Numerical approximations of coupled forward-backward SPDEs
arxiv(2023)
摘要
We propose and study a scheme combining the finite element method and machine learning techniques for the numerical approximations of coupled nonlinear forward-backward stochastic partial differential equations (FBSPDEs) with homogeneous Dirichlet boundary conditions. Precisely, we generalize the pioneering work of Dunst and Prohl [SIAM J. Sci. Comp., 38(2017), 2725-2755] by considering general nonlinear and nonlocal FBSPDEs with more inclusive coupling; self-contained proofs are provided and different numerical techniques for the resulting finite dimensional equations are adopted. For such FBSPDEs allowing for non-Lipschitz nonlinearity on unknown variables, we prove the existence and uniqueness of the strong solution as well as of the weak solution. Then, the finite element method in the spatial domain leads to approximations of FBSPDEs by finite-dimensional forward-backward stochastic differential equations which are numerically computed by using some deep learning-based schemes. A strong convergence analysis is addressed for the spatial discretization of coupled FBSPDEs under an additional Lipschitz assumption, and the numerical examples, including both decoupled and coupled cases, indicate that our methods are quite efficient.
更多查看译文
关键词
Stochastic partial differential equation,numerical analysis,weak and strong solution,deep learning,non-Markovianity
AI 理解论文
溯源树
样例
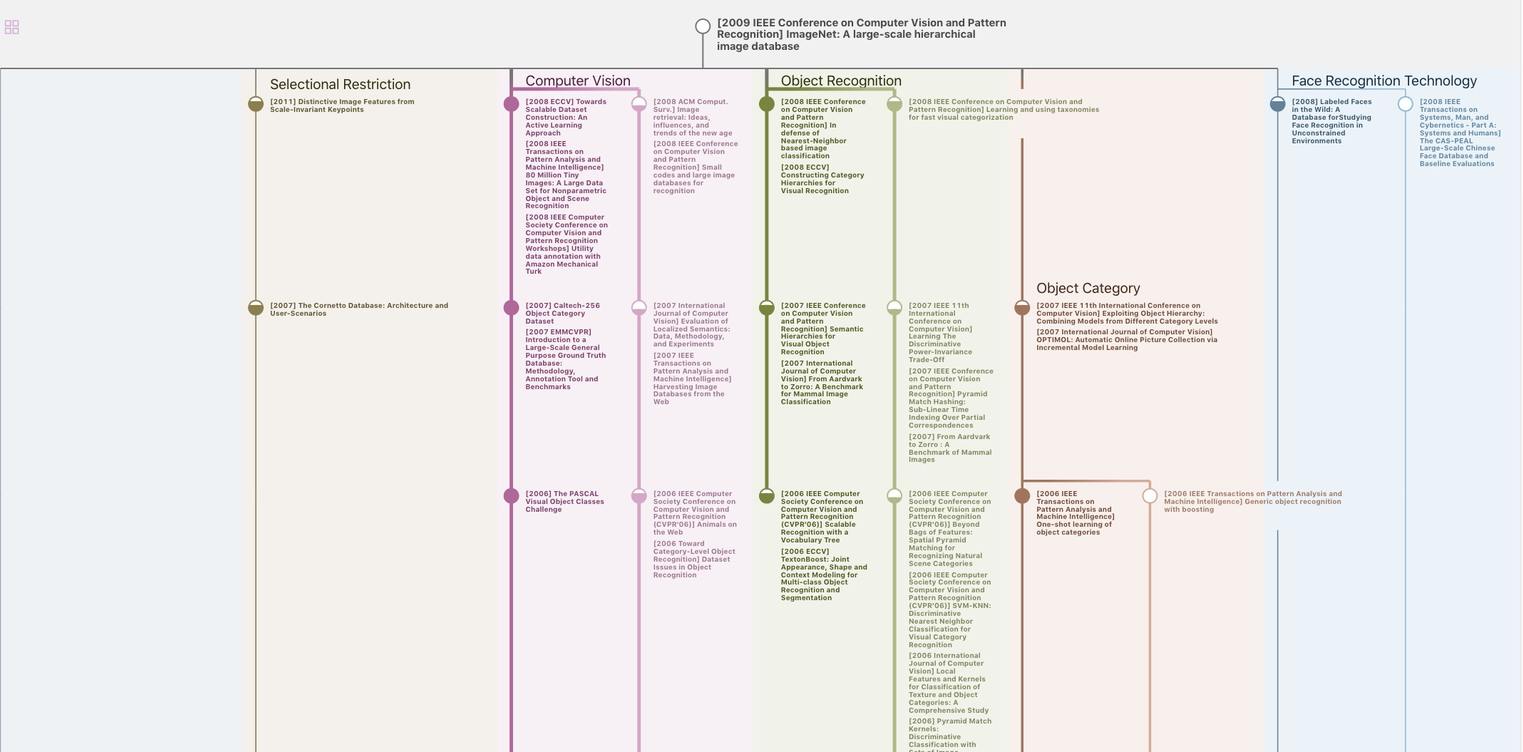
生成溯源树,研究论文发展脉络
Chat Paper
正在生成论文摘要