Upper and Lower Bounds on the Performance of Kernel PCA
arxiv(2023)
摘要
Principal Component Analysis (PCA) is a popular method for dimension reduction and has attracted an unfailing interest for decades. More recently, kernel PCA (KPCA) has emerged as an extension of PCA but, despite its use in practice, a sound theoretical understanding of KPCA is missing. We contribute several lower and upper bounds on the efficiency of KPCA, involving the empirical eigenvalues of the kernel Gram matrix and new quantities involving a notion of variance. These bounds show how much information is captured by KPCA on average and contribute a better theoretical understanding of its efficiency. We demonstrate that fast convergence rates are achievable for a widely used class of kernels and we highlight the importance of some desirable properties of datasets to ensure KPCA efficiency.
更多查看译文
关键词
kernel pca,lower bounds,performance
AI 理解论文
溯源树
样例
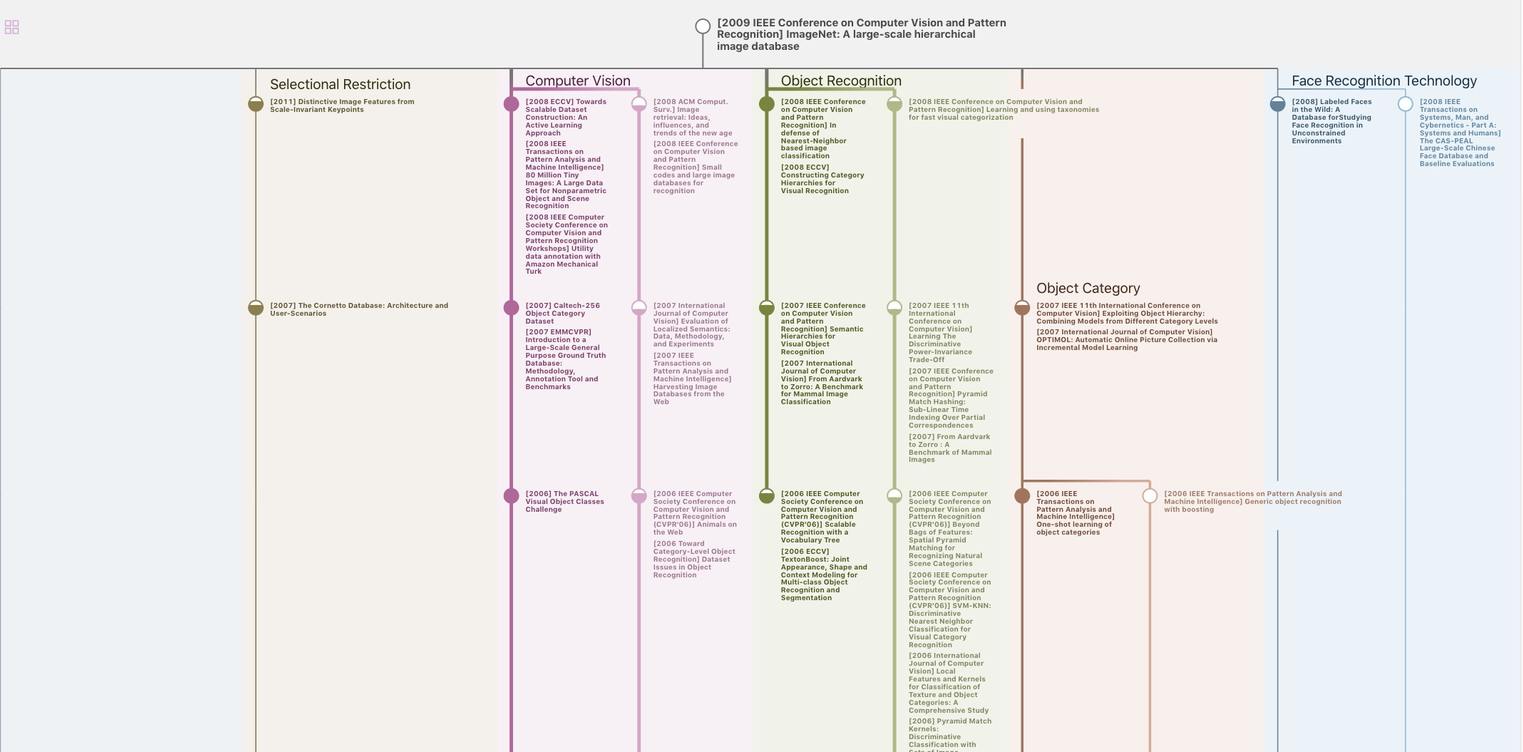
生成溯源树,研究论文发展脉络
Chat Paper
正在生成论文摘要