MEMS IMU and One-Way-Travel-Time Navigation for Autonomous Underwater Vehicles
OCEANS-IEEE(2017)
摘要
The absence of the global positioning system (GPS) is a challenge to navigation and localization in the underwater environment. Thus, for precise and accurate navigation, autonomous underwater vehicles (AUVs) often rely on very precise (yet very expensive and high energy consumption) inertial strap-down sensors which require external observations to constrain their dead-reckoned position error over time - e.g., Doppler velocity logs (DVLs) or acoustic positioning systems. Recent advances in acoustic navigation methodologies are enabling AUVs to extend submerged mission time by providing external position corrections and thereby constraining position error. Additionally, advances in microelectromechanical system (MEMS) inertial sensor technology has drastically lowered the size, power consumption, and cost of inertial sensors; however, they are still noisy and accumulate error over time. This paper builds on recent advances in single beacon one-way-travel-time (OWTT) acoustic navigation and investigates the degree of bounding position error for small AUVs with a minimal navigation strap-down sensor suite, relying on a consumer grade MEMS inertial measurement unit (IMU) and a vehicle's dynamic model velocity. A real time implementation of an Extended Kalman Filter (EKF), containing bias estimation, is obtained in the field with two Ocean-Server, Inc. Iver2 vehicles, and results show an average position error of 10.26 meters and 12.95 meters over a distance traveled of 1.73 kilometers and 1.91 kilometers, respectively.
更多查看译文
关键词
microelectromechanical system,IMU,inertial measurement unit,GPS,vehicle dynamic model velocity,extended Kalman filter,EKF,MEMS,underwater environment,localization,global positioning system,one-way-travel-time navigation,inertial sensors,acoustic navigation methodologies,acoustic positioning systems,inertial strap-down sensors,AUVs,autonomous underwater vehicles
AI 理解论文
溯源树
样例
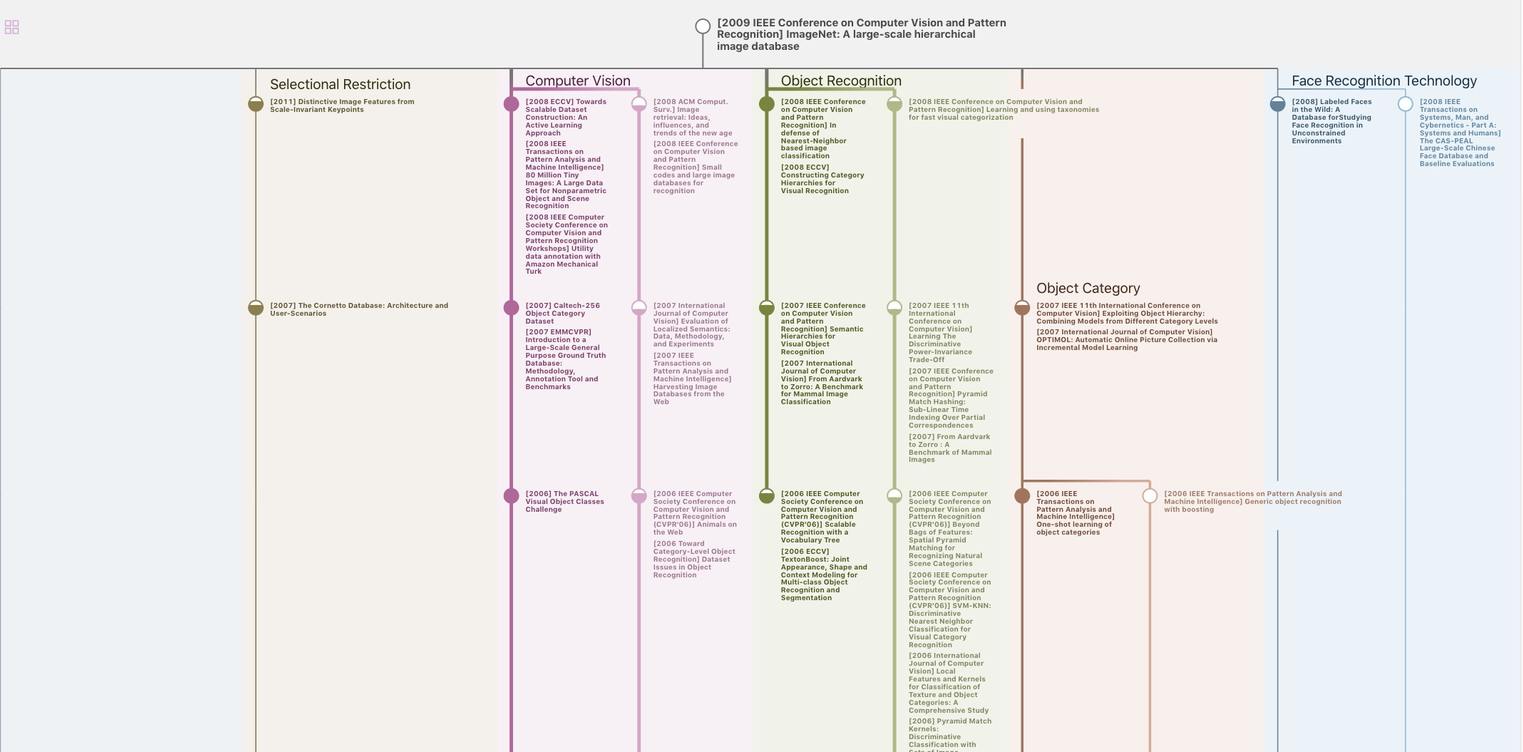
生成溯源树,研究论文发展脉络
Chat Paper
正在生成论文摘要