2-Step Sparse-View CT Reconstruction with a Domain-Specific Perceptual Network
arxiv(2020)
摘要
Computed tomography is widely used to examine internal structures in a non-destructive manner. To obtain high-quality reconstructions, one typically has to acquire a densely sampled trajectory to avoid angular undersampling. However, many scenarios require a sparse-view measurement leading to streak-artifacts if unaccounted for. Current methods do not make full use of the domain-specific information, and hence fail to provide reliable reconstructions for highly undersampled data. We present a novel framework for sparse-view tomography by decoupling the reconstruction into two steps: First, we overcome its ill-posedness using a super-resolution network, SIN, trained on the sparse projections. The intermediate result allows for a closed-form tomographic reconstruction with preserved details and highly reduced streak-artifacts. Second, a refinement network, PRN, trained on the reconstructions reduces any remaining artifacts. We further propose a light-weight variant of the perceptual-loss that enhances domain-specific information, boosting restoration accuracy. Our experiments demonstrate an improvement over current solutions by 4 dB.
更多查看译文
关键词
ct,sparse-view,domain-specific
AI 理解论文
溯源树
样例
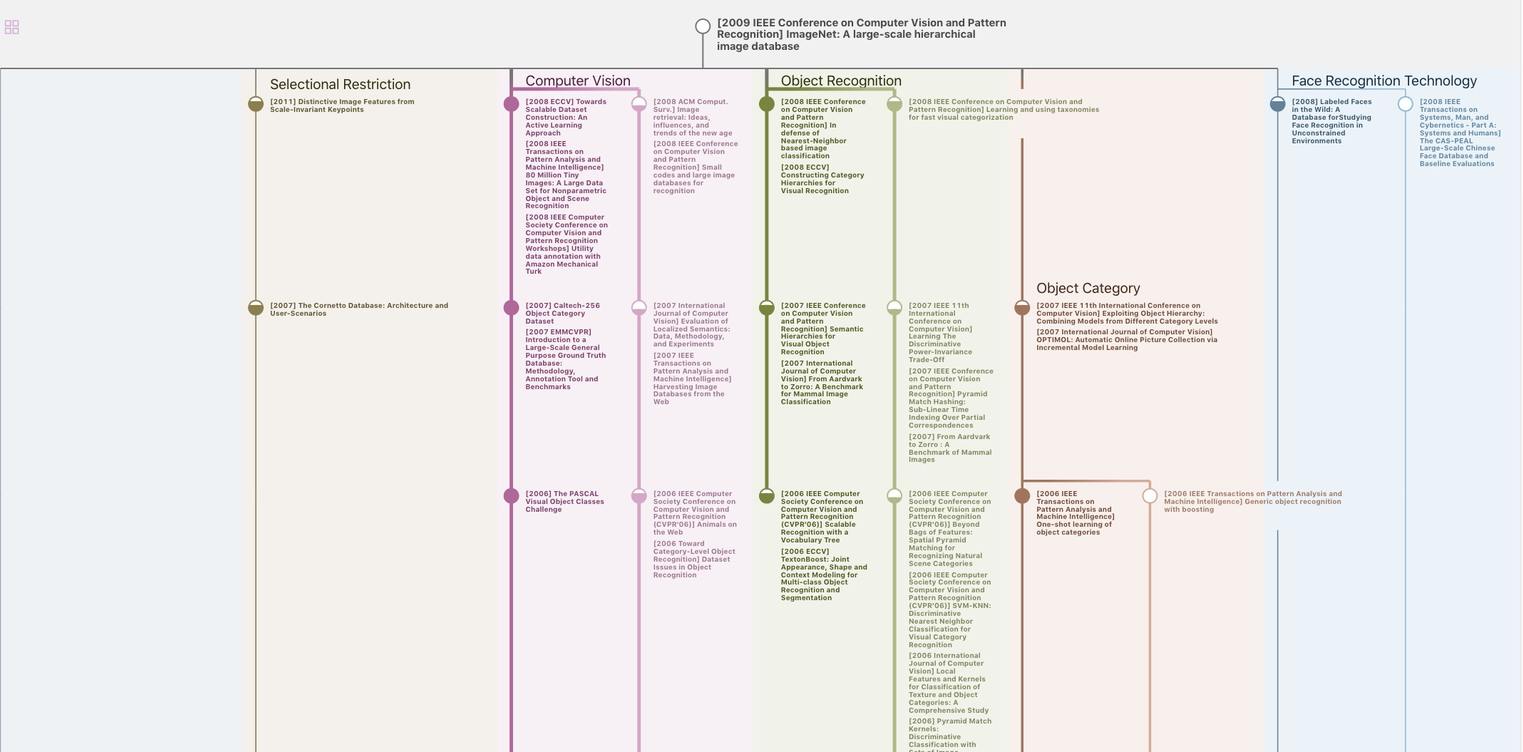
生成溯源树,研究论文发展脉络
Chat Paper
正在生成论文摘要