Data assimilation for flow forecasting in urban drainage systems by updating a hydrodynamic model of Damhusaen Catchment, Copenhagen
URBAN WATER JOURNAL(2020)
摘要
Accurate model-based forecasts (discharge and water level) are considered significant for efficient planning and management of urban drainage systems. These model-based predictions can be improved by assimilating system measurements in physically based, distributed, 1D hydrodynamic urban drainage models. In the present research, a combined filtering and error forecast method was applied for the data assimilation to update the states of the urban drainage model. The developed data assimilation set-up in combination with the 1D hydrodynamic model was applied at the Damhusaen Catchment, Copenhagen. Discharge assimilation represented significant potential to update model forecast, and maximum volume error was reduced by 22% and 6% at two verification locations. The assimilation of water levels had a minor impact on the update of the system states. The updated forecast skill using error forecast models was enhanced up to 1-2 hours and 6-7 hours lead time at upstream assimilation and downstream verification locations, respectively.
更多查看译文
关键词
Filtering algorithm,auto-regressive model,data assimilation,1D hydrodynamic model,updating
AI 理解论文
溯源树
样例
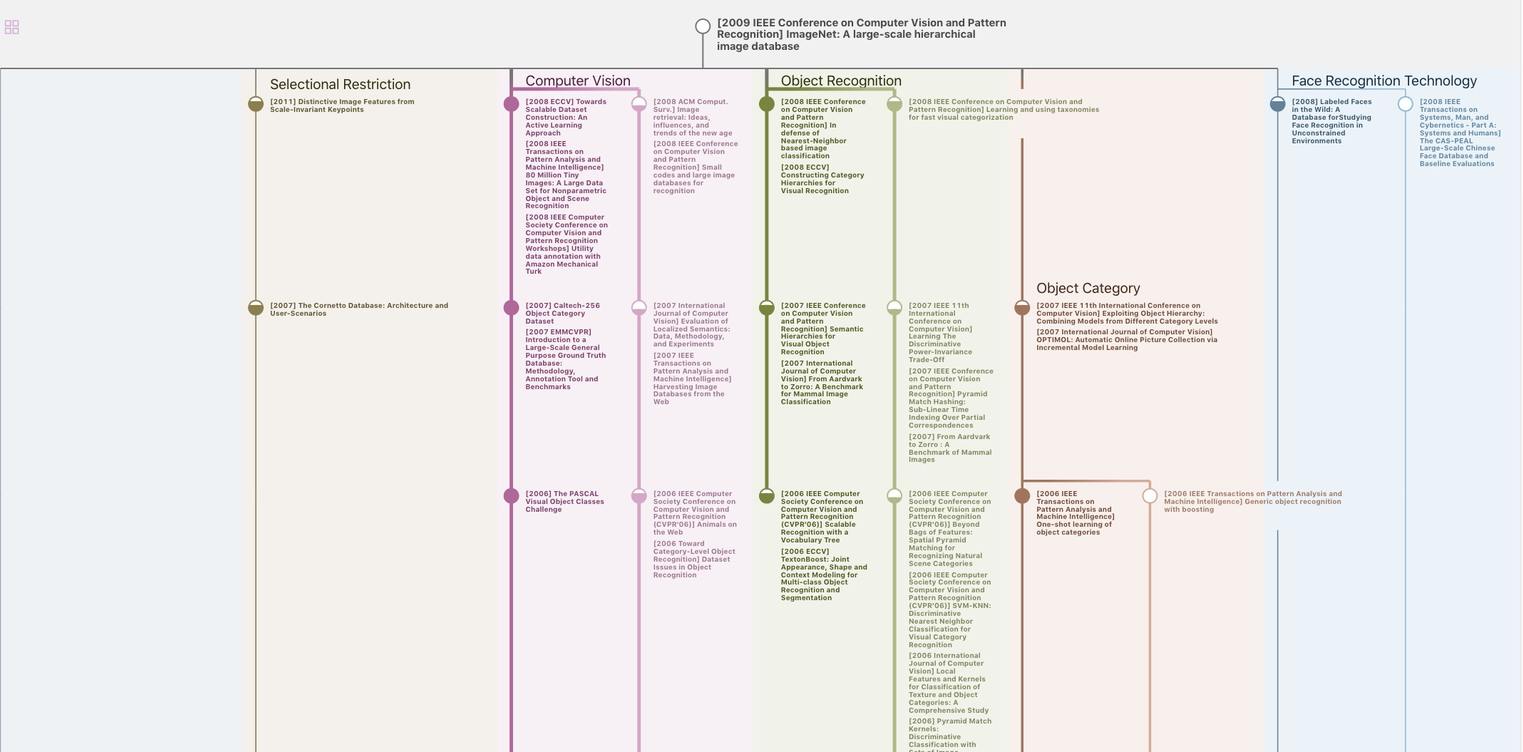
生成溯源树,研究论文发展脉络
Chat Paper
正在生成论文摘要