Frontal electroencephalogram based drug, sex, and age independent sedation level prediction using non-linear machine learning algorithms
Journal of Clinical Monitoring and Computing(2020)
摘要
Brain monitors which track quantitative electroencephalogram (EEG) signatures to monitor sedation levels are drug and patient specific. There is a need for robust sedation level monitoring systems to accurately track sedation levels across all drug classes, sex and age groups. Forty-four quantitative features estimated from a pooled dataset of 204 EEG recordings from 66 healthy adult volunteers who received either propofol, dexmedetomidine, or sevoflurane (all with and without remifentanil) were used in a machine learning based automated system to estimate the depth of sedation. Model training and evaluation were performed using leave-one-out cross validation methodology. We trained four machine learning models to predict sedation levels and evaluated the influence of remifentanil, age, and sex on the prediction performance. The area under the receiver-operator characteristic curve (AUC) was used to assess the performance of the prediction model. The ensemble tree with bagging outperformed other machine learning models and predicted sedation levels with an AUC = 0.88 (0.81–0.90). There were significant differences in the prediction probability of the automated systems when trained and tested across different age groups and sex. The performance of the EEG based sedation level prediction system is drug, sex, and age specific. Nonlinear machine-learning models using quantitative EEG features can accurately predict sedation levels. The results obtained in this study may provide a useful reference for developing next generation EEG based sedation level prediction systems using advanced machine learning algorithms. Clinical trial registration: NCT 02043938 and NCT 03143972.
更多查看译文
关键词
Anaesthesia,Electroencephalogram,Medical informatics,Consciousness Monitors,Machine learning
AI 理解论文
溯源树
样例
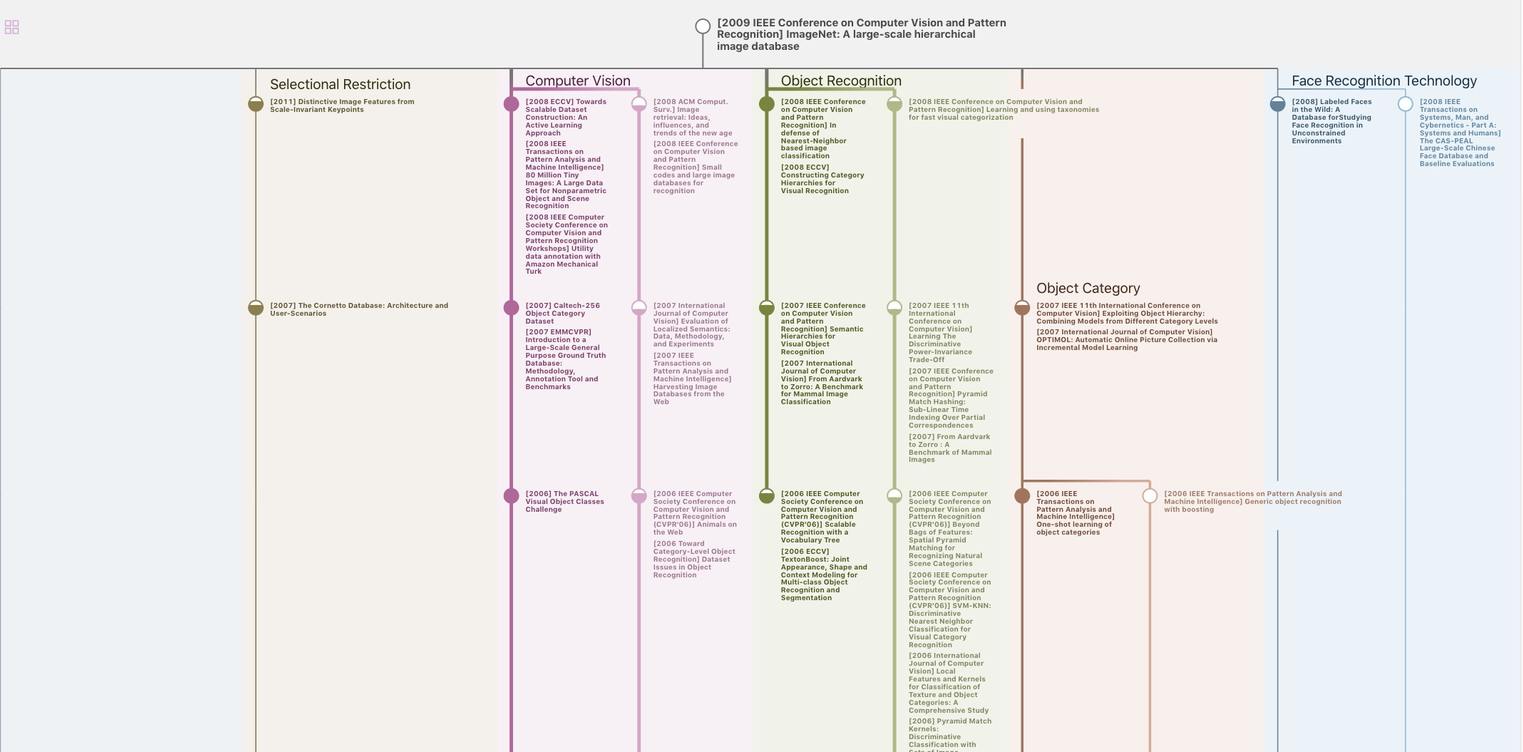
生成溯源树,研究论文发展脉络
Chat Paper
正在生成论文摘要