TrojanZoo: Towards Unified, Holistic, and Practical Evaluation of Neural Backdoors
2022 IEEE 7TH EUROPEAN SYMPOSIUM ON SECURITY AND PRIVACY (EUROS&P 2022)(2022)
摘要
Neural backdoors represent one primary threat to the security of deep learning systems. The intensive research has produced a plethora of backdoor attacks/defenses, resulting in a constant arms race. However, due to the lack of evaluation benchmarks, many critical questions remain under-explored: (i) what are the strengths and limitations of different attacks/defenses? (ii) what are the best practices to operate them? and (iii) how can the existing attacks/defenses be further improved? To bridge this gap, we design and implement TROJANZOO, the first open-source platform for evaluating neural backdoor attacks/defenses in a unified, holistic, and practical manner. Thus far, focusing on the computer vision domain, it has incorporated 8 representative attacks, 14 state-of-the-art defenses, 6 attack performance metrics, 10 defense utility metrics, as well as rich tools for in-depth analysis of the attack-defense interactions. Leveraging TROJANZOO, we conduct a systematic study on the existing attacks/defenses, unveiling their complex design spectrum: both manifest intricate trade-offs among multiple desiderata (e.g., the effectiveness, evasiveness, and transferability of attacks). We further explore improving the existing attacks/defenses, leading to a number of interesting findings: (i) one-pixel triggers often suffice; (ii) training from scratch often outperforms perturbing benign models to craft trojan models; (iii) optimizing triggers and trojan models jointly greatly improves both attack effectiveness and evasiveness; (iv) individual defenses can often be evaded by adaptive attacks; and (v) exploiting model interpretability significantly improves defense robustness. We envision that TROJANZOO will serve as a valuable platform to facilitate future research on neural backdoors.
更多查看译文
关键词
backdoor attack, backdoor defense, benchmark platform, deep learning security
AI 理解论文
溯源树
样例
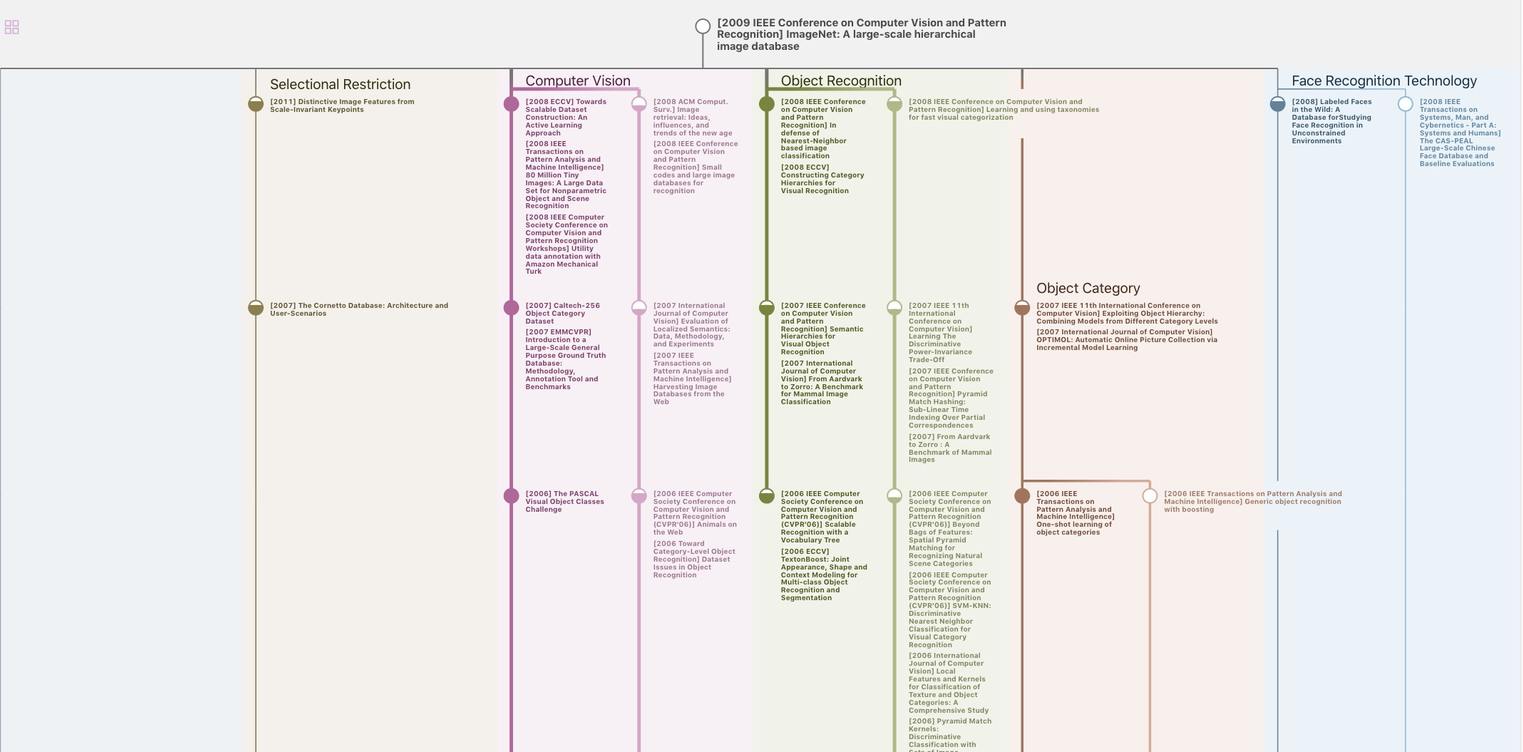
生成溯源树,研究论文发展脉络
Chat Paper
正在生成论文摘要