Research Challenges in Designing Differentially Private Text Generation Mechanisms.
FLAIRS Conference(2021)
摘要
Accurately learning from user data while ensuring quantifiable privacy guarantees provides an opportunity to build better ML models while maintaining user trust. Recent literature has demonstrated the applicability of a generalized form of Differential Privacy to provide guarantees over text queries. Such mechanisms add privacy preserving noise to vectorial representations of text in high dimension and return a text based projection of the noisy vectors. However, these mechanisms are sub-optimal in their trade-off between privacy and utility.\r\nIn this proposal paper, we describe some challenges in balancing this trade-off. At a high level, we provide two proposals: (1) a framework called LAC which defers some of the noise to a privacy amplification step and (2), an additional suite of three different techniques for calibrating the noise based on the local region around a word. Our objective in this paper is not to evaluate a single solution but to further the conversation on these challenges and chart pathways for building better mechanisms.
更多查看译文
关键词
text,generation
AI 理解论文
溯源树
样例
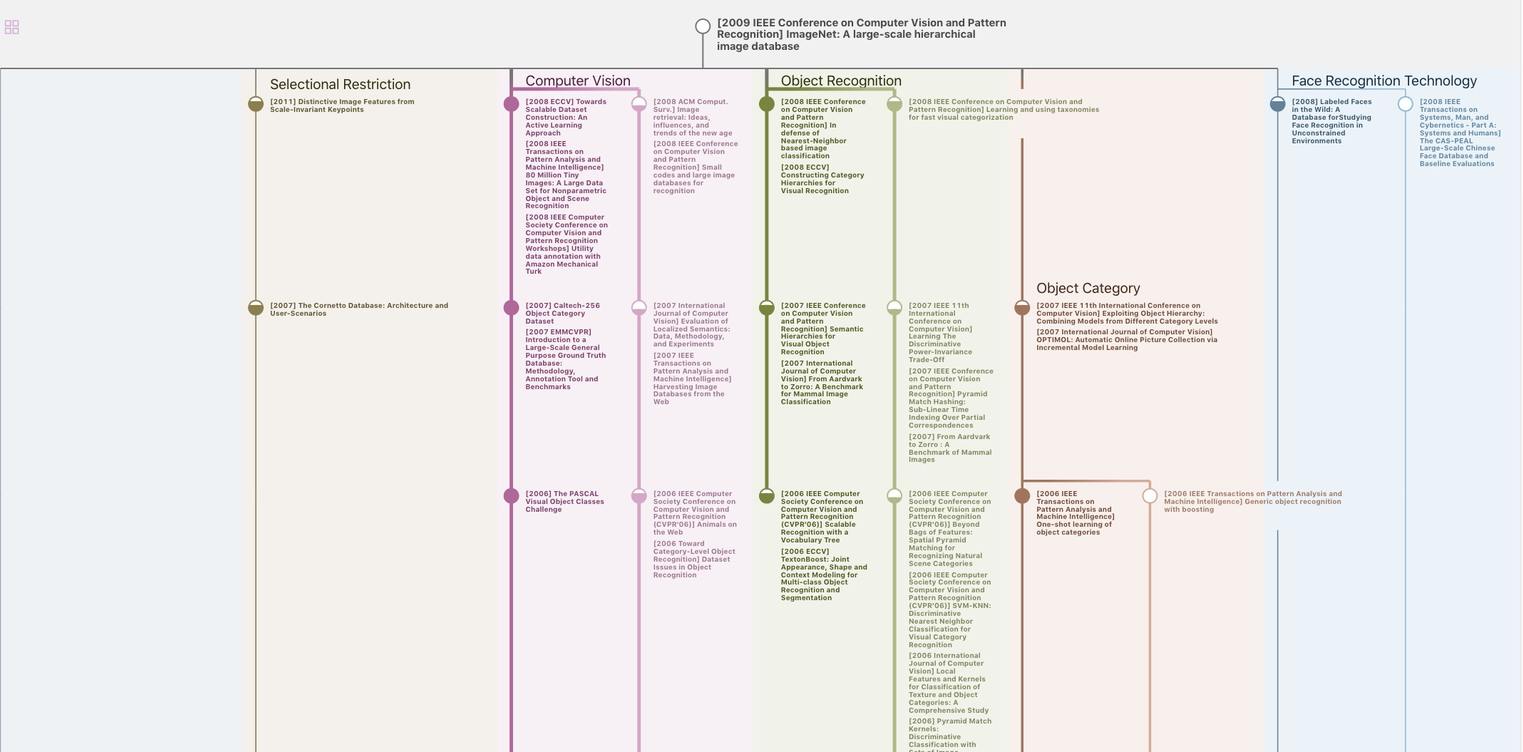
生成溯源树,研究论文发展脉络
Chat Paper
正在生成论文摘要