Exploiting Repeated Behavior Pattern and Long-term Item dependency for Session-based Recommendation
arxiv(2020)
摘要
Session-based recommendation (SBR) is a challenging task, which aims to predict users' future interests based on anonymous behavior sequences. Existing methods for SBR leverage powerful representation learning approaches to encode sessions into a low dimensional space. However, all the existing studies focus on the item transitions in the session, without modeling the behavior patterns, which are strong clues to capture the preference of users. Further, the long-term dependency within the session is neglected in most of the current methods. To this end, we propose a novel Repeat-aware Neural Mechanism for Session-based Recommendation (RNMSR). Specifically, we introduce repeated behavior pattern into SBR, which contains the potential intent information and critical item frequency signal. Furthermore, we also built a similarity-based session graph based on long-term dependencies within a session. Extensive experiments conducted on two benchmark E-commerce datasets, Yoochoose and Diginetica demonstrate our proposed method outperforms the state-of-the-art methods consistently.
更多查看译文
关键词
repeated behavior pattern,recommendation,long-term,session-based
AI 理解论文
溯源树
样例
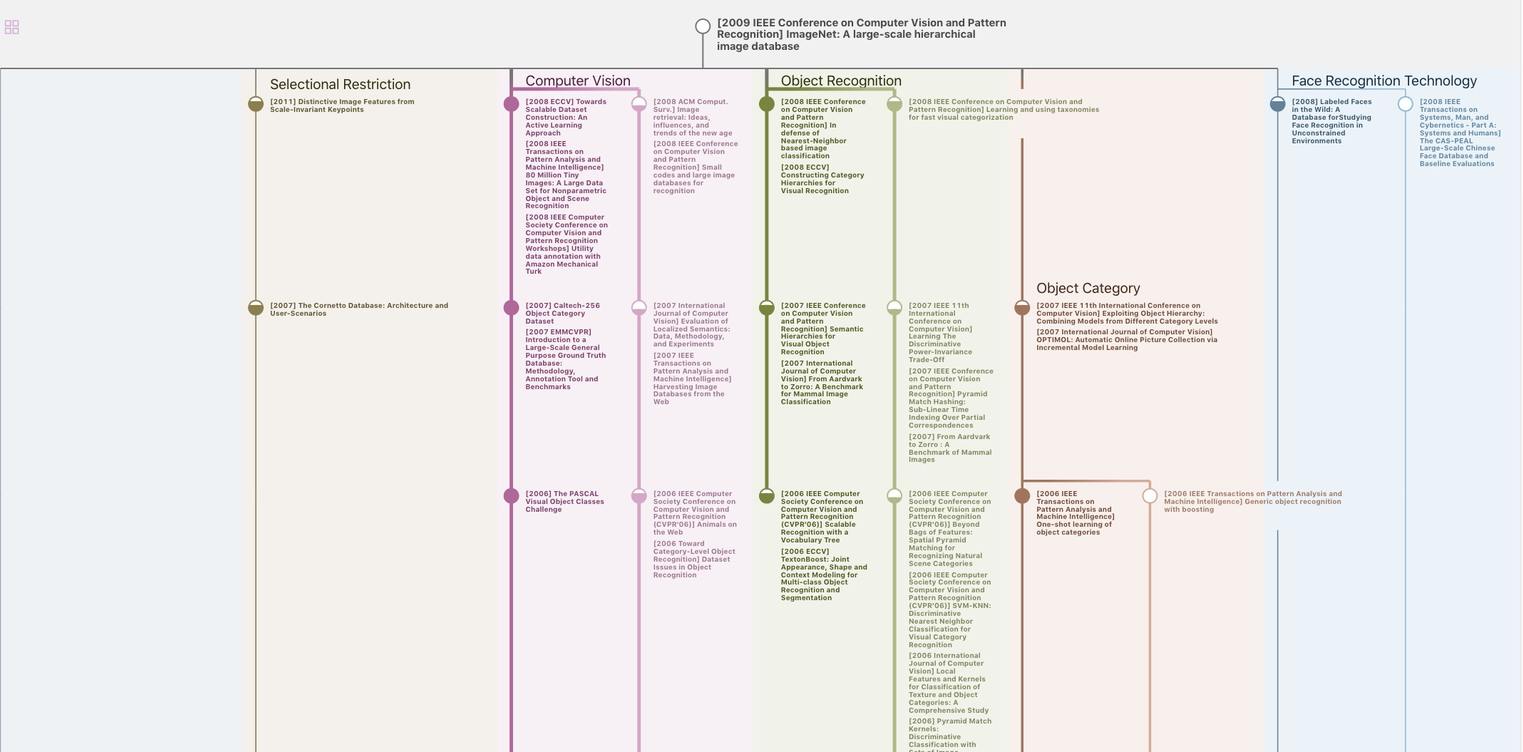
生成溯源树,研究论文发展脉络
Chat Paper
正在生成论文摘要