CityLearn: Standardizing Research in Multi-Agent Reinforcement Learning for Demand Response and Urban Energy Management
arxiv(2020)
摘要
Rapid urbanization, increasing integration of distributed renewable energy resources, energy storage, and electric vehicles introduce new challenges for the power grid. In the US, buildings represent about 70% of the total electricity demand and demand response has the potential for reducing peaks of electricity by about 20%. Unlocking this potential requires control systems that operate on distributed systems, ideally data-driven and model-free. For this, reinforcement learning (RL) algorithms have gained increased interest in the past years. However, research in RL for demand response has been lacking the level of standardization that propelled the enormous progress in RL research in the computer science community. To remedy this, we created CityLearn, an OpenAI Gym Environment which allows researchers to implement, share, replicate, and compare their implementations of RL for demand response. Here, we discuss this environment and The CityLearn Challenge, a RL competition we organized to propel further progress in this field.
更多查看译文
关键词
urban energy management,reinforcement,demand response,multi-agent
AI 理解论文
溯源树
样例
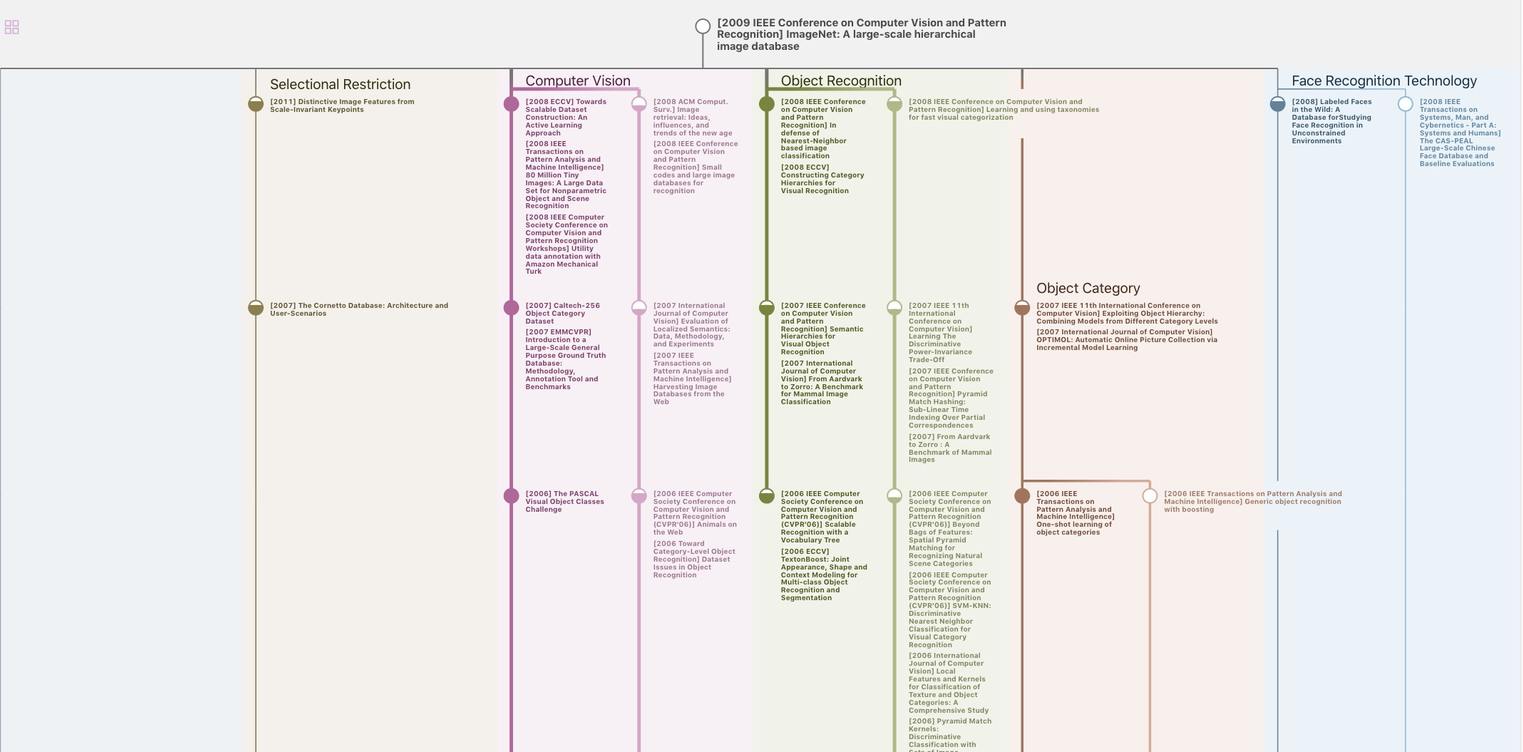
生成溯源树,研究论文发展脉络
Chat Paper
正在生成论文摘要