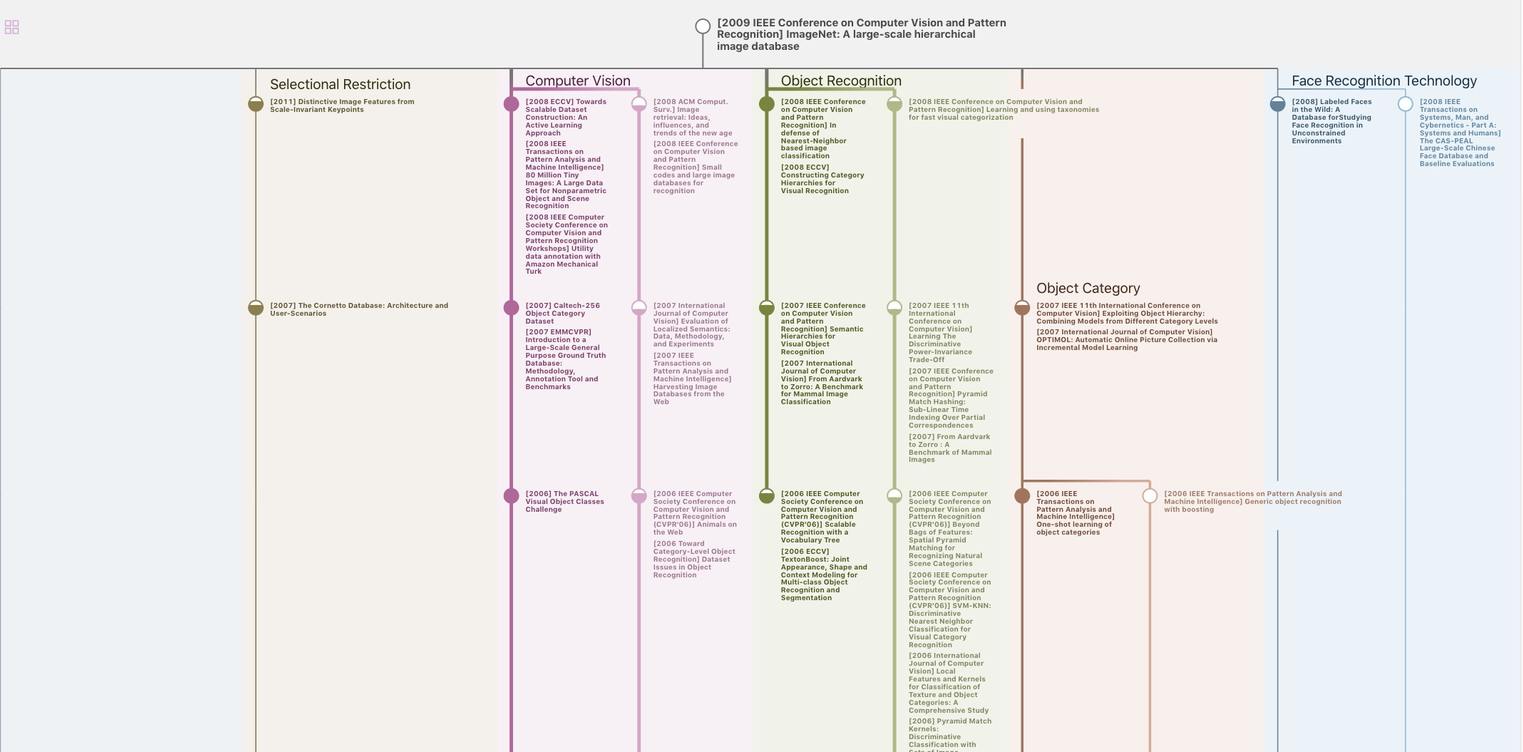
生成溯源树,研究论文发展脉络
Chat Paper
正在生成论文摘要