Split-Attention U-Net: A Fully Convolutional Network For Robust Multi-Label Segmentation From Brain Mri
BRAIN SCIENCES(2020)
摘要
Multi-label brain segmentation from brain magnetic resonance imaging (MRI) provides valuable structural information for most neurological analyses. Due to the complexity of the brain segmentation algorithm, it could delay the delivery of neuroimaging findings. Therefore, we introduce Split-Attention U-Net (SAU-Net), a convolutional neural network with skip pathways and a split-attention module that segments brain MRI scans. The proposed architecture employs split-attention blocks, skip pathways with pyramid levels, and evolving normalization layers. For efficient training, we performed pre-training and fine-tuning with the original and manually modified FreeSurfer labels, respectively. This learning strategy enables involvement of heterogeneous neuroimaging data in the training without the need for many manual annotations. Using nine evaluation datasets, we demonstrated that SAU-Net achieved better segmentation accuracy with better reliability that surpasses those of state-of-the-art methods. We believe that SAU-Net has excellent potential due to its robustness to neuroanatomical variability that would enable almost instantaneous access to accurate neuroimaging biomarkers and its swift processing runtime compared to other methods investigated.
更多查看译文
关键词
multi-label brain segmentation, split-attention block, deep learning, fine-tuning, SAU-Net
AI 理解论文
溯源树
样例
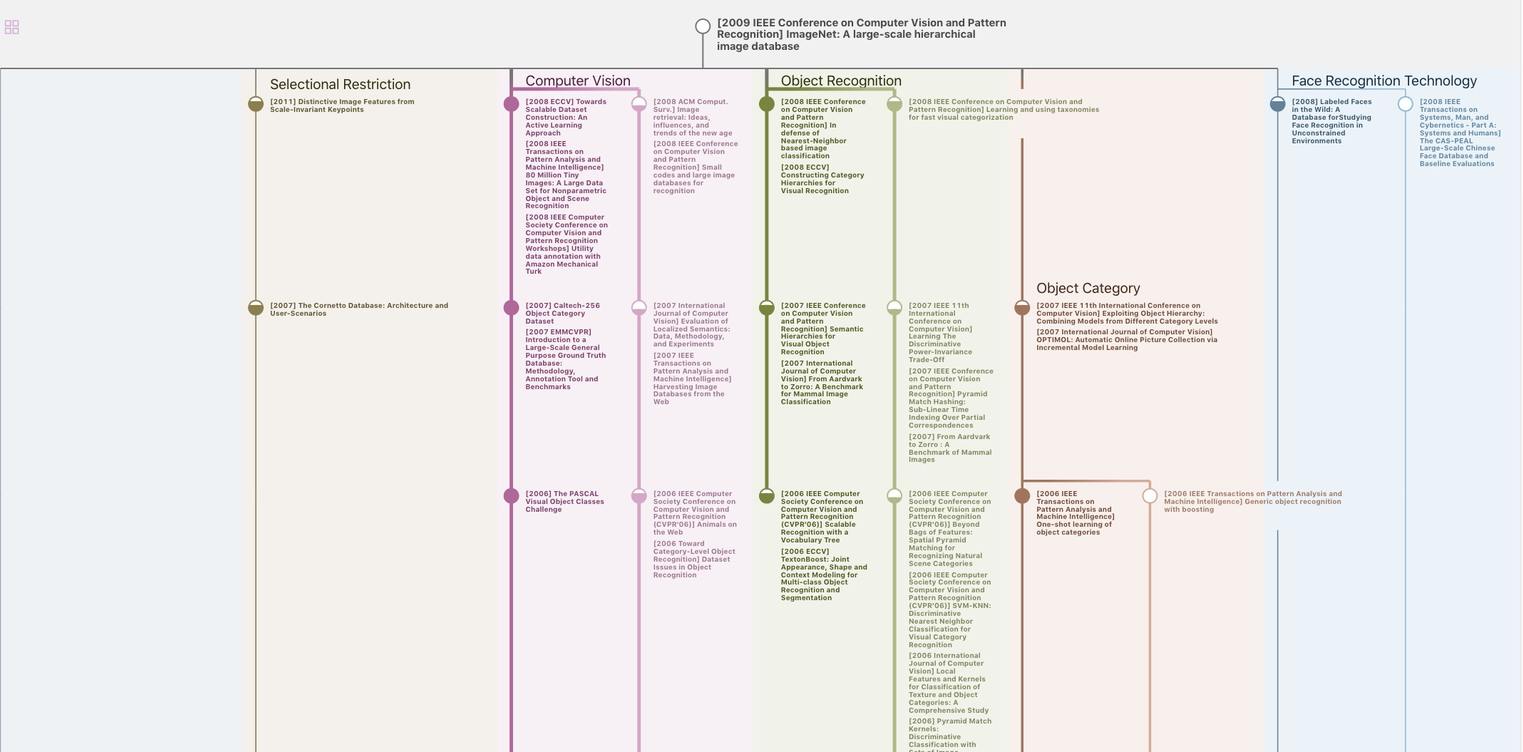
生成溯源树,研究论文发展脉络
Chat Paper
正在生成论文摘要