ViP-DeepLab: Learning Visual Perception with Depth-aware Video Panoptic Segmentation
2021 IEEE/CVF CONFERENCE ON COMPUTER VISION AND PATTERN RECOGNITION, CVPR 2021(2021)
摘要
In this paper, we present ViP-DeepLab, a unified model attempting to tackle the long-standing and challenging inverse projection problem in vision, which we model as restoring the point clouds from perspective image sequences while providing each point with instance-level semantic interpretations. Solving this problem requires the vision models to predict the spatial location, semantic class, and temporally consistent instance label for each 3D point. ViP-DeepLab approaches it by jointly performing monocular depth estimation and video panoptic segmentation. We name this joint task as Depth-aware Video Panoptic Segmentation, and propose a new evaluation metric along with two derived datasets for it, which will be made available to the public. On the individual sub-tasks, ViP-DeepLab also achieves state-of-the-art results, outperforming previous methods by 5.1% VPQ on Cityscapes-VPS, ranking 1st on the KITTI monocular depth estimation benchmark, and 1st on KITTI MOTS pedestrian. The datasets and the evaluation codes are made publicly available
1
.
更多查看译文
关键词
segmentation,visual perception,vip-deeplab,depth-aware
AI 理解论文
溯源树
样例
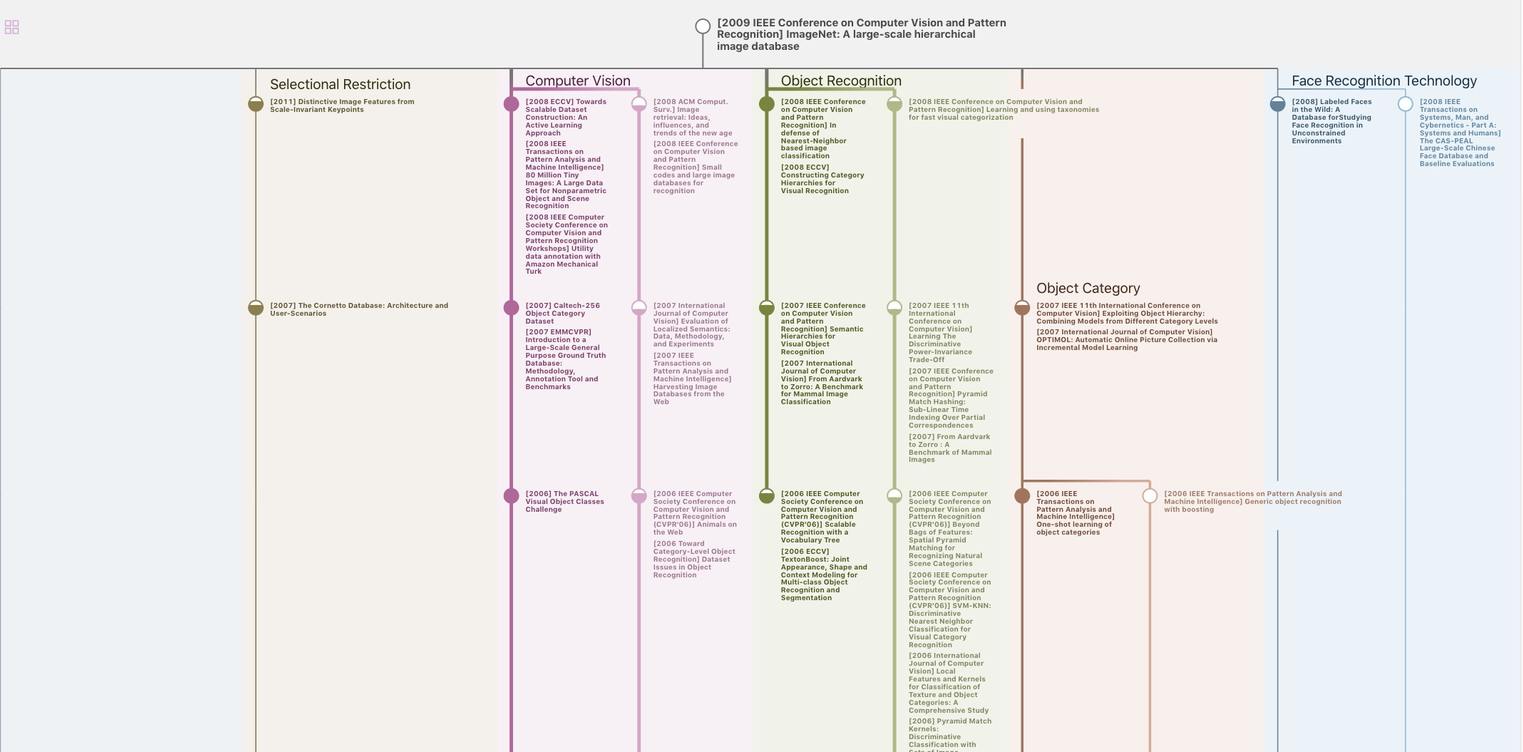
生成溯源树,研究论文发展脉络
Chat Paper
正在生成论文摘要