Machine learning to reveal hidden risk combinations for the trajectory of posttraumatic stress disorder symptoms
SCIENTIFIC REPORTS(2020)
摘要
The nature of the recovery process of posttraumatic stress disorder (PTSD) symptoms is multifactorial. The Massive Parallel Limitless-Arity Multiple-testing Procedure (MP-LAMP), which was developed to detect significant combinational risk factors comprehensively, was utilized to reveal hidden combinational risk factors to explain the long-term trajectory of the PTSD symptoms. In 624 population-based subjects severely affected by the Great East Japan Earthquake, 61 potential risk factors encompassing sociodemographics, lifestyle, and traumatic experiences were analyzed by MP-LAMP regarding combinational associations with the trajectory of PTSD symptoms, as evaluated by the Impact of Event Scale-Revised score after eight years adjusted by the baseline score. The comprehensive combinational analysis detected 56 significant combinational risk factors, including 15 independent variables, although the conventional bivariate analysis between single risk factors and the trajectory detected no significant risk factors. The strongest association was observed with the combination of short resting time, short walking time, unemployment, and evacuation without preparation (adjusted P value = 2.2 × 10 −4 , and raw P value = 3.1 × 10 −9 ). Although short resting time had no association with the poor trajectory, it had a significant interaction with short walking time ( P value = 1.2 × 10 −3 ), which was further strengthened by the other two components ( P value = 9.7 × 10 −5 ). Likewise, components that were not associated with a poor trajectory in bivariate analysis were included in every observed significant risk combination due to their interactions with other components. Comprehensive combination detection by MP-LAMP is essential for explaining multifactorial psychiatric symptoms by revealing the hidden combinations of risk factors.
更多查看译文
关键词
Computational biology and bioinformatics,Environmental social sciences,Health care,Mathematics and computing,Psychology,Risk factors,Signs and symptoms,Science,Humanities and Social Sciences,multidisciplinary
AI 理解论文
溯源树
样例
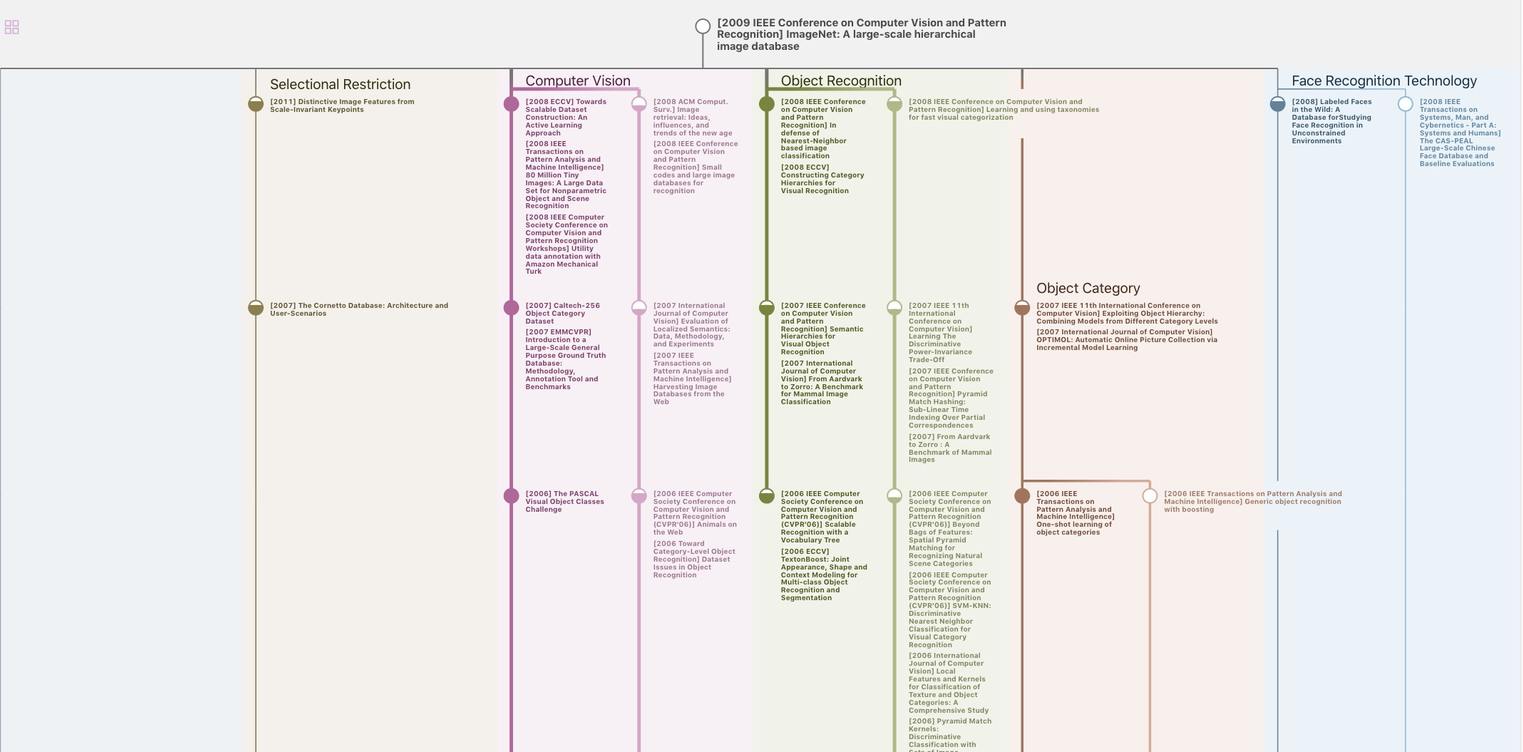
生成溯源树,研究论文发展脉络
Chat Paper
正在生成论文摘要