Unsupervised in-distribution anomaly detection of new physics through conditional density estimation
arxiv(2020)
摘要
Anomaly detection is a key application of machine learning, but is generally focused on the detection of outlying samples in the low probability density regions of data. Here we instead present and motivate a method for unsupervised in-distribution anomaly detection using a conditional density estimator, designed to find unique, yet completely unknown, sets of samples residing in high probability density regions. We apply this method towards the detection of new physics in simulated Large Hadron Collider (LHC) particle collisions as part of the 2020 LHC Olympics blind challenge, and show how we detected a new particle appearing in only 0.08% of 1 million collision events. The results we present are our original blind submission to the 2020 LHC Olympics, where it achieved the state-of-the-art performance.
更多查看译文
关键词
anomaly detection,conditional density estimation,new physics,in-distribution
AI 理解论文
溯源树
样例
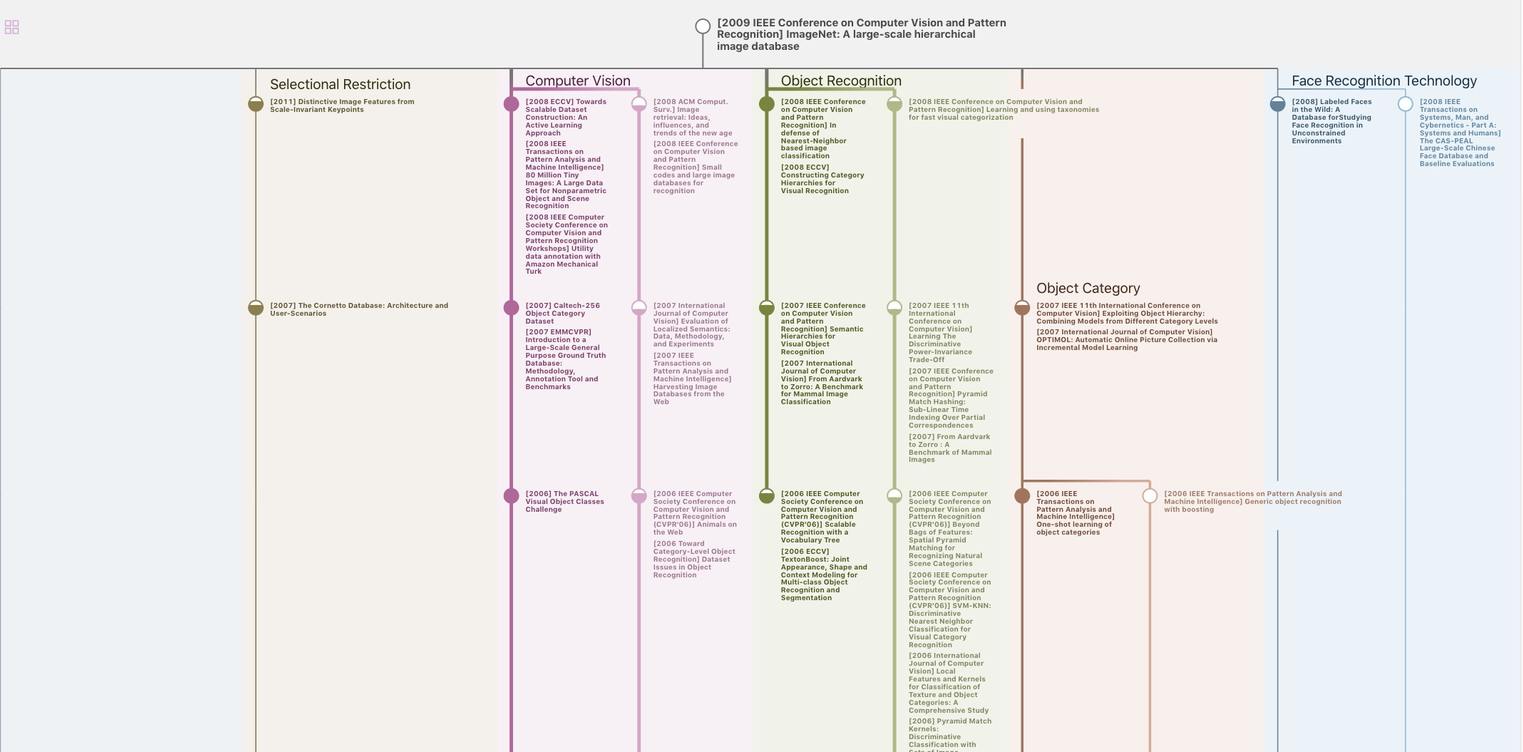
生成溯源树,研究论文发展脉络
Chat Paper
正在生成论文摘要