Dose Super-Resolution In Prostate Volumetric Modulated Arc Therapy Using Cascaded Deep Learning Networks
FRONTIERS IN ONCOLOGY(2020)
摘要
PurposeThis study proposes a cascaded network model for generating high-resolution doses (i.e., a 1 mm grid) from low-resolution doses (i.e., >= 3 mm grids) with reduced computation time.MethodsUsing the anisotropic analytical algorithm with three grid sizes (1, 3, and 5 mm) and the Acuros XB algorithm with two grid sizes (1 and 3 mm), dose distributions were calculated for volumetric modulated arc therapy plans for 73 prostate cancer patients. Our cascaded network model consisted of a hierarchically densely connected U-net (HD U-net) and a residual dense network (RDN), which were trained separately following a two-dimensional slice-by-slice procedure. The first network (HD U-net) predicted the downsampled high-resolution dose (generated through bicubic downsampling of the baseline high-resolution dose) using the low-resolution dose; subsequently, the second network (RDN) predicted the high-resolution dose from the output of the first network. Further, the predicted high-resolution dose was converted to its absolute value. We quantified the network performance using the spatial/dosimetric parameters (dice similarity coefficient, mean dose, maximum dose, minimum dose, homogeneity index, conformity index, and V-95%, V-70%, V-50%, and V-30%) for the low-resolution and predicted high-resolution doses relative to the baseline high-resolution dose. Gamma analysis (between the baseline dose and the low-resolution dose/predicted high-resolution dose) was performed with a 2%/2 mm criterion and 10% threshold.ResultsThe average computation time to predict a high-resolution axial dose plane was <0.02 s. The dice similarity coefficient values for the predicted doses were closer to 1 when compared to those for the low-resolution doses. Most of the dosimetric parameters for the predicted doses agreed more closely with those for the baseline than for the low-resolution doses. In most of the parameters, no significant differences (p-value of >0.05) between the baseline and predicted doses were observed. The gamma passing rates for the predicted high-resolution does were higher than those for the low-resolution doses.ConclusionThe proposed model accurately predicted high-resolution doses for the same dose calculation algorithm. Our model uses only dose data as the input without additional data, which provides advantages of convenience to user over other dose super-resolution methods.
更多查看译文
关键词
deep learning, cascaded networks, dose super-resolution, dose grid size, prostate volumetric modulated arc therapy
AI 理解论文
溯源树
样例
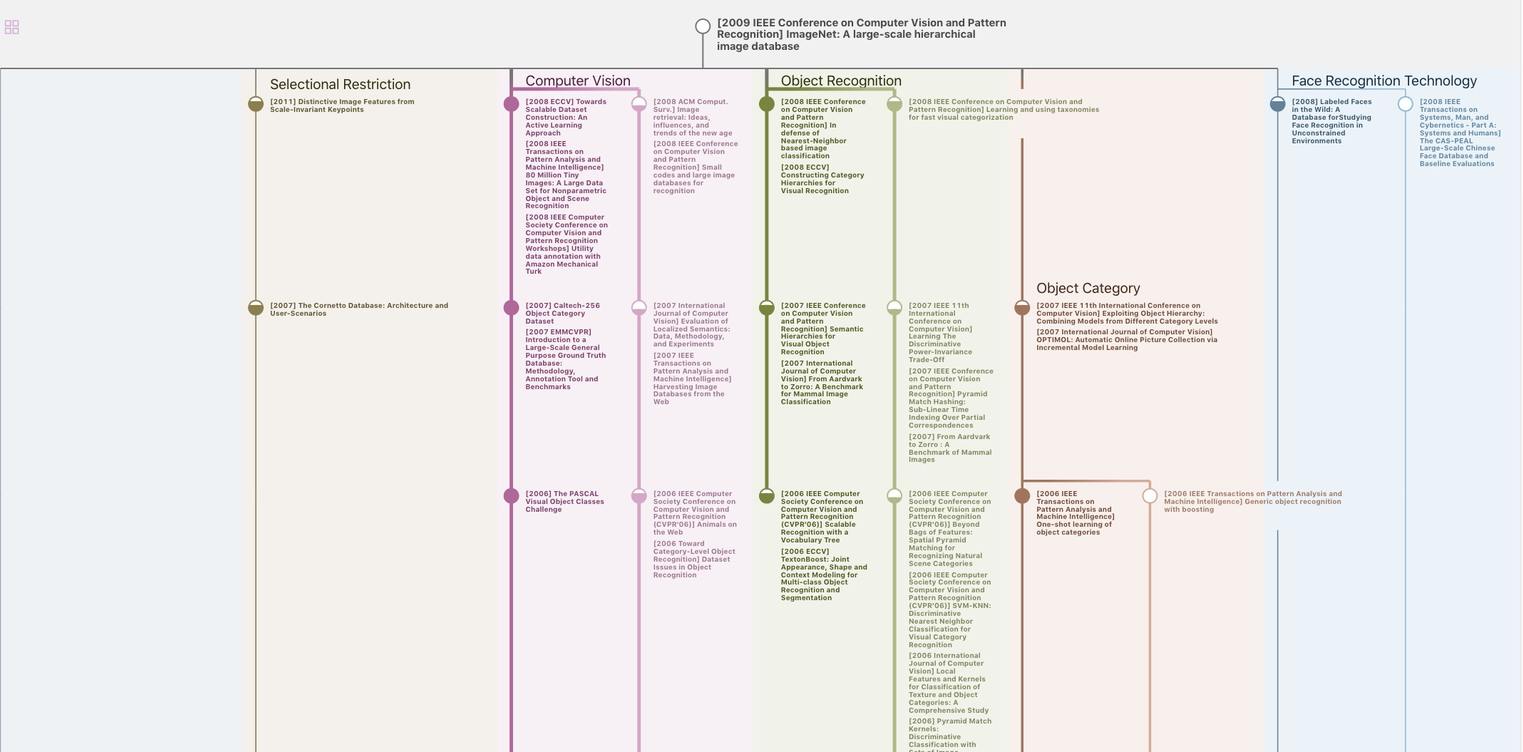
生成溯源树,研究论文发展脉络
Chat Paper
正在生成论文摘要