Towards localisation of keywords in speech using weak supervision
arxiv(2020)
摘要
Developments in weakly supervised and self-supervised models could enable speech technology in low-resource settings where full transcriptions are not available. We consider whether keyword localisation is possible using two forms of weak supervision where location information is not provided explicitly. In the first, only the presence or absence of a word is indicated, i.e. a bag-of-words (BoW) labelling. In the second, visual context is provided in the form of an image paired with an unlabelled utterance; a model then needs to be trained in a self-supervised fashion using the paired data. For keyword localisation, we adapt a saliency-based method typically used in the vision domain. We compare this to an existing technique that performs localisation as a part of the network architecture. While the saliency-based method is more flexible (it can be applied without architectural restrictions), we identify a critical limitation when using it for keyword localisation. Of the two forms of supervision, the visually trained model performs worse than the BoW-trained model. We show qualitatively that the visually trained model sometimes locate semantically related words, but this is not consistent. While our results show that there is some signal allowing for localisation, it also calls for other localisation methods better matched to these forms of weak supervision.
更多查看译文
关键词
weak supervision,keywords,speech,localisation
AI 理解论文
溯源树
样例
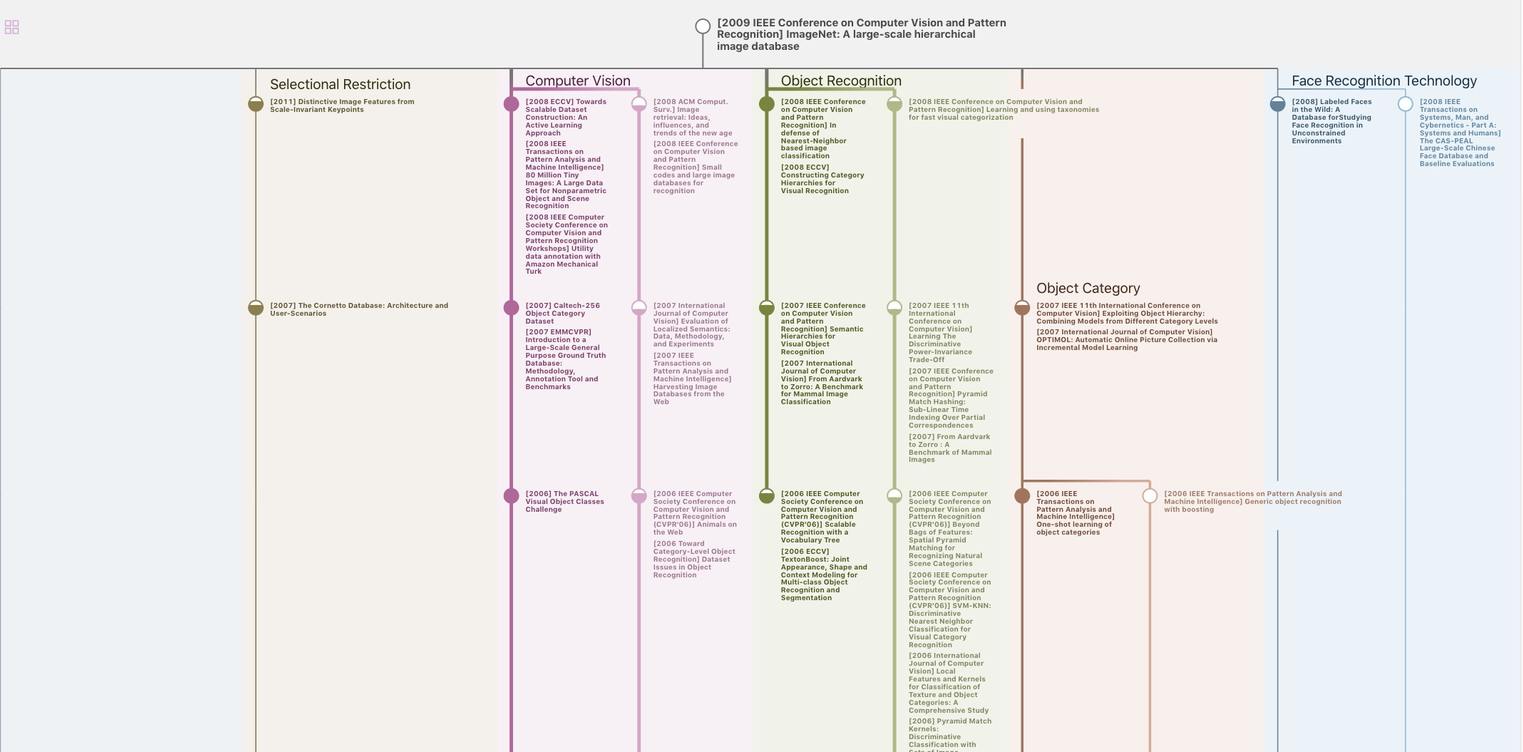
生成溯源树,研究论文发展脉络
Chat Paper
正在生成论文摘要