Toward Scalable and Privacy-preserving Deep Neural Network via Algorithmic-Cryptographic Co-design
ACM Transactions on Intelligent Systems and Technology(2022)
摘要
AbstractDeep Neural Networks (DNNs) have achieved remarkable progress in various real-world applications, especially when abundant training data are provided. However, data isolation has become a serious problem currently. Existing works build privacy-preserving DNN models from either algorithmic perspective or cryptographic perspective. The former mainly splits the DNN computation graph between data holders or between data holders and server, which demonstrates good scalability but suffers from accuracy loss and potential privacy risks. In contrast, the latter leverages time-consuming cryptographic techniques, which has strong privacy guarantee but poor scalability. In this article, we propose SPNN—a Scalable and Privacy-preserving deep Neural Network learning framework, from an algorithmic-cryptographic co-perspective. From algorithmic perspective, we split the computation graph of DNN models into two parts, i.e., the private-data-related computations that are performed by data holders and the rest heavy computations that are delegated to a semi-honest server with high computation ability. From cryptographic perspective, we propose using two types of cryptographic techniques, i.e., secret sharing and homomorphic encryption, for the isolated data holders to conduct private-data-related computations privately and cooperatively. Furthermore, we implement SPNN in a decentralized setting and introduce user-friendly APIs. Experimental results conducted on real-world datasets demonstrate the superiority of our proposed SPNN.
更多查看译文
关键词
Privacy-preserving, secret sharing, homomorphic encryption, deep neural network
AI 理解论文
溯源树
样例
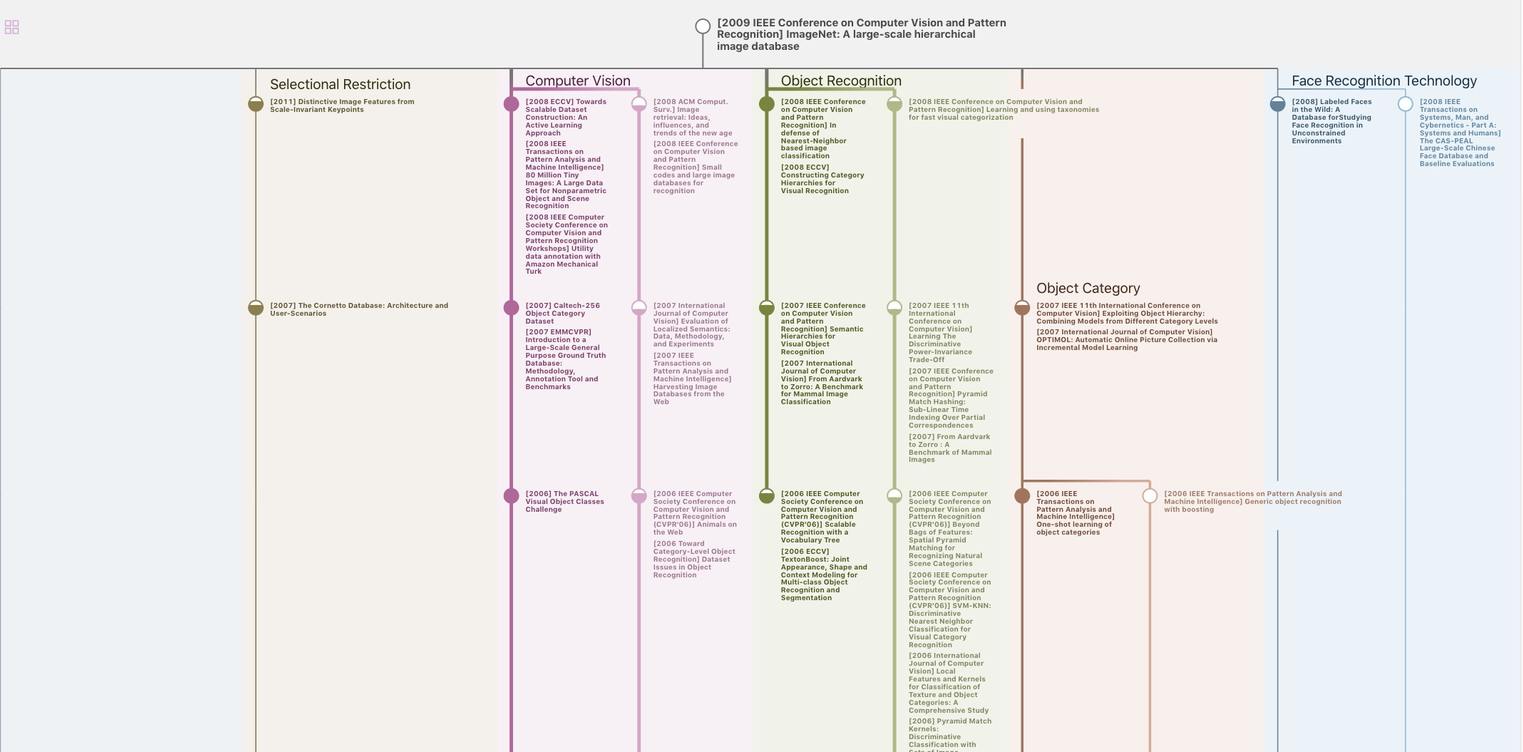
生成溯源树,研究论文发展脉络
Chat Paper
正在生成论文摘要