Probabilistic Conditional System Invariant Generation with Bayesian Inference
arxiv(2020)
摘要
Invariants are a set of properties over program attributes that are expected to be true during the execution of a program. Since developing those invariants manually can be costly and challenging, there are a myriad of approaches that support automated mining of likely invariants from sources such as program traces. Existing approaches, however, are not equipped to capture the rich states that condition the behavior of autonomous mobile robots, or to manage the uncertainty associated with many variables in these systems. This means that valuable invariants that appear only under specific states remain uncovered. In this work we introduce an approach to infer conditional probabilistic invariants to assist in the characterization of the behavior of such rich stateful, stochastic systems. These probabilistic invariants can encode a family of conditional patterns, are generated using Bayesian inference to leverage observed trace data against priors gleaned from previous experience and expert knowledge, and are ranked based on their surprise value and information content. Our studies on two semi-autonomous mobile robotic systems show how the proposed approach is able to generate valuable and previously hidden stateful invariants.
更多查看译文
关键词
bayesian
AI 理解论文
溯源树
样例
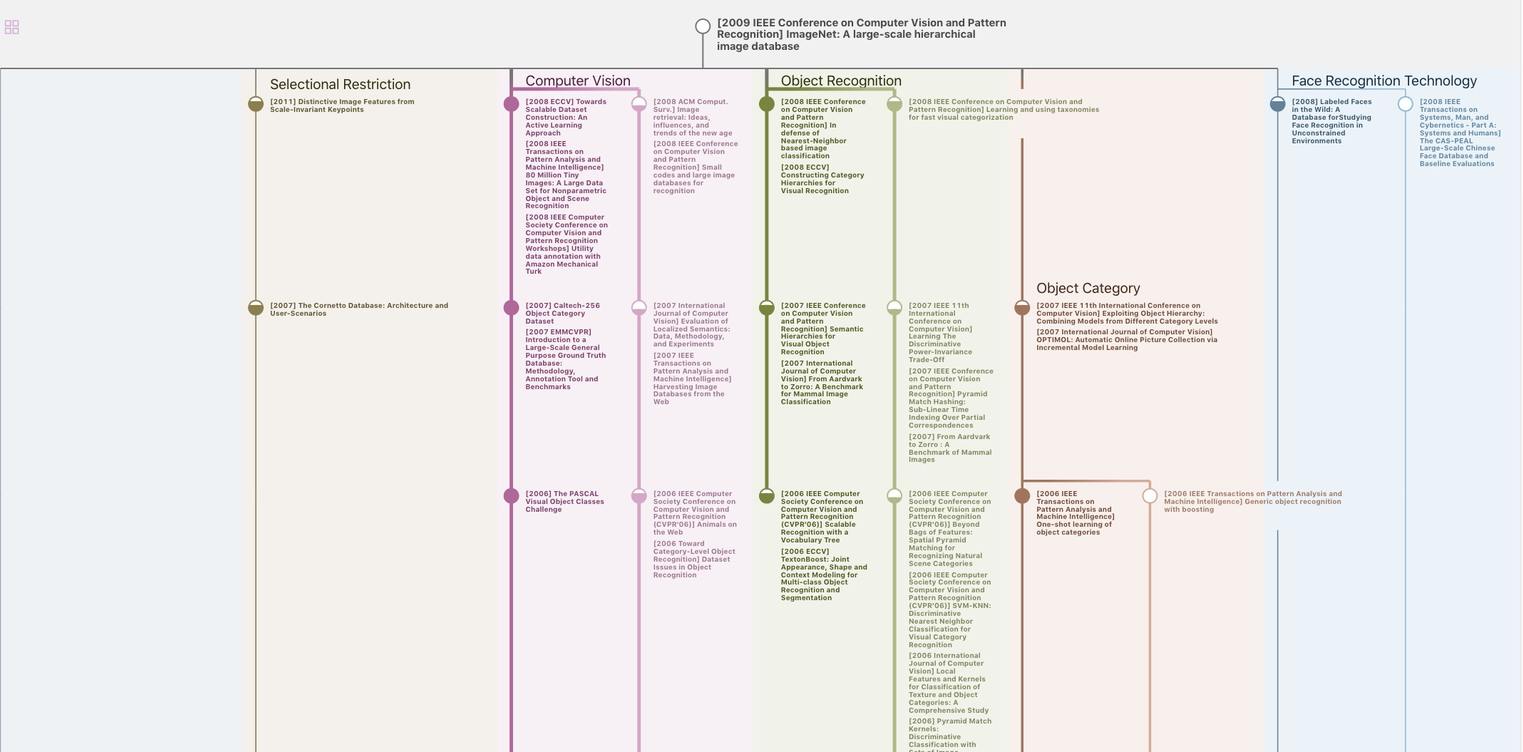
生成溯源树,研究论文发展脉络
Chat Paper
正在生成论文摘要