PoP-Net: Pose over Parts Network for Multi-Person 3D Pose Estimation from a Depth Image
2022 IEEE WINTER CONFERENCE ON APPLICATIONS OF COMPUTER VISION (WACV 2022)(2022)
摘要
In this paper, a real-time method called PoP-Net is proposed to predict multi-person 3D poses from a depth image. PoP-Net learns to predict bottom-up part representations and top-down global poses in a single shot. Specifically, a new part-level representation, called Truncated Part Displacement Field (TPDF), is introduced which enables an explicit fusion process to unify the advantages of bottom-up part detection and global pose detection. Meanwhile, an effective mode selection scheme is introduced to automatically resolve the conflicting cases between global pose and part detections. Finally, due to the lack of high-quality depth datasets for developing multi-person 3D pose estimation, we introduce Multi-Person 3D Human Pose Dataset (MP-3DHP) as a new benchmark. MP-3DHP is designed to enable effective multi-person and background data augmentation in model training, and to evaluate 3D human pose estimators under uncontrolled multi-person scenarios. We show that PoP-Net achieves the state-of-the-art results both on MP-3DHP and on the widely used ITOP dataset, and has significant advantages in efficiency for multi-person processing. MP-3DHP Dataset and the evaluation code have been made available at: https://github.com/oppo-us-research/PoP-Net.
更多查看译文
关键词
pose,parts network,depth,pop-net,multi-person
AI 理解论文
溯源树
样例
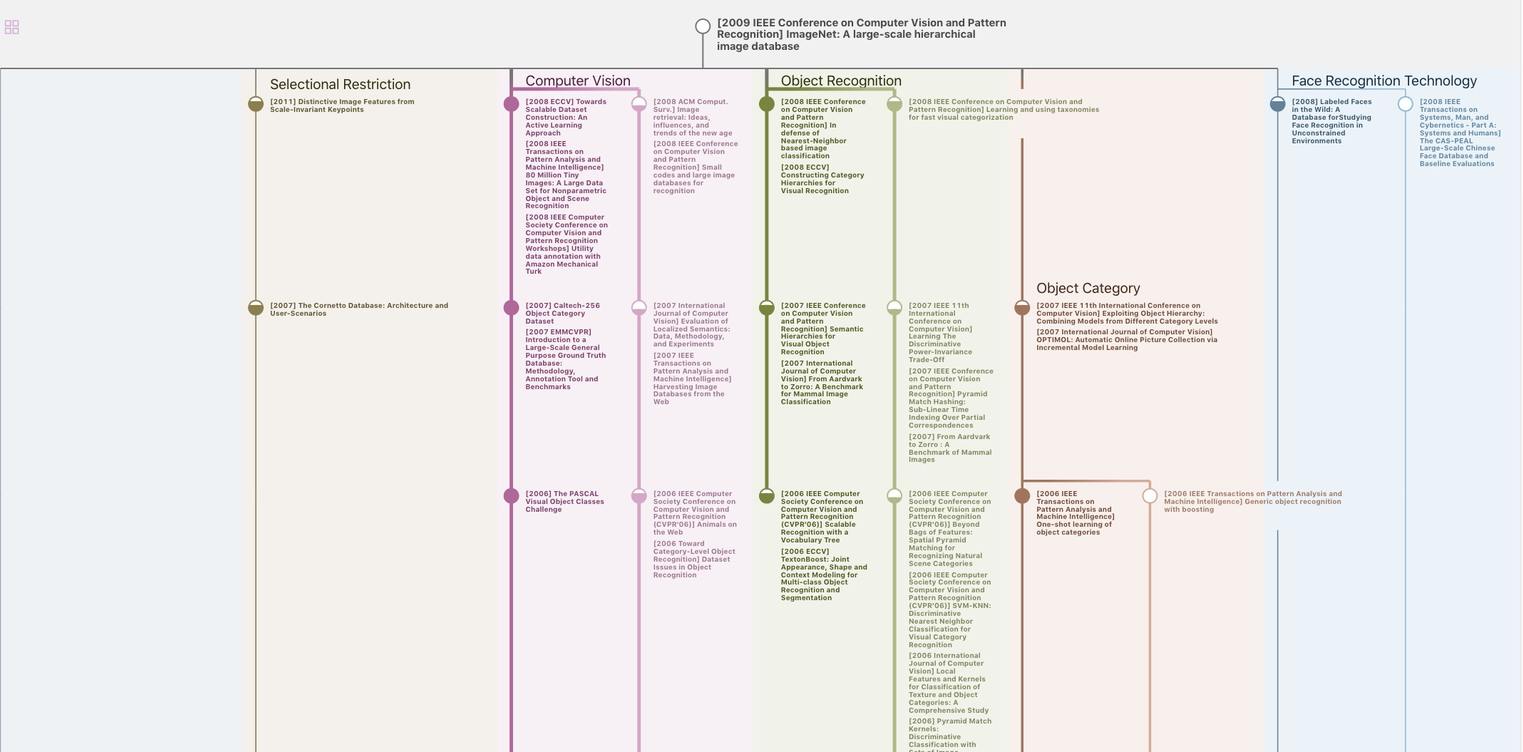
生成溯源树,研究论文发展脉络
Chat Paper
正在生成论文摘要