Identification Of Patients With Pancreatic Cancer By Electron Paramagnetic Resonance Spectroscopy Of Fatty Acid Binding To Human Serum Albumin
ACS PHARMACOLOGY & TRANSLATIONAL SCIENCE(2020)
摘要
An effective biological marker for pancreatic adenocarcinoma (PAC) is not available so far. Here, we investigate how electron paramagnetic resonance (EPR) spectroscopy of spin-labeled fatty acid (FA) molecules binding to human serum albumin (HSA) in human serum is a suitable method for the identification of patients with PAC through detection of PAC-induced changes of FA binding to albumin. The functionality of HSA to bind FA is investigated in serum samples of 35 patients with PAC, 26 patients with benign pancreatic tumors (BPD), and 24 healthy individuals by continuous wave (CW) EPR spectroscopy by simply dissolving 16-DOXYL stearic acid as spin-labeled FA. It is found that FA binding to HSA in PAC is significantly modified when compared with healthy and BPD individuals. The PAC group could best be discriminated from the healthy group based on EPR characteristics at the loading ratio of 1:4 (HSA:FA), while patients with PAC and BPD are distinguishable at a loading ratio of 1:6. Using nanoscale distance measurements through double electron-electron resonance (DEER), it is found that the distribution of FAs in the HSA of one PAC patient is similar to that of FAs in healthy individuals. Combining all EPR spectroscopic data, this leads to a tentative molecular interpretation of only small changes in hydration at the protein's surface as origin of the detectable characteristics for PAC patients. Thus, EPR of FA/HSA binding is a simple and promising tool for clinical detection of patients with PAC and needs to be tested with larger ensembles of different patient groups.
更多查看译文
关键词
albumin, electron spin/paramagnetic resonance spectroscopy, ESR/EPR, DEER, pancreatic cancer, pancreatic adenocarcinoma, IPMN, precancerous pancreatic lesion, tumor marker, biological marker
AI 理解论文
溯源树
样例
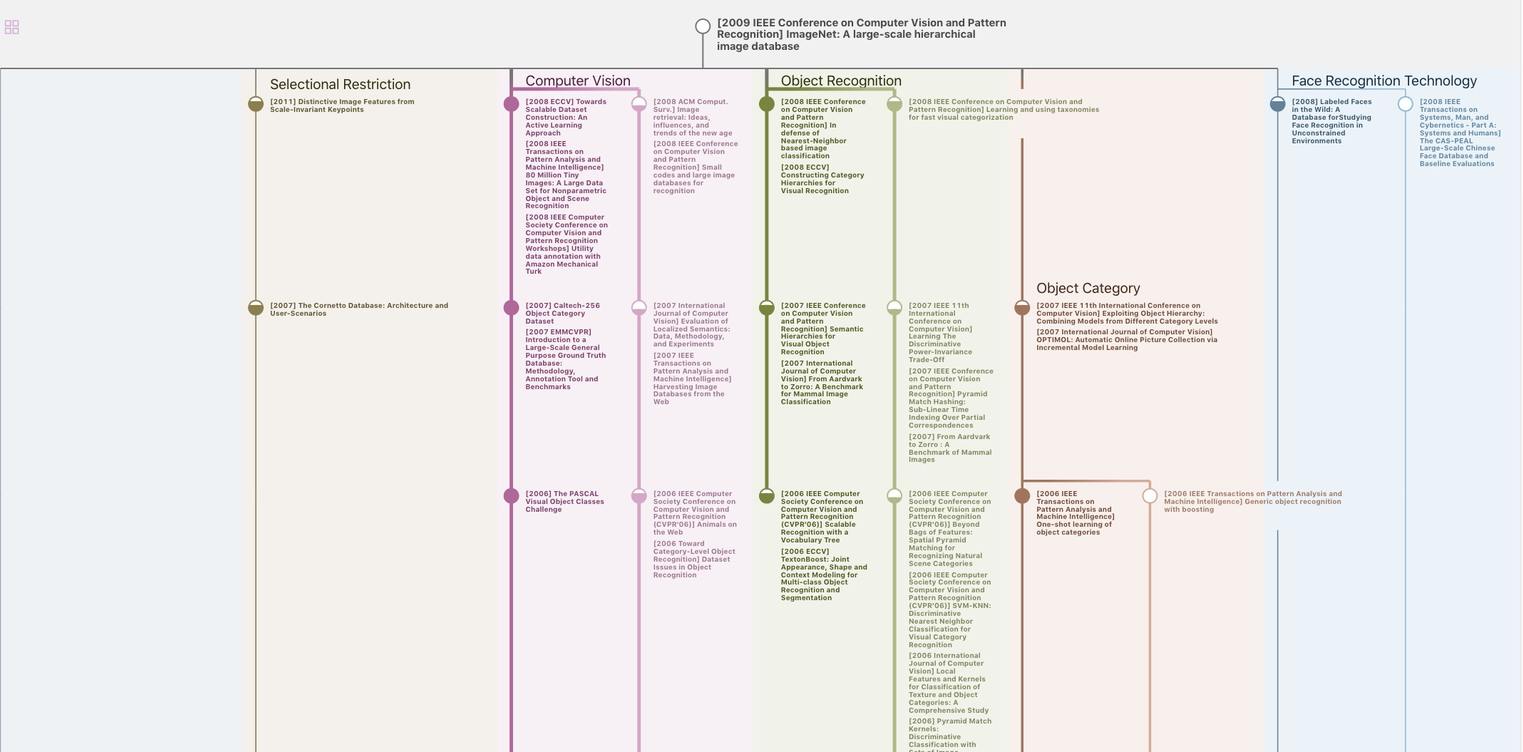
生成溯源树,研究论文发展脉络
Chat Paper
正在生成论文摘要