Bipartite Graph Embedding via Mutual Information Maximization
WSDM(2021)
摘要
ABSTRACTBipartite graph embedding has recently attracted much attention due to the fact that bipartite graphs are widely used in various application domains. Most previous methods, which adopt random walk-based or reconstruction-based objectives, are typically effective to learn local graph structures. However, the global properties of bipartite graph, including community structures of homogeneous nodes and long-range dependencies of heterogeneous nodes, are not well preserved. In this paper, we propose a bipartite graph embedding called BiGI to capture such global properties by introducing a novel local-global infomax objective. Specifically, BiGI first generates a global representation which is composed of two prototype representations. BiGI then encodes sampled edges as local representations via the proposed subgraph-level attention mechanism. Through maximizing the mutual information between local and global representations, BiGI enables nodes in bipartite graph to be globally relevant. Our model is evaluated on various benchmark datasets for the tasks of top-K recommendation and link prediction. Extensive experiments demonstrate that BiGI achieves consistent and significant improvements over state-of-the-art baselines. Detailed analyses verify the high effectiveness of modeling the global properties of bipartite graph.
更多查看译文
关键词
Bipartite Graph Embedding,Global Properties,Mutual Information Maximization,Recommender System
AI 理解论文
溯源树
样例
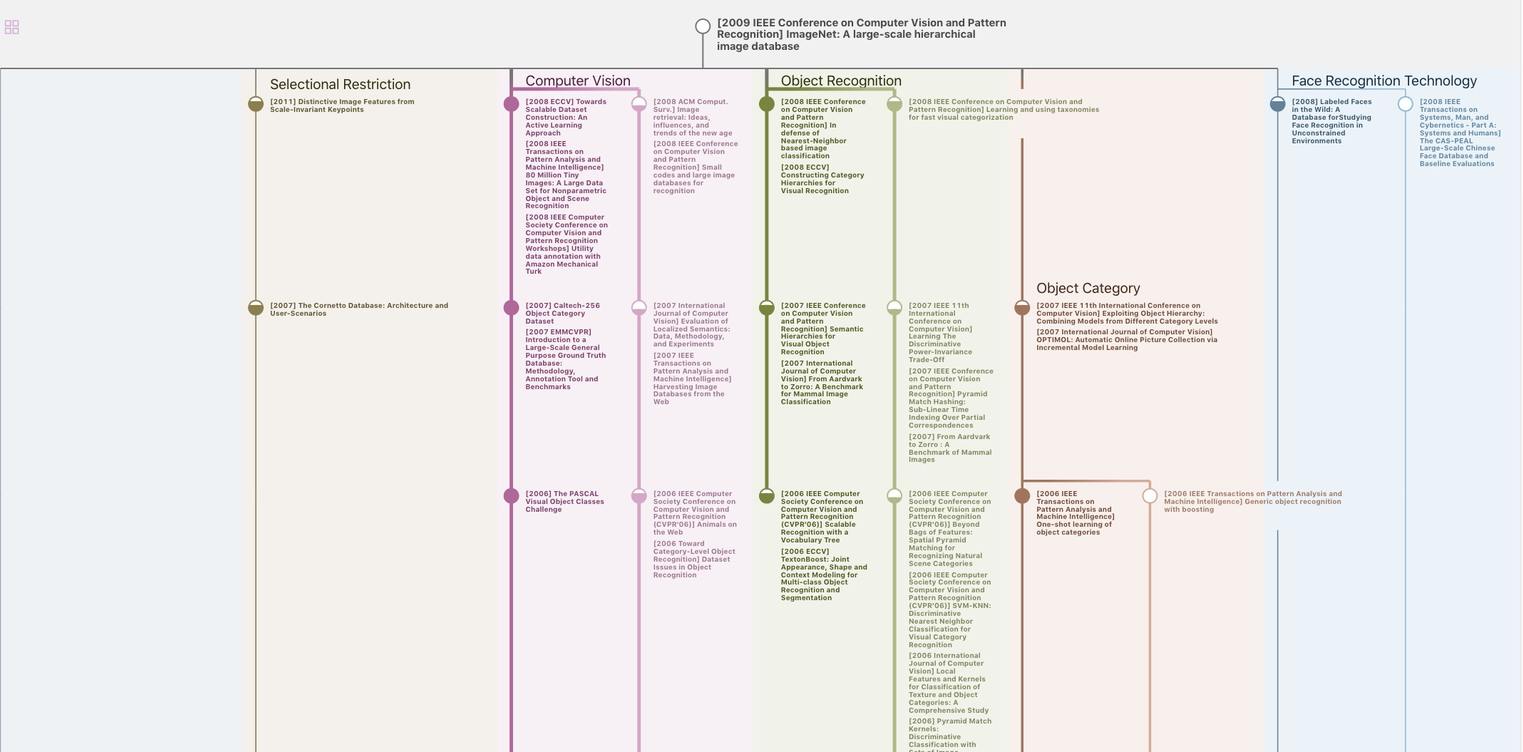
生成溯源树,研究论文发展脉络
Chat Paper
正在生成论文摘要