Pedestrian behavior prediction model with a convolutional LSTM encoder-decoder
PHYSICA A-STATISTICAL MECHANICS AND ITS APPLICATIONS(2020)
摘要
Pedestrian behavior modeling is a challenging problem especially in crowded transportation scenarios. Some recent studies have addressed this problem using deep neural network, but the accuracy of trajectory prediction is still not high because the internal structure of the typical deep neural network with long short-term memory (LSTM) is a one-dimensional vector, which destroys the spatial information around a pedestrian. Therefore, these models cannot fully learn spatial sensing behavior of pedestrians. To solve this, we recommend using multi-channel tensors to represent the environmental information of pedestrians. Meanwhile, the spatiotemporal interactions among the pedestrians are represented by convolution operations of these tensors. Then, an end-to-end fully convolutional LSTM encoder-decoder is designed, trained and tested. Finally, our approach is compared with existing LSTM-based methods using five crowded video sequences with public datasets. The results show that our method reduces the displacement offset error and provides more realistic trajectory prediction in manifold cases. (c) 2020 Published by Elsevier B.V.
更多查看译文
关键词
Pedestrian behavior model,Trajectory prediction,Long short-term memory,Convolution,Encoder-decoder
AI 理解论文
溯源树
样例
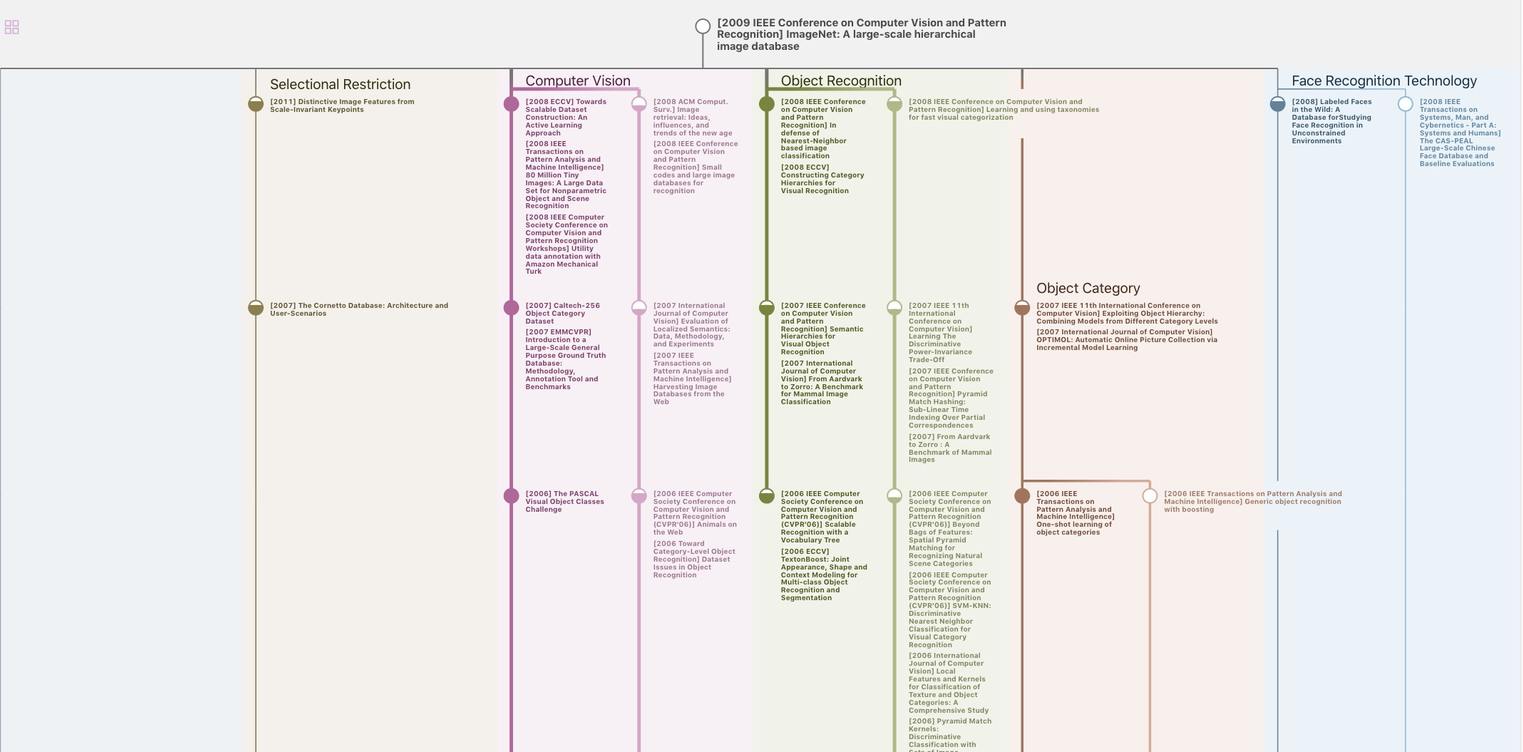
生成溯源树,研究论文发展脉络
Chat Paper
正在生成论文摘要