Amplifying the Anterior-Posterior Difference via Data Enhancement -- A More Robust Deep Monocular Orientation Estimation Solution
arxiv(2020)
摘要
Existing deep-learning based monocular orientation estimation algorithms faces the problem of confusion between the anterior and posterior parts of the objects, caused by the feature similarity of such parts in typical objects in traffic scenes such as cars and pedestrians. While difficult to solve, the problem may lead to serious orientation estimation errors, and pose threats to the upcoming decision making process of the ego vehicle, since the predicted tracks of objects may have directions opposite to ground truths. In this paper, we mitigate this problem by proposing a pretraining method. The method focuses on predicting the left/right semicircle in which the orientation of the object is located. The trained semicircle prediction model is then integrated into the orientation angle estimation model which predicts a value in range $[0, \pi]$. Experiment results show that the proposed semicircle prediction enhances the accuracy of orientation estimation, and mitigates the problem stated above. With the proposed method, a backbone achieves similar state-of-the-art orientation estimation performance to existing approaches with well-designed network structures.
更多查看译文
关键词
orientation,data enhancement,anterior-posterior
AI 理解论文
溯源树
样例
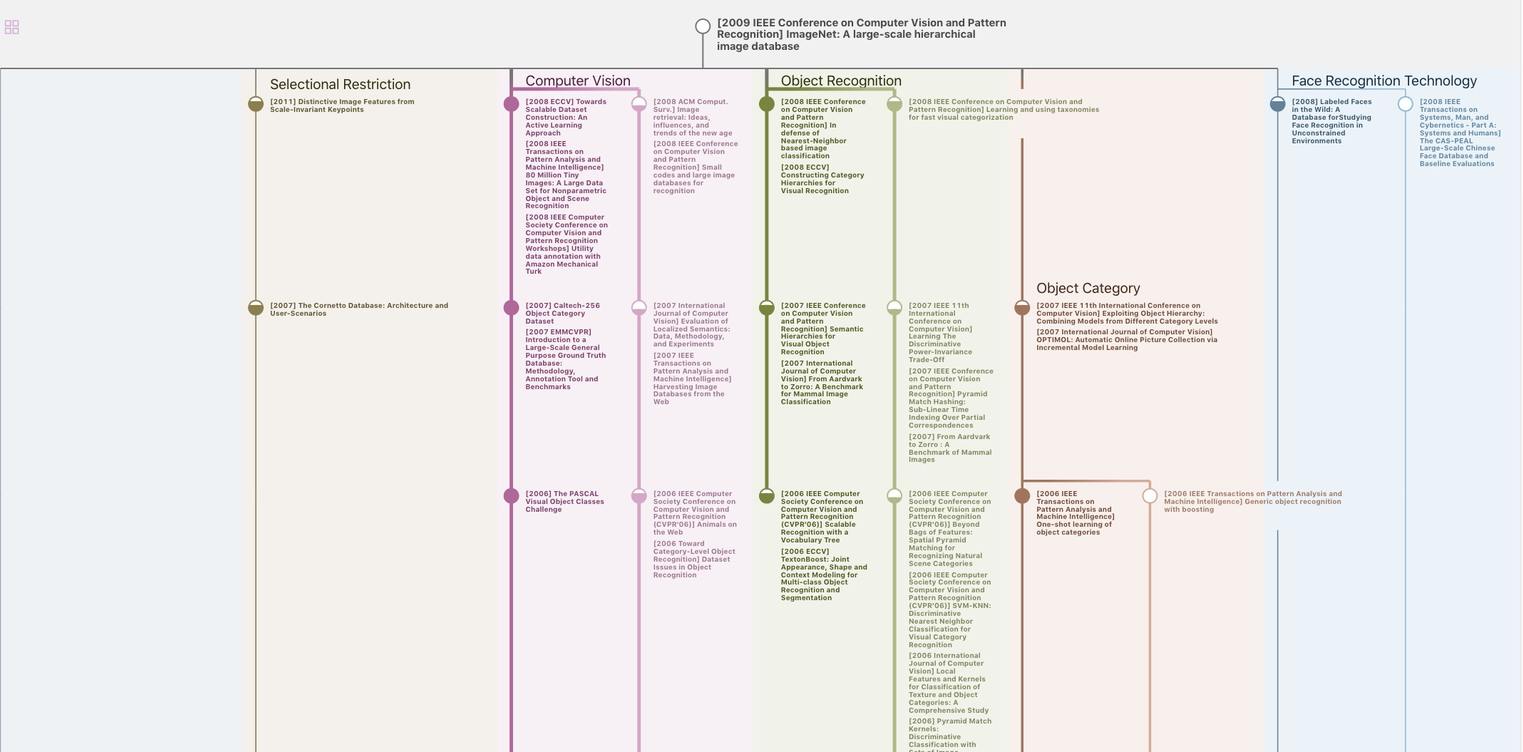
生成溯源树,研究论文发展脉络
Chat Paper
正在生成论文摘要