Measurement bias: a structure perspective
arxiv(2020)
摘要
Objectives: To propose new causal structures to clarify the structures and mechanisms of measurement bias (MB). Methods: We propose a new structure for measuring one singleton variable firstly, and then extend it into clarifying the effect between an exposure and an outcome, aided by the Directed Acyclic Graphs (DAGs). Results: The MB for one singleton variable arises in the selection of an imperfect I/O device-like measurement system only. For effect estimation, however, extra source of MB arises from any redundant association between a measured exposure and a measured outcome. The misclassification will be bidirectionally differential for a common outcome, unidirectionally differential for a causal relation, and non-differential for a common cause or an independence, between the measured exposure and the measured outcome. The measured exposure can actually affect the measured outcome, or vice versa. Reverse causality is a concept defined at the level of measurement. Conclusions: Measurement extends the causality from real world to human thinking. MB has two origins: an imperfect measurement system and any redundant association at the measurement level. Our new DAGs have clarified the structures and mechanisms of MB.
更多查看译文
关键词
measurement bias,structure perspective
AI 理解论文
溯源树
样例
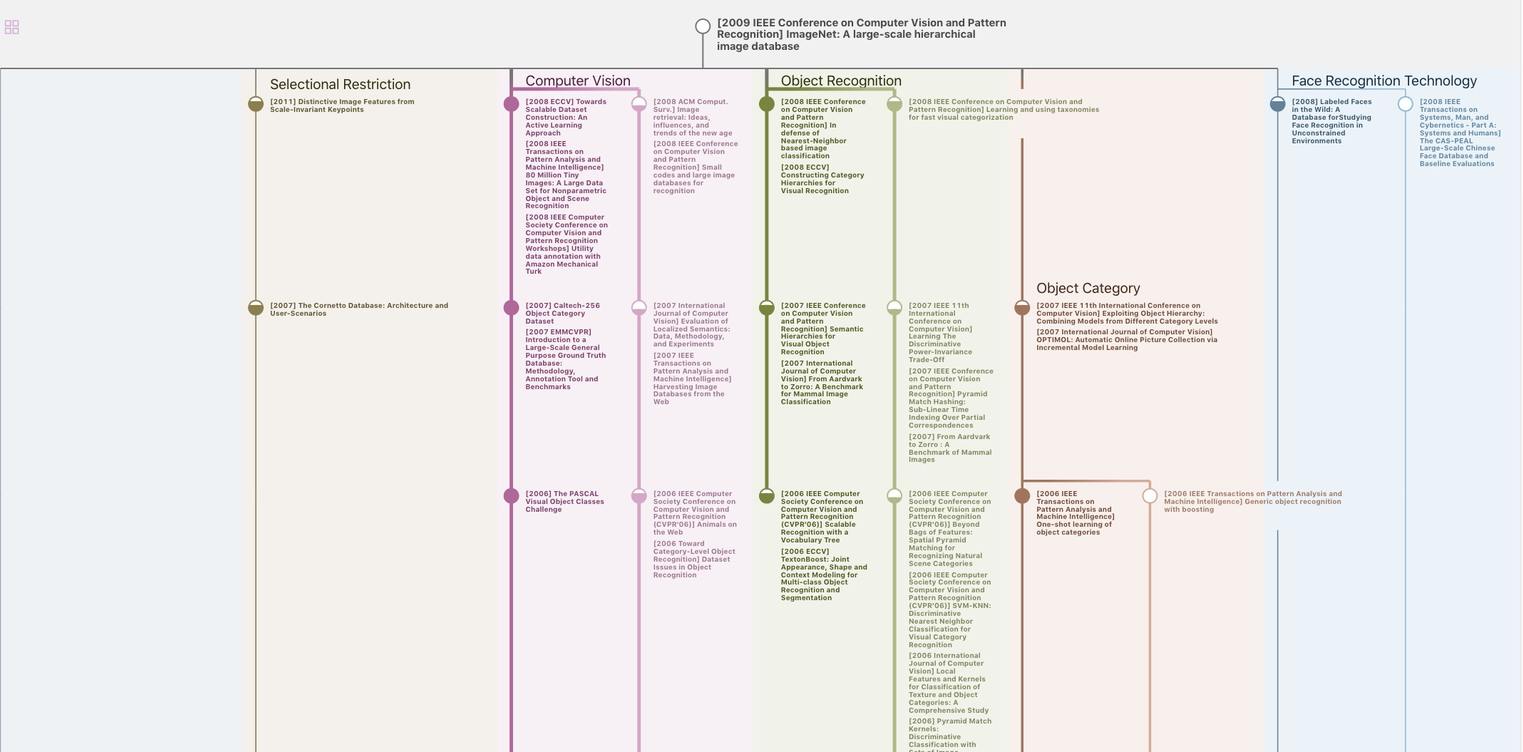
生成溯源树,研究论文发展脉络
Chat Paper
正在生成论文摘要