Mask-Align: Self-Supervised Neural Word Alignment.
59TH ANNUAL MEETING OF THE ASSOCIATION FOR COMPUTATIONAL LINGUISTICS AND THE 11TH INTERNATIONAL JOINT CONFERENCE ON NATURAL LANGUAGE PROCESSING (ACL-IJCNLP 2021), VOL 1(2021)
摘要
Word alignment, which aims to align translationally equivalent words between source and target sentences, plays an important role in many natural language processing tasks. Current unsupervised neural alignment methods focus on inducing alignments from neural machine translation models, which does not leverage the full context in the target sequence. In this paper, we propose MASK-ALIGN, a self-supervised word alignment model that takes advantage of the full context on the target side. Our model parallelly masks out each target token and predicts it conditioned on both source and the remaining target tokens. This two-step process is based on the assumption that the source token contributing most to recovering the masked target token should be aligned. We also introduce an attention variant called leaky attention, which alleviates the problem of high cross-attention weights on specific tokens such as periods. Experiments on four language pairs show that our model outperforms previous unsupervised neural aligners and obtains new state-of-the-art results.
更多查看译文
关键词
word,mask-align,self-supervised
AI 理解论文
溯源树
样例
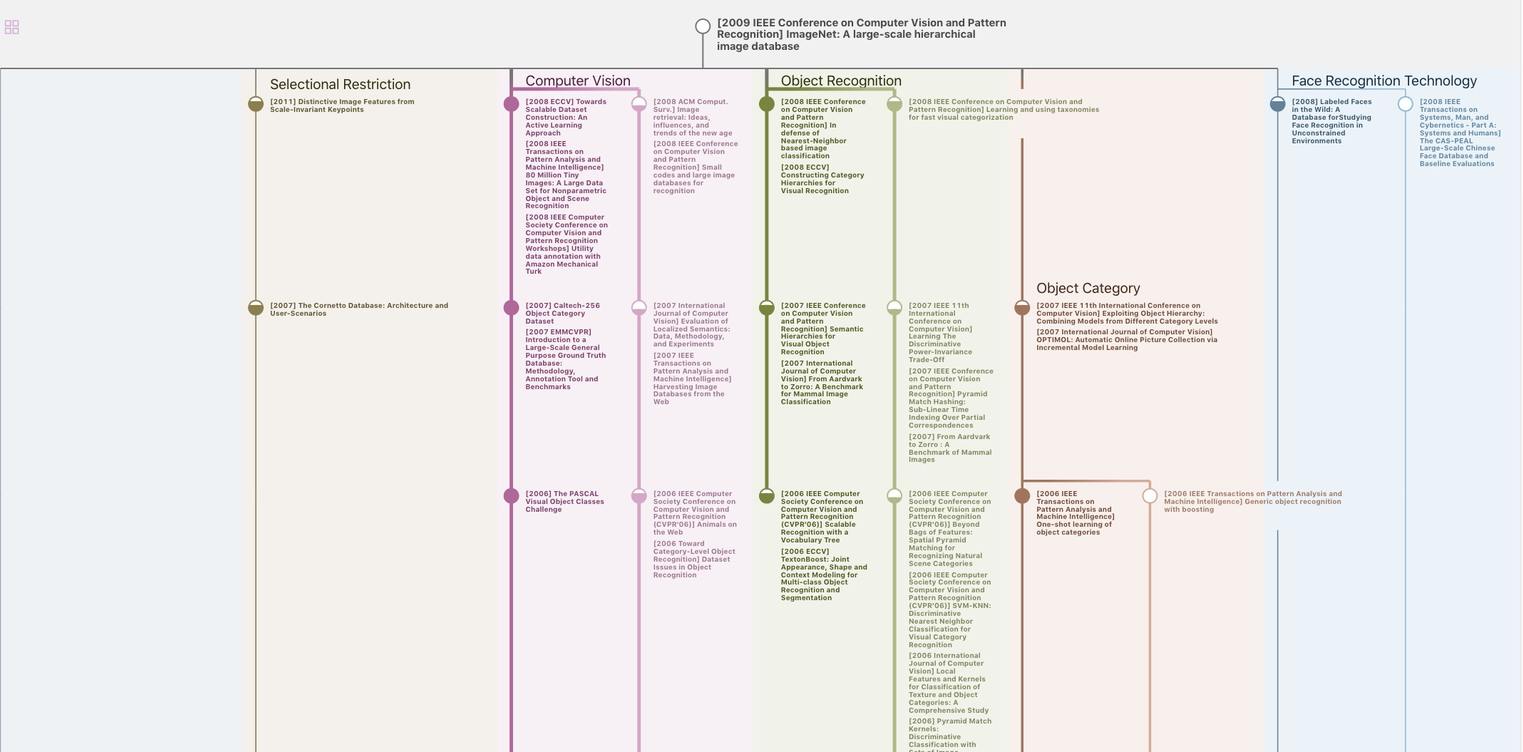
生成溯源树,研究论文发展脉络
Chat Paper
正在生成论文摘要