$\mathbb{X}$Resolution Correspondence Networks
arXiv (Cornell University)(2020)
摘要
In this paper, we aim at establishing accurate dense correspondences between a pair of images with overlapping field of view under challenging illumination variation, viewpoint changes, and style differences. Through an extensive ablation study of the state-of-the-art correspondence networks, we surprisingly discovered that the widely adopted 4D correlation tensor and its related learning and processing modules could be de-parameterised and removed from training with merely a minor impact over the final matching accuracy. Disabling some of the most memory consuming and computational expensive modules dramatically speeds up the training procedure and allows to use 4x bigger batch size, which in turn compensates for the accuracy drop. Together with a multi-GPU inference stage, our method facilitates the systematic investigation of the relationship between matching accuracy and up-sampling resolution of the native testing images from 720p to 4K. This leads to finding an optimal resolution $\mathbb X$ that produces accurate matching performance surpassing the state-of-the-art methods particularly over the lower error band for the proposed network and evaluation datasets.
更多查看译文
关键词
$\mathbb{x}$resolution,networks
AI 理解论文
溯源树
样例
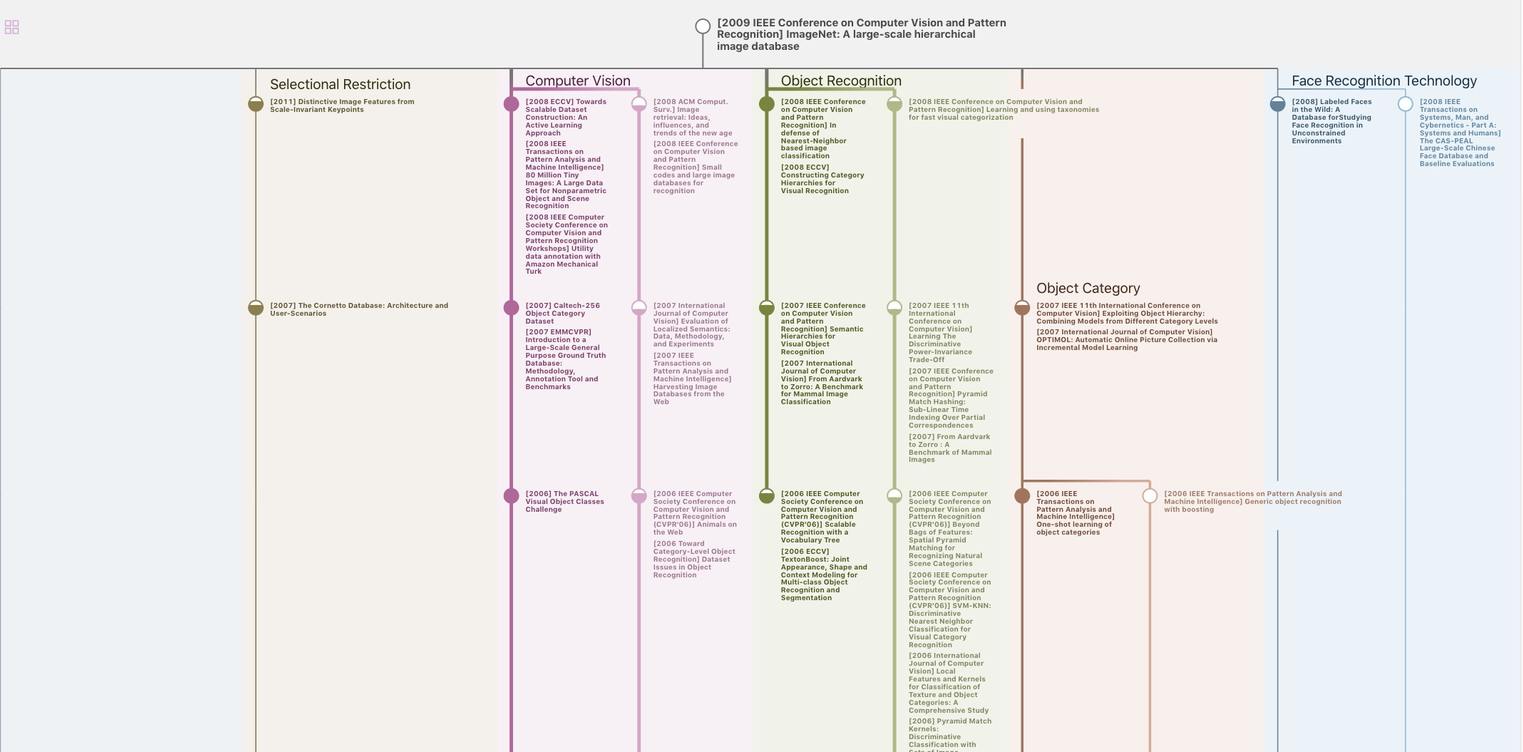
生成溯源树,研究论文发展脉络
Chat Paper
正在生成论文摘要