Do we need different machine learning algorithms for QSAR modeling? A comprehensive assessment of 16 machine learning algorithms on 14 QSAR data sets
BRIEFINGS IN BIOINFORMATICS(2021)
摘要
Although a wide variety of machine learning (ML) algorithms have been utilized to learn quantitative structure-activity relationships (QSARs), there is no agreed single best algorithm for QSAR learning. Therefore, a comprehensive understanding of the performance characteristics of popular ML algorithms used in QSAR learning is highly desirable. In this study, five linear algorithms [linear function Gaussian process regression (linear-GPR), linear function support vector machine (linear-SVM), partial least squares regression (PLSR), multiple linear regression (MLR) and principal component regression (PCR)], three analogizers [radial basis function support vector machine (rbf-SVM), K-nearest neighbor (KNN) and radial basis function Gaussian process regression (rbf-GPR)], six symbolists [extreme gradient boosting (XGBoost), Cubist, random forest (RF), multiple adaptive regression splines (MARS), gradient boosting machine (GBM), and classification and regression tree (CART)] and two connectionists [principal component analysis artificial neural network (pca-ANN) and deep neural network (DNN)] were employed to learn the regression-based QSAR models for 14 public data sets comprising nine physicochemical properties and five toxicity endpoints. The results show that rbf-SVM, rbf-GPR, XGBoost and DNN generally illustrate better performances than the other algorithms. The overall performances of different algorithms can be ranked from the best to the worst as follows: rbf-SVM>XGBoost > rbf-GPR>Cubist > GBM>DNN>RF>pca-ANN>MARS > linear-GPR approximate to KNN>linear-SVM approximate to PLSR > CART approximate to PCR approximate to MLR. In terms of prediction accuracy and computational efficiency, SVM and XGBoost are recommended to the regression learning for small data sets, and XGBoost is an excellent choice for large data sets. We then investigated the performances of the ensemble models by integrating the predictions of multiple ML algorithms. The results illustrate that the ensembles of two or three algorithms in different categories can indeed improve the predictions of the best individual ML algorithms. [GRAPHICS]
更多查看译文
关键词
QSAR, machine learning, XGBoost, support vector machine, ensemble learning
AI 理解论文
溯源树
样例
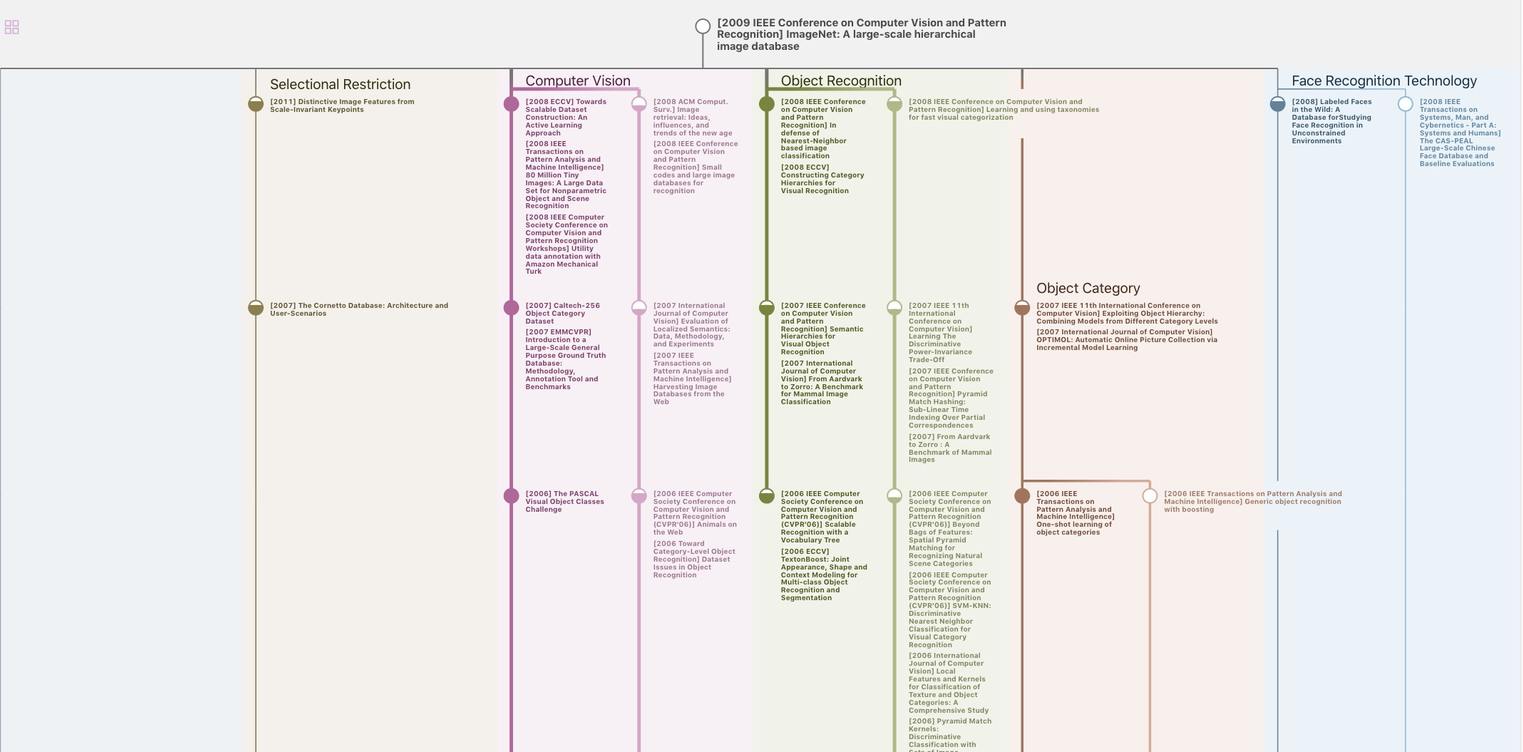
生成溯源树,研究论文发展脉络
Chat Paper
正在生成论文摘要