Off-Policy Optimization of Portfolio Allocation Policies under Constraints
arxiv(2020)
摘要
The dynamic portfolio optimization problem in finance frequently requires learning policies that adhere to various constraints, driven by investor preferences and risk. We motivate this problem of finding an allocation policy within a sequential decision making framework and study the effects of: (a) using data collected under previously employed policies, which may be sub-optimal and constraint-violating, and (b) imposing desired constraints while computing near-optimal policies with this data. Our framework relies on solving a minimax objective, where one player evaluates policies via off-policy estimators, and the opponent uses an online learning strategy to control constraint violations. We extensively investigate various choices for off-policy estimation and their corresponding optimization sub-routines, and quantify their impact on computing constraint-aware allocation policies. Our study shows promising results for constructing such policies when back-tested on historical equities data, under various regimes of operation, dimensionality and constraints.
更多查看译文
关键词
portfolio allocation policies,optimization,constraints,off-policy
AI 理解论文
溯源树
样例
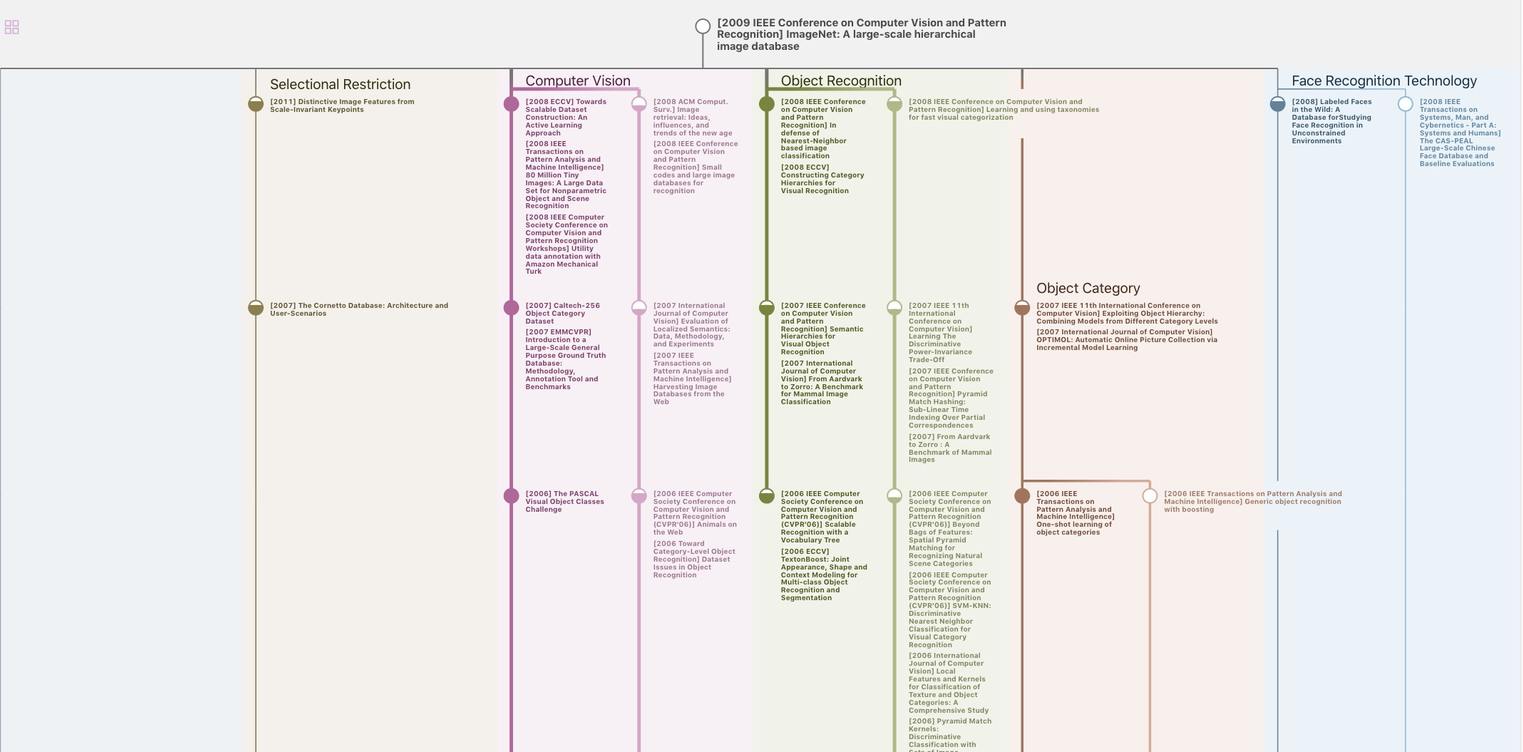
生成溯源树,研究论文发展脉络
Chat Paper
正在生成论文摘要